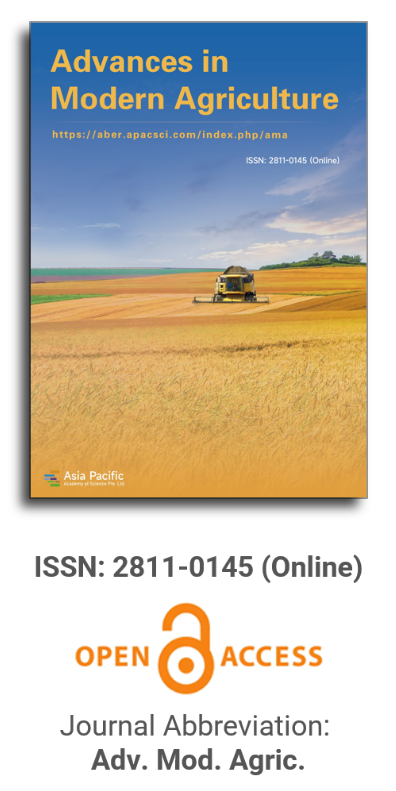
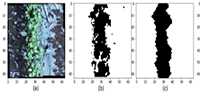
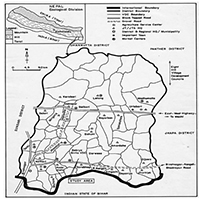
Issue release: 30 June 2025
This study investigates pesticide residues in cucumber plants and their impact on soil nematode populations while evaluating the effect of pesticides on cucumber growth and yield. Gas Chromatography Tandem Mass Spectrometry (GC-MS/MS) was used to quantify pesticide residues, comparing the results to the Maximum Residue Limits (MRLs) defined by the Codex Alimentarius. Significant differences in residue levels were found between various pesticides and application rates. Diazinon residues ranged from 0.86 to 2.28 mg/kg, exceeding the MRL of 0.1 mg/kg, indicating soil contamination. Endosulfan had the lowest residues, from 0.44 to 1.75 mg/kg, which were within acceptable limits. Conversely, Malathion and Methoxychlor residues notably surpassed their MRLs, raising potential safety concerns. Further analysis using a linear regression model revealed a negative correlation between pesticide application and soil nematode populations. There was a proportional decrease in nematode populations with increasing pesticide application rate, with Malathion having the most significant impact, followed by Endosulfan, Methoxychlor, and Diazinon. The impact of pesticide application on cucumber plant growth and yield was assessed using one-way ANOVA, which uncovered significant differences across treatment groups. While pesticides are effective for pest control, their application must be carefully managed to avoid phytotoxicity and ensure optimal plant and environmental health, thereby enhancing maximum productivity.
Issue release: 30 June 2025
This study examined the impacts of land use on the physical and chemical properties of soils of land use types along agroforestry and agricultural landscapes in a rainforest zone of Nigeria. The land use systems are forest, agroforestry, fallow, and ornamental plant fields in addition to permanent crop fields (cocoa, oil palm, and citrus) and annual crop fields (maize). Profile pits were dug on the land use types and samples were collected 0–20 cm and 20–50 cm for laboratory analysis. Soil samples were collected from undisturbed soil and profile pits for bulk density and moisture content determination following standard analytical procedures. Among the land use types, physical properties (sand, clay, soil bulk density) and chemical properties (soil pH, SOC, total N, P, K, Ca, Mg, and CEC) differed significantly. Bulk density, pH, SOC, total, and stocks of SOC and N differed statistically for 0–20 and 20–50 cm soil depths with downward increases in N and SOC stocks along sampling depth. Permanent croplands (forest and agroforestry fields) had higher soil pH, SOC, total N, and CEC, while arable crop fields had relatively lower pH, SOC, TN, P, K, Ca, Mg, and CEC. Arable fields had significantly lower C and N stocks within 50 cm compared with permanent crop fields, which may be attributed to continuous tillage by the smallholder farmers and soil erosion-enhanced SOC and N removal from top soil. For both permanent and annual crop fields, SOC and total N stocks ranged from 5.75 to 3.12 kg/m2 for 0–20 cm depths and 2.44 to 1.93 kg/m2 for deeper (20–50 cm) layers. Relative to forest soil, stocks of SOC in the surface soils (0–20 cm) decreased in the order: agroforestry > ornamental plant field > cocoa > fallow land > citrus > oil palm > annual cropping system. Following this decreasing order, soil deterioration indices are equivalent to 27% > 28% > 30% > 31% > 32% > 34% > 38% compared with forest soil, respectively. Strong significant correlations (p < 0.05) were observed between SOC and TN stocks and some soil properties (bulk density, clay contents, pH, and CEC) with R2 values ranging from 1.0 to 0.85. It is concluded that the soil's physical and chemical properties and carbon storage potential differed among the land uses of the study site.
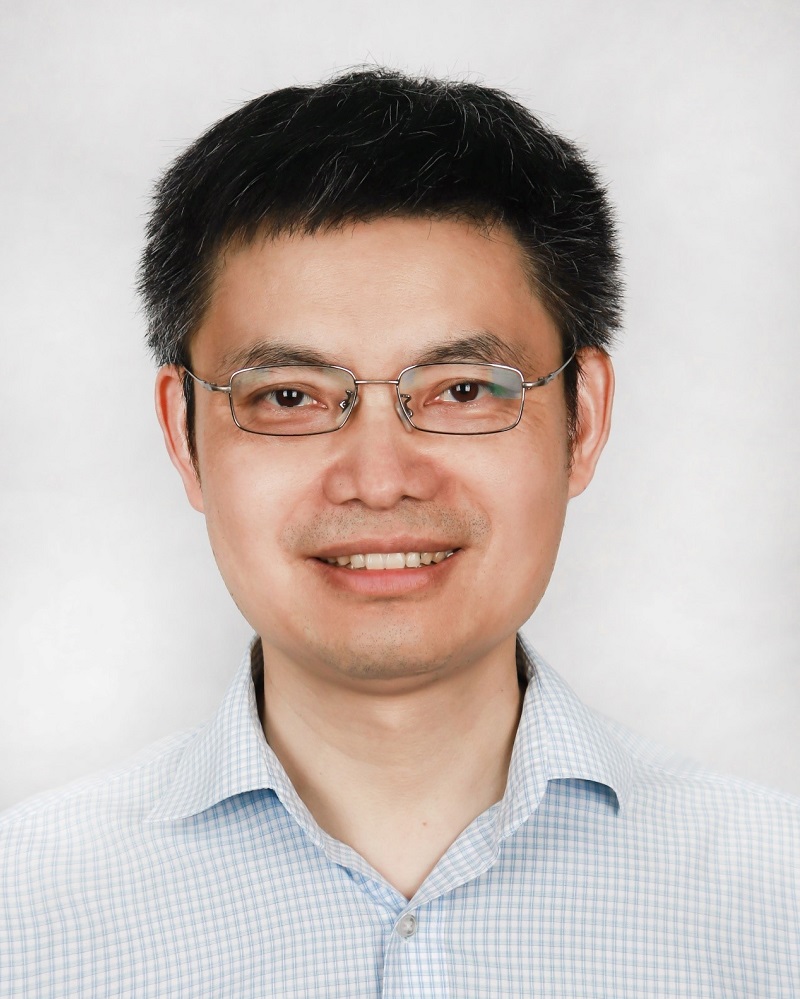
Prof. Zhengjun Qiu
Zhejiang University, China
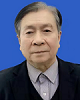
Cheng Sun
Academician of World Academy of Productivity Science; Executive Chairman, World Confederation of Productivity Science China Chapter, China
Indexing & Archiving
-
-
-
-
-
-
- J-Gate
-
-
-
In the realm of modern agriculture, the integration of cutting-edge technologies is revolutionizing the way we approach sustainable farming practices. A recent study published in Advances in Modern Agriculture titled "Classification of cotton water stress using convolutional neural networks and UAV-based RGB imagery" has garnered significant attention for its innovative approach to precision irrigation management. Conducted by researchers from Institute of Data Science and the AgriLife Research and Extension Center of Texas A&M University (authors's information is below). This study introduces a novel method for classifying cotton water stress using unmanned aerial vehicles (UAVs) and convolutional neural networks (CNNs), offering a powerful solution for optimizing water use in agriculture.
Modern agricultural technology is evolving rapidly, with scientists collaborating with leading agricultural enterprises to develop intelligent management practices. These practices utilize advanced systems that provide tailored fertilization and treatment options for large-scale land management.
This journal values human initiative and intelligence, and the employment of AI technologies to write papers that replace the human mind is expressly prohibited. When there is a suspicious submission that uses AI tools to quickly piece together and generate research results, the editorial board of the journal will reject the article, and all journals under the publisher's umbrella will prohibit all authors from submitting their articles.
Readers and authors are asked to exercise caution and strictly adhere to the journal's policy regarding the usage of Artificial Intelligence Generated Content (AIGC) tools.
Asia Pacific Academy of Science Pte. Ltd. (APACSCI) specializes in international journal publishing. APACSCI adopts the open access publishing model and provides an important communication bridge for academic groups whose interest fields include engineering, technology, medicine, computer, mathematics, agriculture and forestry, and environment.