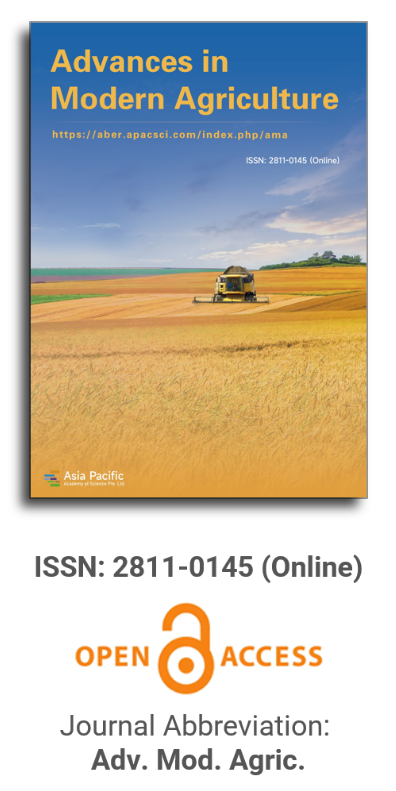
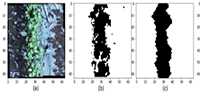
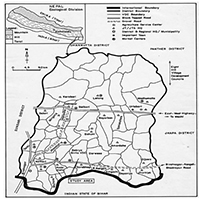
Using satellite image data to identify rice varieties through linear spectral unmixing method (case study: Karangjati Sub District, Ngawi Regency)
Vol 5, Issue 2, 2024
VIEWS - 2849 (Abstract)
Download PDF
Abstract
Remote sensing technology has increasingly emerged as a potent tool for precision agriculture, particularly in facilitating the mapping and monitoring of crops on a large scale. An application of this technology is the identification of different types of rice by analyzing the pixels acquired in satellite images. Regrettably, the pixels in the image have been mixed from different recorded items. Therefore, they have the potential to influence the outcome of the identification. An effective approach to addressing this problem is to employ the linear spectral unmixing (LSU) technique. The LSU approach quantifies the ratio of pure objects in every pixel of an image by utilizing the spectral value associated with the endmember of the rice variety. The investigation was carried out in the Karangjati District during the generative stage (70 ± DAP) of the rice planting season. The data indicates that the dominant variety is Inpari 32 HDB. The data validation tests, which involved the use of a confusion matrix and Kappa analysis, resulted in an overall accuracy rate of 85.48% and a Kappa analysis score of 70.6%.
Keywords
References
1. Azhar HM, Susilastuti D. Analisis Keragaman Hayati Tanaman Padi (Oryza sativa, L). AGRISIA - J. Ilmu-Ilmu Pertan. 2017; 9(2).
2. Ningsih F. Identification of morphological and agronomic characters of some local paddy rice cultivars from the north kampar sub-district of kampar district in the vegetative phase (Indonesian). Universitas Islam Negeri Sultan Syarif Kasim, Riau; 2018.
3. Supriyanti A, Supriyanta, Kristamtini. Characterization of Twenty Local Rice (Oryza Sativa L.) in Yogyakarta Special Region. Vegetalika. 2015; 4(3): 29-41.
4. Pratiwi SH. Growth and Yield of Rice (Oryza sativa L.) on various planting pattern and addition of organic fertilizers. Gontor AGROTECH Science Journal. 2016; 2(2). doi: 10.21111/agrotech.v2i2.410
5. Sari VD, Sukojo BM. Analysis of Rice Production Estimation Based on Growth Phase and Autoregressive Integrated Moving Average (Arima) Forecasting Model Using Landsat 8 Satellite Image (Case Study: Bojonegoro Regency) (Indonesian). Geoid. 2015; 10(2): 194. doi: 10.12962/j24423998.v10i2.828
6. Cipta IM, Jaelani LM, Sanjaya H. Identification of Paddy Varieties from Landsat 8 Satellite Image Data Using Spectral Unmixing Method in Indramayu Regency, Indonesia. ISPRS International Journal of Geo-Information. 2022; 11(10): 510. doi: 10.3390/ijgi11100510
7. Congalton RG. Remote Sensing and Image Interpretation. 7th Edition. Photogrammetric Engineering & Remote Sensing. 2015; 81(8): 615-616. doi: 10.14358/pers.81.8.615
8. Hidayat I, Wijayanto H, Afendi FM. Evaluation of Paddy Production Mesurement in Indonesia. IOP Conference Series: Earth and Environmental Science. 2018; 187: 012035. doi: 10.1088/1755-1315/187/1/012035
9. Liu M, Liu X, Wu L, et al. A Modified Spatiotemporal Fusion Algorithm Using Phenological Information for Predicting Reflectance of Paddy Rice in Southern China. Remote Sensing. 2018; 10(5): 772. doi: 10.3390/rs10050772
10. Sabins FF. Remote Sensing: Principles and Interpretation, 3rd ed. Waveland Press; 2007.
11. Zhao R, Li Y, Chen J, et al. Mapping a Paddy Rice Area in a Cloudy and Rainy Region Using Spatiotemporal Data Fusion and a Phenology-Based Algorithm. Remote Sensing. 2021; 13(21): 4400. doi: 10.3390/rs13214400
12. Parsons AJ. Principles of Remote Sensing. By P. J. Curran. (London: Longman, 1985) [Pp. 260.] Price £1195. International Journal of Remote Sensing. 1985; 6(11): 1765-1765. doi: 10.1080/01431168508948322
13. Wei J, Wang X. An Overview on Linear Unmixing of Hyperspectral Data. Mathematical Problems in Engineering. 2020; 2020: 1-12. doi: 10.1155/2020/3735403
14. Yin Z, Yang B. Unsupervised Nonlinear Hyperspectral Unmixing with Reduced Spectral Variability via Superpixel-Based Fisher Transformation. Remote Sensing. 2023; 15(20): 5028. doi: 10.3390/rs15205028
15. Gandharum Mubekti L, Sanjaya H, Sadmono H, Wibowo BS. Penerapan Metode Spectral Linear Unmixing Pada Citra Landsat TM dan Data Spektrometer Untuk Memetakan Tanaman Padi Terserang Penyakit Hawar Daun Bakteri. In: Pertemuan Ilmiah Tahunan XX dan Kongres MAPIN; 2015.
16. Rivani AP, Jaelani LM, Sumargana L. Identifikasi Varietas Jagung dari Data Citra Satelit Menggunakan Metode Linier Spectral Unmixing (Studi Kasus: Kabupaten Ngawi). Geoid. 2023; 19(1): 106. doi: 10.12962/j24423998.v19i1.18749
17. Sanjaya H, Sukotjo BM, Jaelani LM, et al. Utilizing a Spectroradiometer to Build a Spectral-Library of Rice Leaves. 2022 IEEE Asia-Pacific Conference on Geoscience, Electronics and Remote Sensing Technology (AGERS). Published online December 21, 2022. doi: 10.1109/agers56232.2022.10093263
18. Gorelick N, Hancher M, Dixon M, et al. Google Earth Engine: Planetary-scale geospatial analysis for everyone. Remote Sensing of Environment. 2017; 202: 18-27. doi: 10.1016/j.rse.2017.06.031
19. Rouse JW, Haas RH, Schell JA, Deeering D. Monitoring vegetation systems in the Great Plains with ERTS (Earth Resources Technology Satellite). In: Third Earth Resources Technology Satellite-1 Symposium; 1973.
20. Rouse JW Jr, Haas R, Schell J, Deering D. Monitoring vegetation systems in the Great Plains with ERTS. NASA Spec. Publ; 1974.
21. Xu H. Modification of normalised difference water index (NDWI) to enhance open water features in remotely sensed imagery. International Journal of Remote Sensing. 2006; 27(14): 3025-3033. doi: 10.1080/01431160600589179
22. Keshava N. A Survey of Spectral Unmixing Algorithms. Lincoln Lab. J. 2003; 14(1): 55-78.
23. Keshava N, Mustard JF. Spectral unmixing. IEEE Signal Processing Magazine. 2002; 19(1): 44-57. doi: 10.1109/79.974727
24. Plaza A, Plaza J. Parallel implementation of linear and nonlinear spectral unmixing of remotely sensed hyperspectral images. High-Performance Computing in Remote Sensing. Published online October 6, 2011. doi: 10.1117/12.897326
25. Congalton RG. A review of assessing the accuracy of classifications of remotely sensed data. Remote Sens. Environ. 1991; 37(1): 35-46. doi: 10.1016/0034-4257(91)90048-B
26. Anderson JR, Hardy EE, Roach JT, et al. A land use and land cover classification system for use with remote sensor data. Professional Paper. Published online 1976. doi: 10.3133/pp964
27. Landis JR, Koch GG. The Measurement of Observer Agreement for Categorical Data. Biometrics. 1977; 33(1): 159. doi: 10.2307/2529310
28. Kosasih D, Buce Saleh M, Budi Prasetyo L. Visual and Digital Interpretations for Land Cover Classification in Kuningan District, West Java. Jurnal Ilmu Pertanian Indonesia. 2019; 24(2): 101-108. doi: 10.18343/jipi.24.2.101
Refbacks
- There are currently no refbacks.
Copyright (c) 2024 Moch Rafli Kusoiry, Lalu Muhamad Jaelani, Hartanto Sanjaya
License URL: https://creativecommons.org/licenses/by/4.0/
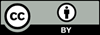
This site is licensed under a Creative Commons Attribution 4.0 International License (CC BY 4.0).
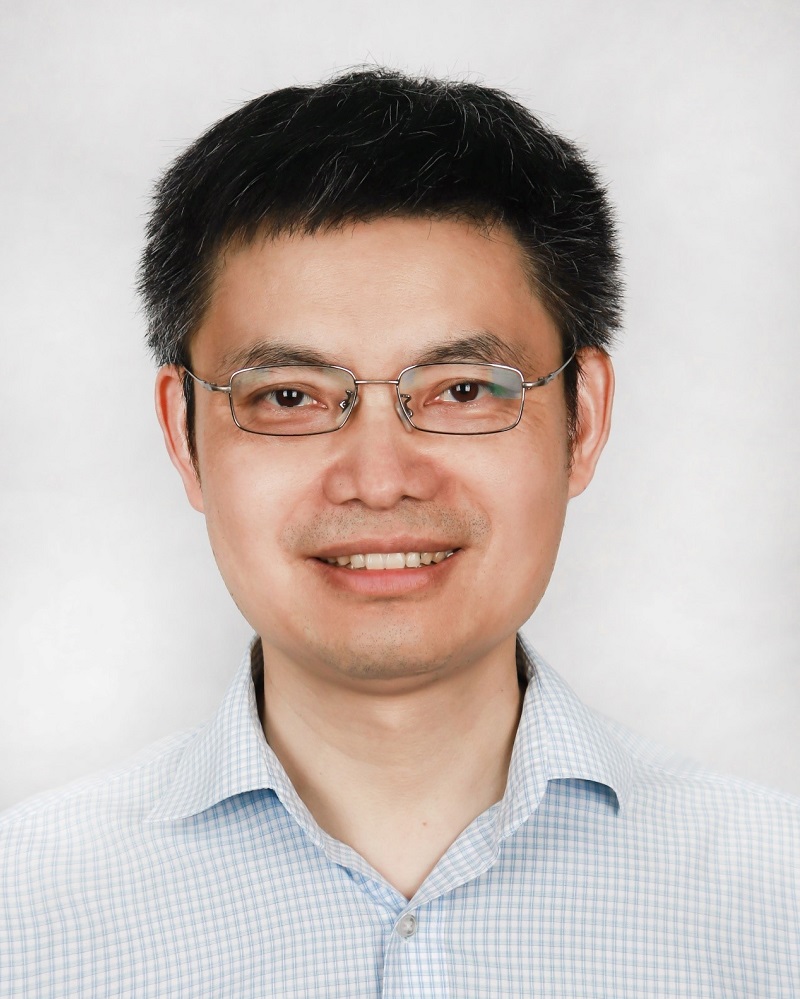
Prof. Zhengjun Qiu
Zhejiang University, China
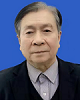
Cheng Sun
Academician of World Academy of Productivity Science; Executive Chairman, World Confederation of Productivity Science China Chapter, China
Indexing & Archiving
In the realm of modern agriculture, the integration of cutting-edge technologies is revolutionizing the way we approach sustainable farming practices. A recent study published in Advances in Modern Agriculture titled "Classification of cotton water stress using convolutional neural networks and UAV-based RGB imagery" has garnered significant attention for its innovative approach to precision irrigation management. Conducted by researchers from Institute of Data Science and the AgriLife Research and Extension Center of Texas A&M University (authors's information is below). This study introduces a novel method for classifying cotton water stress using unmanned aerial vehicles (UAVs) and convolutional neural networks (CNNs), offering a powerful solution for optimizing water use in agriculture.
Modern agricultural technology is evolving rapidly, with scientists collaborating with leading agricultural enterprises to develop intelligent management practices. These practices utilize advanced systems that provide tailored fertilization and treatment options for large-scale land management.
This journal values human initiative and intelligence, and the employment of AI technologies to write papers that replace the human mind is expressly prohibited. When there is a suspicious submission that uses AI tools to quickly piece together and generate research results, the editorial board of the journal will reject the article, and all journals under the publisher's umbrella will prohibit all authors from submitting their articles.
Readers and authors are asked to exercise caution and strictly adhere to the journal's policy regarding the usage of Artificial Intelligence Generated Content (AIGC) tools.
Asia Pacific Academy of Science Pte. Ltd. (APACSCI) specializes in international journal publishing. APACSCI adopts the open access publishing model and provides an important communication bridge for academic groups whose interest fields include engineering, technology, medicine, computer, mathematics, agriculture and forestry, and environment.