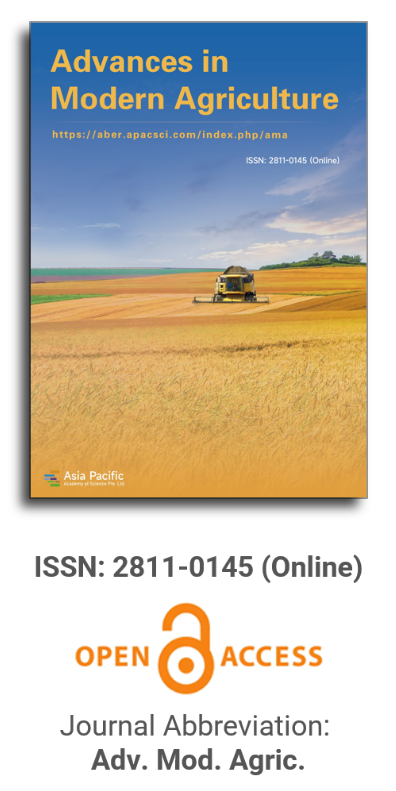
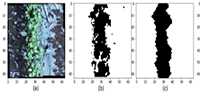
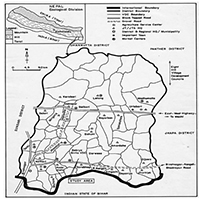
Modern agricultural technology is evolving rapidly, with scientists collaborating with leading agricultural enterprises to develop intelligent management practices. These practices utilize advanced systems that provide tailored fertilization and treatment options for large-scale land management. For instance, at a farm operated by the Beidahuang Group in Heilongjiang province, China, managers employ smart systems that integrate drones and high-precision multispectral imaging. This technology generates detailed maps indicating plant health, where red areas signify poor growth and blue areas indicate healthy growth. The data collected from these sensors allows farmers to monitor rice plant growth, manage pest and disease outbreaks, and optimize nutrient application, enhancing overall agricultural efficiency and convenience through real-time messaging networks[1]. Moreover, research conducted by Moch Rafli Kusoiry et al.[2] has employed the linear spectral unmixing (LSU) technique to classify different rice varieties based on pixel analysis from multispectral images. Their validation tests, which included a confusion matrix and Kappa analysis, achieved an overall accuracy rate of 85.48% and a Kappa score of 70.6%, demonstrating the effectiveness of this approach in agricultural monitoring. The advancements in agricultural intelligence are progressively replacing traditional manual labor, moving from theoretical concepts to practical applications. As a result, achieving precise and refined agricultural management is increasingly becoming a reality rather than a distant goal.
References:
|
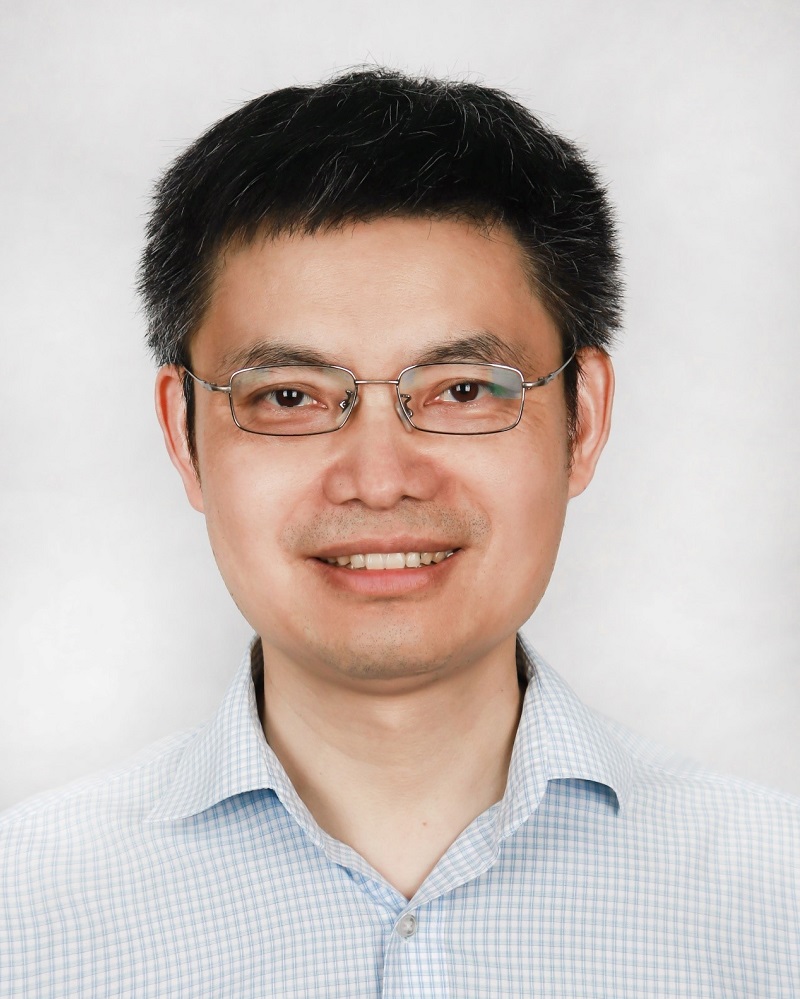
Prof. Zhengjun Qiu
Zhejiang University, China
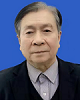
Cheng Sun
Academician of World Academy of Productivity Science; Executive Chairman, World Confederation of Productivity Science China Chapter, China
Indexing & Archiving
Modern agricultural technology is evolving rapidly, with scientists collaborating with leading agricultural enterprises to develop intelligent management practices. These practices utilize advanced systems that provide tailored fertilization and treatment options for large-scale land management.
This journal values human initiative and intelligence, and the employment of AI technologies to write papers that replace the human mind is expressly prohibited. When there is a suspicious submission that uses AI tools to quickly piece together and generate research results, the editorial board of the journal will reject the article, and all journals under the publisher's umbrella will prohibit all authors from submitting their articles.
Readers and authors are asked to exercise caution and strictly adhere to the journal's policy regarding the usage of Artificial Intelligence Generated Content (AIGC) tools.
Asia Pacific Academy of Science Pte. Ltd. (APACSCI) specializes in international journal publishing. APACSCI adopts the open access publishing model and provides an important communication bridge for academic groups whose interest fields include engineering, technology, medicine, computer, mathematics, agriculture and forestry, and environment.