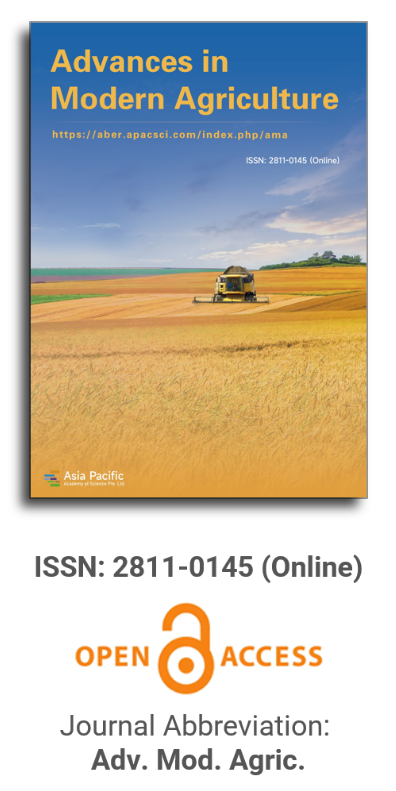
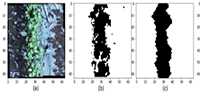
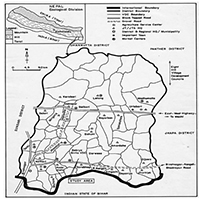
Recent advances of three-dimensional reconstruction technology and its application in modern agriculture
Vol 5, Issue 4, 2024
VIEWS - 1895 (Abstract)
Download PDF
Abstract
The timely acquisition of agricultural information is fundamental to smart agriculture, providing a basis for decision-making in agricultural production and ensuring protection against risks. With advancements in computer vision and machine learning, 3D reconstruction, the process of generating detailed digital models, has demonstrated substantial potential for mining and recording crucial information from objects, including geometry, structural attributes, visual appearance and other properties. This paper summarizes the applications of 3D reconstruction and measurement in the field of agricultural information acquisition based on prior research. It first reviews the 3D reconstruction and its related techniques and algorithms, then conducts a comprehensive analysis of the applications of 3D reconstruction and measurement in crop cultivation, animal husbandry, aquaculture and post-harvest products. It can be concluded that compared to traditional two-dimensional imagery, 3D reconstruction and measurement offer richer and more comprehensive information for agricultural practices, showing better performance in tasks such as organ segmentation, geometry measurement, health monitoring and simulation analysis. Future works can be launched from keeping up with the latest reconstruction technology, accelerating the 3D reconstruction, fusing multi-sensor data and combining 3D reconstruction with other information acquisition technologies.
Keywords
References
1. Poutanen KS, Kårlund AO, Gómez-Gallego C, et al. Grains—A major source of sustainable protein for health. Nutrition Reviews. 2022; 80(6): 1648-1663. doi: 10.1093/nutrit/nuab084
2. Godfray HCJ, Aveyard P, Garnett T, et al. Meat consumption, health, and the environment. Science. 2018; 361(6399). doi: 10.1126/science.aam5324
3. Huang J, Yang G. Understanding recent challenges and new food policy in China. Global Food Security. 2017; 12: 119-126. doi: 10.1016/j.gfs.2016.10.002
4. Foley JA, Ramankutty N, Brauman KA, et al. Solutions for a cultivated planet. Nature. 2011; 478(7369): 337-342. doi: 10.1038/nature10452
5. Tilman D, Balzer C, Hill J, et al. Global food demand and the sustainable intensification of agriculture. Proceedings of the National Academy of Sciences. 2011; 108(50): 20260-20264. doi: 10.1073/pnas.1116437108
6. FAO. The State of Food and Agriculture 2023. In FAO eBooks; 2023.
7. Elijah O, Rahman TA, Orikumhi I, et al. An Overview of Internet of Things (IoT) and Data Analytics in Agriculture: Benefits and Challenges. IEEE Internet of Things Journal. 2018; 5(5): 3758-3773. doi: 10.1109/jiot.2018.2844296
8. Saiz-Rubio V, Rovira-Más F. From Smart Farming towards Agriculture 5.0: A Review on Crop Data Management. Agronomy. 2020; 10(2): 207. doi: 10.3390/agronomy10020207
9. Nie J, Wang Y, Li Y, et al. Artificial intelligence and digital twins in sustainable agriculture and forestry: a survey. Turkish Journal of Agriculture and Forestry. 2022; 46(5): 642-661. doi: 10.55730/1300-011x.3033
10. Karunathilake EMBM, Le AT, Heo S, et al. The Path to Smart Farming: Innovations and Opportunities in Precision Agriculture. Agriculture. 2023; 13(8): 1593. doi: 10.3390/agriculture13081593
11. Fanzo J, Davis C, McLaren R, et al. The effect of climate change across food systems: Implications for nutrition outcomes. Global Food Security. 2018; 18: 12-19. doi: 10.1016/j.gfs.2018.06.001
12. Wang T, Chen B, Zhang Z, et al. Applications of machine vision in agricultural robot navigation: A review. Computers and Electronics in Agriculture. 2022; 198: 107085. doi: 10.1016/j.compag.2022.107085
13. Rehman TU, Mahmud MdS, Chang YK, et al. Current and future applications of statistical machine learning algorithms for agricultural machine vision systems. Computers and Electronics in Agriculture. 2019; 156: 585-605. doi: 10.1016/j.compag.2018.12.006
14. Yu F, Wang M, Xiao J, et al. Advancements in Utilizing Image-Analysis Technology for Crop-Yield Estimation. Remote Sensing. 2024; 16(6): 1003. doi: 10.3390/rs16061003
15. Niu H, Landivar J, Duffield N. Classification of cotton water stress using convolutional neural networks and UAV-based RGB imagery. Advances in Modern Agriculture. 2024; 5(1). doi: 10.54517/ama.v5i1.2457
16. Xiao L, Ding K, Gao Y, et al. Behavior-induced health condition monitoring of caged chickens using binocular vision. Computers and Electronics in Agriculture. 2019; 156: 254-262. doi: 10.1016/j.compag.2018.11.022
17. Jin X, Zarco-Tejada PJ, Schmidhalter U, et al. High-Throughput Estimation of Crop Traits: A Review of Ground and Aerial Phenotyping Platforms. IEEE Geoscience and Remote Sensing Magazine. 2021; 9(1): 200-231. doi: 10.1109/mgrs.2020.2998816
18. Verdouw C, Tekinerdogan B, Beulens A, et al. Digital twins in smart farming. Agricultural Systems. 2021; 189: 103046. doi: 10.1016/j.agsy.2020.103046
19. Pylianidis C, Osinga S, Athanasiadis IN. Introducing digital twins to agriculture. Computers and Electronics in Agriculture. 2021; 184: 105942. doi: 10.1016/j.compag.2020.105942
20. Lei L, Yang Q, Yang L, et al. Deep learning implementation of image segmentation in agricultural applications: a comprehensive review. Artificial Intelligence Review. 2024; 57(6). doi: 10.1007/s10462-024-10775-6
21. Zheng Y, Jiang W. Evaluation of Vision Transformers for Traffic Sign Classification. Wireless Communications and Mobile Computing. 2022; 2022: 1-14. doi: 10.1155/2022/3041117
22. Yang B, Wang X, Xing Y, et al. Modality Fusion Vision Transformer for Hyperspectral and LiDAR Data Collaborative Classification. IEEE Journal of Selected Topics in Applied Earth Observations and Remote Sensing. 2024; 17: 17052-17065. doi: 10.1109/jstars.2024.3415729
23. Tummala S, Kadry S, Bukhari SAC, et al. Classification of Brain Tumor from Magnetic Resonance Imaging Using Vision Transformers Ensembling. Current Oncology. 2022; 29(10): 7498-7511. doi: 10.3390/curroncol29100590
24. He M, Jiang W, Gu W. TriChronoNet: Advancing electricity price prediction with Multi-module fusion. Applied Energy. 2024; 371: 123626. doi: 10.1016/j.apenergy.2024.123626
25. Lu Y, Wang W, Bai R, et al. Hyper-relational interaction modeling in multi-modal trajectory prediction for intelligent connected vehicles in smart cites. Information Fusion. 2025; 114: 102682. doi: 10.1016/j.inffus.2024.102682
26. Han XF, Laga H, Bennamoun M. Image-Based 3D Object Reconstruction: State-of-the-Art and Trends in the Deep Learning Era. IEEE Transactions on Pattern Analysis and Machine Intelligence. 2021; 43(5): 1578-1604. doi: 10.1109/tpami.2019.2954885
27. Akhtar MS, Zafar Z, Nawaz R, et al. Unlocking plant secrets: A systematic review of 3D imaging in plant phenotyping techniques. Computers and Electronics in Agriculture. 2024; 222: 109033. doi: 10.1016/j.compag.2024.109033
28. Wang J, Xie Z, Mao P, et al. Fruit modeling and application based on 3D imaging technology: a review. Journal of Food Measurement and Characterization. 2024; 18(6): 4120-4136. doi: 10.1007/s11694-024-02480-3
29. Ma W, Qi X, Sun Y, et al. Computer Vision-Based Measurement Techniques for Livestock Body Dimension and Weight: A Review. Agriculture. 2024; 14(2): 306. doi: 10.3390/agriculture14020306
30. Lee Y. Three-Dimensional Dense Reconstruction: A Review of Algorithms and Datasets. Sensors. 2024; 24(18): 5861. doi: 10.3390/s24185861
31. Sutherland IE. Sketchpad—a man-machine graphical communication system. Seminal graphics. 1998.
32. Edl M, Mizerák M, Trojan J. 3D Laser Scanners: History and Applications. Acta Simulatio. 2018; 4(4): 1-5. doi: 10.22306/asim.v4i4.54
33. Van As H, van Duynhoven J. MRI of plants and foods. Journal of Magnetic Resonance. 2013; 229: 25-34. doi: 10.1016/j.jmr.2012.12.019
34. Ghosh T, Maity PP, Rabbi SMF, et al. Application of X-ray computed tomography in soil and plant -a review. Frontiers in Environmental Science. 2023; 11. doi: 10.3389/fenvs.2023.1216630
35. Mildenhall B, Srinivasan PP, Tancik M, et al. NeRF. Communications of the ACM. 2021; 65(1): 99-106. doi: 10.1145/3503250
36. Müller T, Evans A, Schied C, et al. Instant neural graphics primitives with a multiresolution hash encoding. ACM Transactions on Graphics. 2022; 41(4): 1-15. doi: 10.1145/3528223.3530127
37. Kerbl B, Kopanas G, Leimkuehler T, et al. 3D Gaussian Splatting for Real-Time Radiance Field Rendering. ACM Transactions on Graphics. 2023; 42(4): 1-14. doi: 10.1145/3592433
38. de Villiers HAC, Otten G, Chauhan A, et al. Autoencoder-based 3D representation learning for industrial seedling abnormality detection. Computers and Electronics in Agriculture. 2023; 206: 107619. doi: 10.1016/j.compag.2023.107619
39. Shi Z, Meng Z, Xing Y, Ma Y, Wattenhofer R. 3D-RETR: End-to-end single and multi-view 3d reconstruction with transformers. Computer Vision and Pattern Recognition; 2021.
40. Paproki A, Sirault X, Berry S, et al. A novel mesh processing based technique for 3D plant analysis. BMC Plant Biology. 2012; 12(1): 63. doi: 10.1186/1471-2229-12-63
41. Heinemann M, Herzfeld J, Sliwinski M, et al. A metrological and application-related comparison of six consumer grade stereo depth cameras for the use in robotics. In: Proceedings of the 2022 IEEE International Symposium on Robotic and Sensors Environments (ROSE); 2022.
42. Xu X, Zhang L, Yang J, et al. A Review of Multi-Sensor Fusion SLAM Systems Based on 3D LIDAR. Remote Sensing. 2022; 14(12): 2835. doi: 10.3390/rs14122835
43. Lowe DG. Distinctive Image Features from Scale-Invariant Keypoints. International Journal of Computer Vision. 2004.
44. Bay H, Ess A, Tuytelaars T, et al. Speeded-Up Robust Features (SURF). Computer Vision and Image Understanding. 2008; 110(3): 346-359. doi: 10.1016/j.cviu.2007.09.014
45. Fischler MA, Bolles RC. Random sample consensus. Communications of the ACM. 1981; 24(6): 381-395. doi: 10.1145/358669.358692
46. Maćkiewicz A, Ratajczak W. Principal components analysis (PCA). Computers & Geosciences; 1993.
47. Rusinkiewicz S, Levoy M. Efficient variants of the ICP algorithm. In: Proceedings of the Third International Conference on 3-D Digital Imaging and Modeling; 2001.
48. Ester M, Kriegel HP, Sander J, Xu X. A density-based algorithm for discovering clusters in large spatial databases with noise. In: Proceedings of the Second International Conference on Knowledge Discovery and Data Mining; 1996.
49. Qi CR, Yi L, Su H, Guibas LJ. PointNet++: deep hierarchical feature learning on point sets in a metric space. In: Proceedings of the 31st International Conference on Neural Information Processing Systems; 2017.
50. Hanocka R, Hertz A, Fish N, et al. MeshCNN. ACM Transactions on Graphics. 2019; 38(4): 1-12. doi: 10.1145/3306346.3322959
51. Liu Z, Tang H, Lin Y, Han S. Point-voxel CNN for efficient 3D deep learning. In: Proceedings of the 33rd International Conference on Neural Information Processing Systems; 2019.
52. Wu X, Jiang L, Wang PS, et al. Point Transformer V3: Simpler, Faster, Stronger. In: Proceedings of the 2024 IEEE/CVF Conference on Computer Vision and Pattern Recognition (CVPR); 2024.
53. Cao J, Tagliasacchi A, Olson M, et al. Point Cloud Skeletons via Laplacian Based Contraction. In: Proceedings of the 2010 Shape Modeling International Conference; 2010.
54. Huang H, Wu S, Cohen-Or D, et al. L 1 -medial skeleton of point cloud. ACM Transactions on Graphics. 2013; 32(4): 1-8. doi: 10.1145/2461912.2461913
55. Guo Y, Wang H, Hu Q, et al. Deep Learning for 3D Point Clouds: A Survey. IEEE Transactions on Pattern Analysis and Machine Intelligence. 2021; 43(12): 4338-4364. doi: 10.1109/tpami.2020.3005434
56. Hornik K, Stinchcombe M, White H. Universal approximation of an unknown mapping and its derivatives using multilayer feedforward networks. Neural Networks; 1990.
57. Park JJ, Florence P, Straub J, et al. DeepSDF: Learning Continuous Signed Distance Functions for Shape Representation. In: Proceedings of the 2019 IEEE/CVF Conference on Computer Vision and Pattern Recognition (CVPR); 2019.
58. Wang P, Liu L, Liu Y, et al. NeuS: Learning Neural Implicit Surfaces by Volume Rendering for Multi-view Reconstruction. In Advances in Neural Information Processing Systems; 2021.
59. Xiao S, Fei S, Li Q, et al. The Importance of Using Realistic 3D Canopy Models to Calculate Light Interception in the Field. Plant Phenomics. 2023; 5. doi: 10.34133/plantphenomics.0082
60. Chaudhury A, Godin C. Skeletonization of Plant Point Cloud Data Using Stochastic Optimization Framework. Frontiers in Plant Science. 2020; 11. doi: 10.3389/fpls.2020.00773
61. Li W, Wu S, Wen W, et al. Using high-throughput phenotype platform MVS-Pheno to reconstruct the 3D morphological structure of wheat. AoB PLANTS. 2024; 16(2). doi: 10.1093/aobpla/plae019
62. Fernandez R, Le Cunff L, Mérigeaud S, et al. End-to-end multimodal 3D imaging and machine learning workflow for non-destructive phenotyping of grapevine trunk internal structure. Scientific Reports. 2024; 14(1). doi: 10.1038/s41598-024-55186-3
63. Zhu W, Sun Z, Peng J, et al. Estimating Maize Above-Ground Biomass Using 3D Point Clouds of Multi-Source Unmanned Aerial Vehicle Data at Multi-Spatial Scales. Remote Sensing. 2019; 11(22): 2678. doi: 10.3390/rs11222678
64. Liu H, Xin C, Lai M, et al. RepC-MVSNet: A Reparameterized Self-Supervised 3D Reconstruction Algorithm for Wheat 3D Reconstruction. Agronomy. 2023; 13(8): 1975. doi: 10.3390/agronomy13081975
65. Wu S, Wen W, Xiao B, et al. An Accurate Skeleton Extraction Approach From 3D Point Clouds of Maize Plants. Frontiers in Plant Science. 2019; 10. doi: 10.3389/fpls.2019.00248
66. Arshad MA, Jubery T, Afful J, et al. Evaluating Neural Radiance Fields for 3D Plant Geometry Reconstruction in Field Conditions. Plant Phenomics. 2024; 6. doi: 10.34133/plantphenomics.0235
67. Mccormick RF, Truong SK, Mullet JE. 3D sorghum reconstructions from depth images identify QTL regulating shoot architecture. Plant Physiology; 2016.
68. Gong L, Du X, Zhu K, et al. Panicle-3D: Efficient Phenotyping Tool for Precise Semantic Segmentation of Rice Panicle Point Cloud. Plant Phenomics. 2021; 2021. doi: 10.34133/2021/9838929
69. Wu D, Yu L, Ye J, et al. Panicle-3D: A low-cost 3D-modeling method for rice panicles based on deep learning, shape from silhouette, and supervoxel clustering. The Crop Journal. 2022; 10(5): 1386-1398. doi: 10.1016/j.cj.2022.02.007
70. Chang A, Jung J, Yeom J, et al. 3D Characterization of Sorghum Panicles Using a 3D Point Cloud Derived from UAV Imagery. Remote Sensing. 2021; 13(2): 282. doi: 10.3390/rs13020282
71. Li Y, Wen W, Miao T, et al. Automatic organ-level point cloud segmentation of maize shoots by integrating high-throughput data acquisition and deep learning. Computers and Electronics in Agriculture. 2022; 193: 106702. doi: 10.1016/j.compag.2022.106702
72. Su Y, Wu F, Ao Z, et al. Evaluating maize phenotype dynamics under drought stress using terrestrial lidar. Plant Methods. 2019; 15(1). doi: 10.1186/s13007-019-0396-x
73. Qiu R, Miao Y, Zhang M, et al. Detection of the 3D temperature characteristics of maize under water stress using thermal and RGB-D cameras. Computers and Electronics in Agriculture. 2021; 191: 106551. doi: 10.1016/j.compag.2021.106551
74. Gilliot JM, Michelin J, Hadjard D, et al. An accurate method for predicting spatial variability of maize yield from UAV-based plant height estimation: a tool for monitoring agronomic field experiments. Precision Agriculture. 2020; 22(3): 897-921. doi: 10.1007/s11119-020-09764-w
75. Okamoto T, Kimura A, Shimono H, et al. 3D reconstruction of rice plant community using spectral images with a goal of making rice breeding efficient. In: Proceedings of the International Workshop on Advanced Imaging Technology (IWAIT) 2023; 2023.
76. Gu Y, Wang Y, Wu Y, et al. Novel 3D photosynthetic traits derived from the fusion of UAV LiDAR point cloud and multispectral imagery in wheat. Remote Sensing of Environment. 2024; 311: 114244. doi: 10.1016/j.rse.2024.114244
77. Sun Z, Li Q, Jin S, et al. Simultaneous Prediction of Wheat Yield and Grain Protein Content Using Multitask Deep Learning from Time-Series Proximal Sensing. Plant Phenomics. 2022; 2022. doi: 10.34133/2022/9757948
78. Kurdyś-Kujawska A, Strzelecka A, Zawadzka D. The Impact of Crop Diversification on the Economic Efficiency of Small Farms in Poland. Agriculture. 2021; 11(3): 250. doi: 10.3390/agriculture11030250
79. Marks E, Magistri F, Stachniss C. Precise 3D Reconstruction of Plants from UAV Imagery Combining Bundle Adjustment and Template Matching. In: Proceedings of the 2022 International Conference on Robotics and Automation (ICRA); 2022.
80. Zhang J, Wang X, Ni X, et al. Neural radiance fields for multi-scale constraint-free 3D reconstruction and rendering in orchard scenes. Computers and Electronics in Agriculture. 2024; 217: 108629. doi: 10.1016/j.compag.2024.108629
81. Smitt C, Halstead M, Zimmer P, et al. PAg-NeRF: Towards Fast and Efficient End-to-End Panoptic 3D Representations for Agricultural Robotics. IEEE Robotics and Automation Letters. 2024; 9(1): 907-914. doi: 10.1109/lra.2023.3338515
82. Kelly S, Riccardi A, Marks E, et al. Target-Aware Implicit Mapping for Agricultural Crop Inspection. In: Proceedings of the 2023 IEEE International Conference on Robotics and Automation (ICRA); 2023.
83. Xie P, Ma Z, Du R, et al. An unmanned ground vehicle phenotyping-based method to generate three-dimensional multispectral point clouds for deciphering spatial heterogeneity in plant traits. Molecular Plant. 2024; 17(10): 1624-1638. doi: 10.1016/j.molp.2024.09.004
84. Xu Y, Hu C, Xie Y. An improved space colonization algorithm with DBSCAN clustering for a single tree skeleton extraction. International Journal of Remote Sensing. 2022; 43(10): 3692-3713. doi: 10.1080/01431161.2022.2102950
85. Kim CH, Kantor G. Occlusion Reasoning for Skeleton Extraction of Self-Occluded Tree Canopies. In: Proceedings of the 2023 IEEE International Conference on Robotics and Automation (ICRA); 2023.
86. Chebrolu N, Labe T, Stachniss C. Spatio-Temporal Non-Rigid Registration of 3D Point Clouds of Plants. In: Proceedings of the 2020 IEEE International Conference on Robotics and Automation (ICRA); 2020.
87. Sun S, Li C, Chee PW, et al. High resolution 3D terrestrial LiDAR for cotton plant main stalk and node detection. Computers and Electronics in Agriculture. 2021; 187: 106276. doi: 10.1016/j.compag.2021.106276
88. Ma B, Du J, Wang L, et al. Automatic branch detection of jujube trees based on 3D reconstruction for dormant pruning using the deep learning-based method. Computers and Electronics in Agriculture. 2021; 190: 106484. doi: 10.1016/j.compag.2021.106484
89. Du R, Ma Z, Xie P, et al. PST: Plant segmentation transformer for 3D point clouds of rapeseed plants at the podding stage. ISPRS Journal of Photogrammetry and Remote Sensing. 2023; 195: 380-392. doi: 10.1016/j.isprsjprs.2022.11.022
90. Tsoulias N, Paraforos DS, Xanthopoulos G, et al. Apple Shape Detection Based on Geometric and Radiometric Features Using a LiDAR Laser Scanner. Remote Sensing. 2020; 12(15): 2481. doi: 10.3390/rs12152481
91. Yuan Q, Wang P, Luo W, et al. Simultaneous Localization and Mapping System for Agricultural Yield Estimation Based on Improved VINS-RGBD: A Case Study of a Strawberry Field. Agriculture. 2024; 14(5): 784. doi: 10.3390/agriculture14050784
92. Xiao S, Fei S, Ye Y, et al. 3D reconstruction and characterization of cotton bolls in situ based on UAV technology. ISPRS Journal of Photogrammetry and Remote Sensing. 2024; 209: 101-116. doi: 10.1016/j.isprsjprs.2024.01.027
93. Saeed F, Sun J, Ozias-Akins P, et al. PeanutNeRF: 3D Radiance Field for Peanuts. In: Proceedings of the 2023 IEEE/CVF Conference on Computer Vision and Pattern Recognition Workshops (CVPRW); 2023.
94. Moualeu-Ngangué D, Bötzl M, Stützel H. First Form, Then Function: 3D Reconstruction of Cucumber Plants (Cucumis sativus L.) Allows Early Detection of Stress Effects through Leaf Dimensions. Remote Sensing. 2022; 14(5): 1094. doi: 10.3390/rs14051094
95. Hao H, Wu S, Li Y, et al. Automatic acquisition, analysis and wilting measurement of cotton 3D phenotype based on point cloud. Biosystems Engineering. 2024; 239: 173-189. doi: 10.1016/j.biosystemseng.2024.02.010
96. Feng L, Chen S, Wu B, et al. Detection of oilseed rape clubroot based on low-field nuclear magnetic resonance imaging. Computers and Electronics in Agriculture. 2024; 218: 108687. doi: 10.1016/j.compag.2024.108687
97. Wang Y, Mücher S, Wang W, et al. A review of three-dimensional computer vision used in precision livestock farming for cattle growth management. Computers and Electronics in Agriculture. 2023; 206: 107687. doi: 10.1016/j.compag.2023.107687
98. Zhou H, Li Q, Xie Q. Individual Pig Identification Using Back Surface Point Clouds in 3D Vision. Sensors. 2023; 23(11): 5156. doi: 10.3390/s23115156
99. Lei K, Tang X, Li X, et al. Research and Preliminary Evaluation of Key Technologies for 3D Reconstruction of Pig Bodies Based on 3D Point Clouds. Agriculture. 2024; 14(6): 793. doi: 10.3390/agriculture14060793
100. Hao H, Jincheng Y, Ling Y, et al. An improved PointNet++ point cloud segmentation model applied to automatic measurement method of pig body size. Computers and Electronics in Agriculture. 2023; 205: 107560. doi: 10.1016/j.compag.2022.107560
101. Le Cozler Y, Allain C, Caillot A, et al. High-precision scanning system for complete 3D cow body shape imaging and analysis of morphological traits. Computers and Electronics in Agriculture. 2019; 157: 447-453. doi: 10.1016/j.compag.2019.01.019
102. Pezzuolo A, Guarino M, Sartori L, et al. A Feasibility Study on the Use of a Structured Light Depth-Camera for Three-Dimensional Body Measurements of Dairy Cows in Free-Stall Barns. Sensors. 2018; 18(2): 673. doi: 10.3390/s18020673
103. Tun SC, Onizuka T, Tin P, et al. Revolutionizing Cow Welfare Monitoring: A Novel Top-View Perspective with Depth Camera-Based Lameness Classification. Journal of Imaging. 2024; 10(3): 67. doi: 10.3390/jimaging10030067
104. Zhang X, Zhang Y, Geng J, et al. Feather Damage Monitoring System Using RGB-Depth-Thermal Model for Chickens. Animals. 2022; 13(1): 126. doi: 10.3390/ani13010126
105. Veinidis C, Arnaoutoglou F, Syvridis D. 3D Reconstruction of Fishes Using Coded Structured Light. Journal of Imaging. 2023; 9(9): 189. doi: 10.3390/jimaging9090189
106. Feng G, Pan B, Chen M. Non-Contact Tilapia Mass Estimation Method Based on Underwater Binocular Vision. Applied Sciences. 2024; 14(10): 4009. doi: 10.3390/app14104009
107. Audira G, Sampurna B, Juniardi S, et al. A Simple Setup to Perform 3D Locomotion Tracking in Zebrafish by Using a Single Camera. Inventions. 2018; 3(1): 11. doi: 10.3390/inventions3010011
108. Yin J, Zhu D, Shi M, et al. MoFiM: A morphable fish modeling method for underwater binocular vision system. Computer Animation and Virtual Worlds. 2022; 33(5). doi: 10.1002/cav.2104
109. Wu R, Deussen O, Li L. DeepShapeKit: accurate 4D shape reconstruction of swimming fish. In: Proceedings of the 2022 IEEE/RSJ International Conference on Intelligent Robots and Systems (IROS); 2022.
110. Muñoz-Benavent P, Andreu-García G, Valiente-González JM, et al. Enhanced fish bending model for automatic tuna sizing using computer vision. Computers and Electronics in Agriculture. 2018; 150: 52-61. doi: 10.1016/j.compag.2018.04.005
111. Deng Y, Tan H, Zhou D, et al. An automatic body length estimating method for Micropterus salmoides using local water surface stereo vision. Biosystems Engineering. 2023; 235: 166-179. doi: 10.1016/j.biosystemseng.2023.09.013
112. Huang K, Li Y, Suo F, et al. Stereo Vison and Mask-RCNN Segmentation Based 3D Points Cloud Matching for Fish Dimension Measurement. In: Proceedings of the 2020 39th Chinese Control Conference (CCC); 2020.
113. Gao T, Xiong Z, Li Z, et al. Precise underwater fish measurement: A geometric approach leveraging medium regression. Computers and Electronics in Agriculture. 2024; 221: 108932. doi: 10.1016/j.compag.2024.108932
114. Shi C, Zhao R, Liu C, et al. Underwater fish mass estimation using pattern matching based on binocular system. Aquacultural Engineering. 2022; 99: 102285. doi: 10.1016/j.aquaeng.2022.102285
115. Nygård TA, Jahren JH, Schellewald C, et al. Motion trajectory estimation of salmon using stereo vision. IFAC-PapersOnLine. 2022; 55(31): 363-368. doi: 10.1016/j.ifacol.2022.10.455
116. Aya S, Stian J, Morten B, Mats M, Eleni K. StereoYolo + DeepSORT: A framework to track fish from underwater stereo camera in situ. In Proc.SPIE; 2024.
117. Atienza-Vanacloig V, Andreu-García G, López-García F, et al. Vision-based discrimination of tuna individuals in grow-out cages through a fish bending model. Computers and Electronics in Agriculture. 2016; 130: 142-150. doi: 10.1016/j.compag.2016.10.009
118. Pérez-Escudero A, Vicente-Page J, Hinz RC, et al. idTracker: tracking individuals in a group by automatic identification of unmarked animals. Nature Methods. 2014; 11(7): 743-748. doi: 10.1038/nmeth.2994
119. Wang Y, Chen Y. Fruit Morphological Measurement Based on Three-Dimensional Reconstruction. Agronomy. 2020; 10(4): 455. doi: 10.3390/agronomy10040455
120. Zheng HY, Li Y, Wang N, et al. A novel framework for three-dimensional electrical impedance tomography reconstruction of maize ear via feature reconfiguration and residual networks. PeerJ Computer Science. 2024; 10: e1944. doi: 10.7717/peerj-cs.1944
121. Li G, Li H, Li X, et al. Establishment and Calibration of Discrete Element Model for Buckwheat Seed Based on Static and Dynamic Verification Test. Agriculture. 2023; 13(5): 1024. doi: 10.3390/agriculture13051024
122. Xie W, Wei S, Yang D. Morphological measurement for carrot based on three-dimensional reconstruction with a ToF sensor. Postharvest Biology and Technology. 2023; 197: 112216. doi: 10.1016/j.postharvbio.2022.112216
123. Ma H, Zhu X, et al. Rapid estimation of apple phenotypic parameters based on 3D reconstruction. International Journal of Agricultural and Biological Engineering. 2021; 14(5): 180-188. doi: 10.25165/j.ijabe.20211405.6258
124. Yu S, Yan X, Jia T, et al. Binocular structured light-based 3D reconstruction for morphological measurements of apples. Postharvest Biology and Technology. 2024; 213: 112952. doi: 10.1016/j.postharvbio.2024.112952
125. Gao Y, Wang Q, Rao X, et al. OrangeStereo: A navel orange stereo matching network for 3D surface reconstruction. Computers and Electronics in Agriculture. 2024; 217: 108626. doi: 10.1016/j.compag.2024.108626
126. Huang T, Bian Y, Niu Z, et al. Fast neural distance field-based three-dimensional reconstruction method for geometrical parameter extraction of walnut shell from multiview images. Computers and Electronics in Agriculture. 2024; 224: 109189. doi: 10.1016/j.compag.2024.109189
127. Mollazade K, Lucht J van der, Jörissen S, et al. 3D laser imaging for measuring volumetric shrinkage of horticultural products during drying process. Computers and Electronics in Agriculture. 2023; 207: 107749. doi: 10.1016/j.compag.2023.107749
128. Ni X, Li C, Jiang H, et al. Three-dimensional photogrammetry with deep learning instance segmentation to extract berry fruit harvestability traits. ISPRS Journal of Photogrammetry and Remote Sensing. 2021; 171: 297-309. doi: 10.1016/j.isprsjprs.2020.11.010
129. Bernard A, Hamdy S, Le Corre L, et al. 3D characterization of walnut morphological traits using X-ray computed tomography. Plant Methods. 2020; 16(1). doi: 10.1186/s13007-020-00657-7
130. Zhang Y, Hui Y, Zhou Y, et al. Characterization and Detection Classification of Moldy Corn Kernels Based on X-CT and Deep Learning. Applied Sciences. 2024; 14(5): 2166. doi: 10.3390/app14052166
131. Zhao H, Wang J, Liao S, et al. Study on the micro-phenotype of different types of maize kernels based on Micro-CT. Smart Agriculture; 2021.
132. Yan H. 3D Scanner-Based Corn Seed Modeling. Applied Engineering in Agriculture; 2016.
133. Mi G, Liu Y, Wang T, et al. Measurement of Physical Properties of Sorghum Seeds and Calibration of Discrete Element Modeling Parameters. Agriculture. 2022; 12(5): 681. doi: 10.3390/agriculture12050681
134. Huang H, Tian G, Chen C. Evaluating the Point Cloud of Individual Trees Generated from Images Based on Neural Radiance Fields (NeRF) Method. Remote Sensing. 2024; 16(6): 967. doi: 10.3390/rs16060967
Refbacks
- There are currently no refbacks.
Copyright (c) 2024 Ting Huang, Tao Wang, Ziang Niu, Chen Yang, Zixing Wu, Zhengjun Qiu
License URL: https://creativecommons.org/licenses/by/4.0/
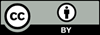
This site is licensed under a Creative Commons Attribution 4.0 International License (CC BY 4.0).
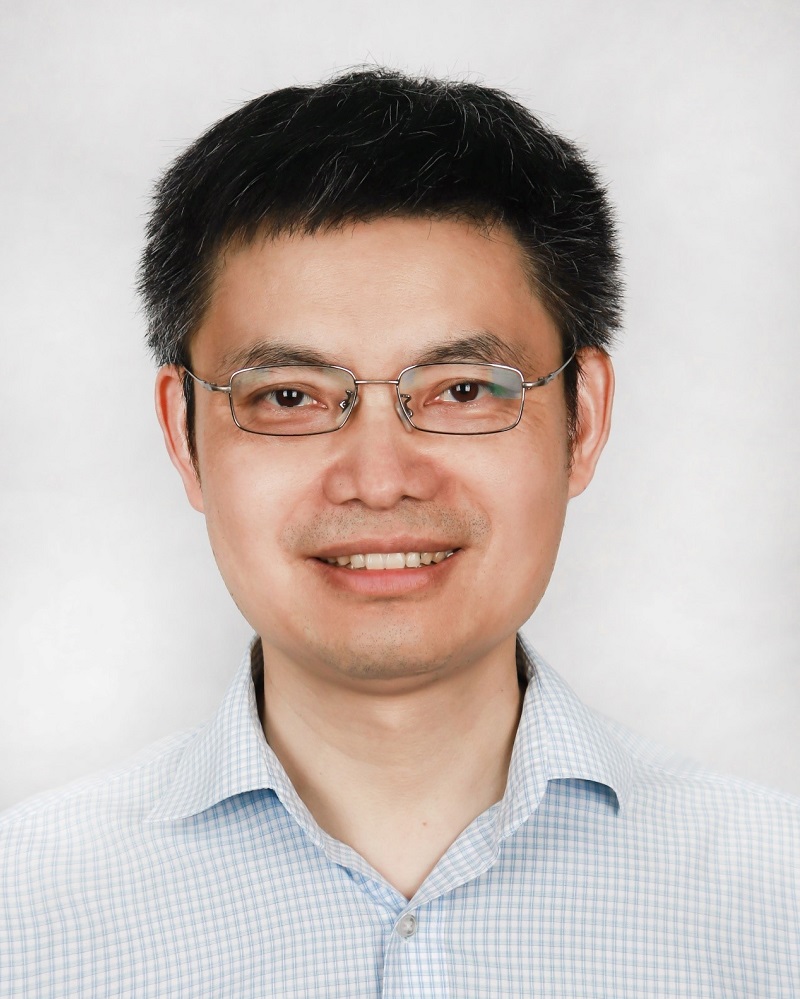
Prof. Zhengjun Qiu
Zhejiang University, China
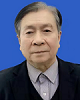
Cheng Sun
Academician of World Academy of Productivity Science; Executive Chairman, World Confederation of Productivity Science China Chapter, China
Indexing & Archiving
In the realm of modern agriculture, the integration of cutting-edge technologies is revolutionizing the way we approach sustainable farming practices. A recent study published in Advances in Modern Agriculture titled "Classification of cotton water stress using convolutional neural networks and UAV-based RGB imagery" has garnered significant attention for its innovative approach to precision irrigation management. Conducted by researchers from Institute of Data Science and the AgriLife Research and Extension Center of Texas A&M University (authors's information is below). This study introduces a novel method for classifying cotton water stress using unmanned aerial vehicles (UAVs) and convolutional neural networks (CNNs), offering a powerful solution for optimizing water use in agriculture.
Modern agricultural technology is evolving rapidly, with scientists collaborating with leading agricultural enterprises to develop intelligent management practices. These practices utilize advanced systems that provide tailored fertilization and treatment options for large-scale land management.
This journal values human initiative and intelligence, and the employment of AI technologies to write papers that replace the human mind is expressly prohibited. When there is a suspicious submission that uses AI tools to quickly piece together and generate research results, the editorial board of the journal will reject the article, and all journals under the publisher's umbrella will prohibit all authors from submitting their articles.
Readers and authors are asked to exercise caution and strictly adhere to the journal's policy regarding the usage of Artificial Intelligence Generated Content (AIGC) tools.
Asia Pacific Academy of Science Pte. Ltd. (APACSCI) specializes in international journal publishing. APACSCI adopts the open access publishing model and provides an important communication bridge for academic groups whose interest fields include engineering, technology, medicine, computer, mathematics, agriculture and forestry, and environment.