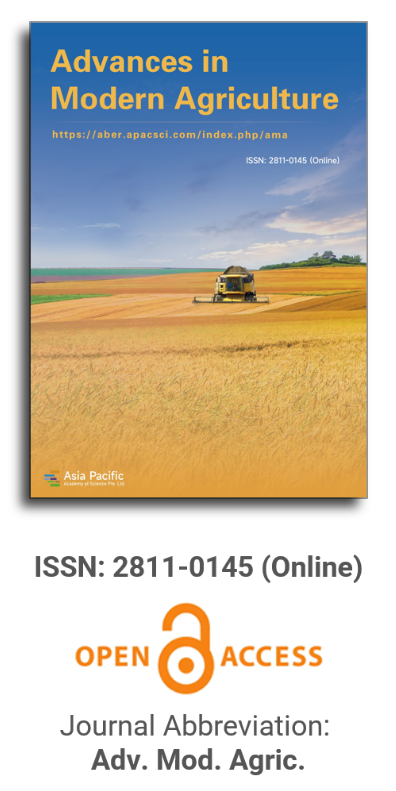
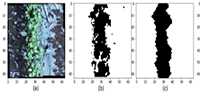
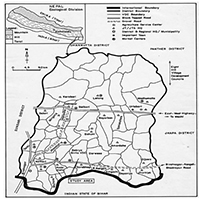
Application progress of machine vision technology in the field of modern agricultural equipment
Vol 2, Issue 1, 2021
VIEWS - 4836 (Abstract)
Download PDF
Abstract
With the rapid progress of image processing algorithms and computer equipment, the development of machine vision technology in the field of modern agricultural equipment is on the ascendant, and major application results have been obtained in many production links to improve the efficiency and automation of agricultural production. In the face of China, the world’s largest agricultural market, agricultural machine vision equipment undoubtedly has tremendous development potential and market prospects. This paper introduces the research and application of machine vision technology in agricultural equipment in the fields of agricultural product sorting, production automation, pest control, picking machinery, navigation, and positioning, analyzes and summarizes the current problems, and looks forward to the future development trend.
Keywords
References
1. Xiao L. “Agricultural Machinery Equipment Development Action Plan (2016–2025)” was released and implemented (Chinese). Agricultural Engineering 2017; 1: 54.
2. Chai M, Chen H, Li L. Development and outlook of precision farming in China. Journal of Chinese Agricultural Mechanization 2015; 36(5): 342–344, 348.
3. Focus on the Central Central Document No. 1 in 2017 (Chinese). New Agriculture 2017; 4: 7–9.
4. Zhang H, Liu Y. Research on the application of machine vision technology (Chinese). Electronics World 2013; 17: 106, 108.
5. Baigvand M, Banakar A, Minaei S, et al. Machine vision system for grading of dried figs. Computers and Electronics in Agriculture 2015; 119: 158–165. doi: 10.1016/j.compag.2015.10.019
6. Sture Ø, Øye ER, Skavhaug A, et al. A 3D machine vision system for quality grading of Atlantic salmon. Computers and Electronics in Agriculture 2016; 123: 142–148. doi: 10.1016/j.compag.2016.02.020
7. Omid O, Moghaddam PA, Motlagh AM. Online tomato sorting base on shape, maturity, size, and surface defects using machine vision. Turkish Journal Agriculture & Forestry 2014: 37(1): 62–68.
8. Wang S, Liu M, He J, et al. Study of rapid and automatic non0destructive testing grader for red jujubes. Transactions of Chinese Society for Agricultural Machinery 2014; 45(9): 79–83, 95.
9. Sofu MM, Er O, Kayacan MC, et al. Design of an automatic apple sorting system using machine vision. Computers and Electronics in Agriculture 2016; 127: 395–405. doi: 10.1016/j.compag.2016.06.030
10. Zhang J, Wu Z, Song P, et al. Embryo feature extraction and dynamic recognition method for maize haploid seeds. Transactions of Chinese Society for Agricultural Machinery 2013; 29(4): 199–203.
11. ShenY, Xu H, Liu H, et al. A new detection method with color and depth images based on Kinectsensor for greenhouse plants. Journal of Chinese Agricultural Mechanization 2016; 37(8): 155–161.
12. Shan J, Peng Y, Wang W, et al. Simultaneous detection of external and internal quality parameters of apples using hyperspectral technology. Transactions of Chinese Society for Agricultural Machinery 2011; 31(3): 771–775.
13. Zhang D, Liu R, Song X, et al. A field-based pushbroom imaging spectrometer for estimating chlorophyll content of maize. Spectroscopy and Spectral Analysis 2011; 31(3): 771–775.
14. Nasirahmadi A, Richter U, Hensel O, et al. Using machine vision for investigation of changes in pig group lying patterns. Computers and Electronics in Agriculture 2015; 119: 184–190. doi: 10.1016/j.compag.2015.10.023
15. Qureshi WS, Payne A, Walsh KB, et al. Machine vision for counting fruit on mango tree canopies. Precision Agriculture 2016; 17(3): 1–21.
16. Saberioon M, Gholizadeh A, Cisar P, et al. Application of machine vision systems in aquaculture with emphasis on fish: State-of-the-art and key issues. Reviews in Aquaculture 2016; 110(2): 466–469.
17. Zhang J, Ma Z, Li W, et al. Seeding accuracy test experiment based on soil substrate. Transactions of Chinese Society for Agricultural Machinery 2012; 43(12): 62–66, 71.
18. Ma Z, Hiroshi S, Gu S. Non-destructive measurement system for plant growth information based on machine vision. Transactions of Chinese Society for Agricultural Machinery 2010; 26(9): 203–209.
19. Jiang K, Zheng W, Zhang Q, et al. Development and experiment of vegetable grafting robot. Transactions of Chinese Society for Agricultural Machinery 2012; 28(4): 8–14.
20. Yang Y, Cao Q, Sheng G, et al. Plug tray localization and detection system based on machine vision. Transactions of Chinese Society for Agricultural Machinery 2013; 14(5): 232–235.
21. Gongal A, Silwal A, Amatya S, et al. Apple crop-load estimation with over-the-row machine vision system. Computers and Electronics in Agriculture 2016; 120: 26–35. doi: 10.1016/j.compag.2015.10.022
22. Shide AK, Shukla MY. Crop detection by machine vision for weed management. International Journal of Advances in Engineering & Technology 2014; 7(3).
23. Zhang J, Cao Z, Geng C, et al. Research on precision target spray robot in greenhouse. Transactions of Chinese Society for Agricultural Machinery 2009; 25(S2): 70–73.
24. Yuan X, Guo Z. Applied research on machine vison application in automatic detection of crop diseases. Journal of Huaiyin Institute of Technology 2017; 26(3): 6–11, 32.
25. Li Z, Hong T, Wang J, et al. Development and experiment of Panonychus citri infestation fast detector. Transactions of Chinese Society for Agricultural Machinery 2014; 30(14): 49–56.
26. Han R, He Y. Remote automatic identification system of field pests based on computer vision. Transactions of Chinese Society for Agricultural Machinery 2013; 29(3): 156–162.
27. Zhang H, Mao H, Han L. Detection of live Sitophilus oryzae (L.) in stored wheat by near-infrared hyperspectral imaging. Transactions of Chinese Society for Agricultural Machinery 2012; 28(8); 263–268.
28. Xiang R, Ying Y, Jiang H. Development of real-time recognition and localization methods for fruits and vegetables in field. Transactions of Chinese Society for Agricultural Machinery 2013; 44(11): 208–223.
29. Yuan T, Li W, Tan Y, et al. Information acquisition for cucumber harvesting robot in greenhouse. Transactions of Chinese Society for Agricultural Machinery 2009; 40(10): 151–155.
30. Si Y, Qiao J, Liu G, et al. Recognition and location of fruits for apple harvesting robot. Transactions of Chinese Society for Agricultural Machinery 2010; 41(9): 148–153.
31. Li S, Zhou J, Ji C, et al. Moving obstacle detection based on panoramic vision for intelligent agricultural vehicle. Transactions of Chinese Society for Agricultural Machinery 2013; 44(12): 239–244.
32. Xiong J, Zhou X, Chen L, et al. Visual position of picking manipulator for disturbed litchi. Transactions of Chinese Society for Agricultural Machinery 2012; 28(14): 36–41.
33. Feng J, Liu G, Si Y, et al. Construction of lase vision system for apple harvesting robot. Transactions of Chinese Society for Agricultural Machinery 2013; 29(S): 32–37.
34. Zhang R, Zhang X, Gao W, et al. The application of Beidou Navigation System in the field of agriculture (Chinese). Bulletin of Surveying and Mapping 2012; S1: 685–686, 694.
35. Davies ER. The application of machine vision to food and agriculture: A review. The Imaging Science Journal 2009; 57(4): 197–217. doi: 10.1179/174313109x454756
36. Wang P, Zhang J, Lan Y, et al. Radiometric calibration of low altitude multispectral remote sensing images. Transactions of Chinese Society for Agricultural Machinery 2014; 30(19): 199–206.
37. Ding Y, Wang S. Vision navigation control system for combine harvester. Transactions of Chinese Society for Agricultural Machinery 2010; 41(5): 137–142.
38. Guo W, Chen Y. Fuzzy control based autonomous navigation for a weeding robot. Robot 2010; 32(2): 204–209. doi: 10.3724/sp.j.1218.2010.00204
39. Chen Y, Zhang M, Ma W, et al. Positioning method of integrated navigation based on GPS and machine vision. Transactions of Chinese Society for Agricultural Machinery 2011; 27(3): 126–130.
40. Wang Z, Ma R. Rustic opinion on the existing problems and countermeasures to the development of precision agriculture in China. Journal of Chinese Agricultural Mechanization 2013; 34(5): 16–19, 34.
41. Wang Y, Jin B. Application of machine vision recognition technology in agricultural machinery. Mechanical Research & Application 2014; 6: 87–90.
42. Zhang J, Xun Y, Li W. Mangosteen grading based on computer vision. Transactions of Chinese Society for Agricultural Machinery 2009; 40(11): 176–179, 188.
43. Liu Y, Li Y. The application of machine vision technology in agricultural engineering (Chinese). Computer Knowledge and Technology 2017; 13(23): 149–150, 169.
44. Zhao N, Zhao P, Gao Y. Study on application of machine vision technology to modern agriculture in China. Journal of Tianjin Agricultural College 2015; 2: 55–58.
45. He Z, Wu F, Zhang H. General situation and development of precision agriculture in our country. Chinese Agricultural Mechanization 2009; 6: 23–26.
46. Hao G, Hou J, Guo C. Study on mode of socialized peasants choosing precision agriculture. Chinese Agricultural Mechanization 2011; 32(2): 24–27.
47. Wang F, Zheng J, Tang Y, et al. Analysis on application and research progress of machine vision in agriculture in China. Shandong Agricultural Sciences 2016; 48(4): 139–144.
48. Li Z, Fu Z, Li L. Advances in agricultural plant protection technology based on machine vision. Transactions of Chinese Society for Agricultural Machinery 2005; 36(8): 143–146.
49. Wang W. Application of machine vision technique in modern agriculture. Modern Agricultural Science and Technology 2014; 8: 335, 340.
50. Zhang J, Wu K , Song P, et al. Image segmentation of maize haploid seeds based on BP neural network. Journal of Jiangsu University (Natural Science Edition) 2011; 32(6): 621–625.
51. Wu X, Chen X, Wu W, et al. Application of information technology in agricultural technology extension. Journal of Chinese Agricultural Mechanization 2013; 34(5): 217–223, 264.
Refbacks
- There are currently no refbacks.
Copyright (c) 2021 Hang Zhou, Zhilong Du, Zhanyuan Wu, Cheng Song, Nan Guo, Yaling Lin

This work is licensed under a Creative Commons Attribution 4.0 International License.
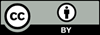
This site is licensed under a Creative Commons Attribution 4.0 International License (CC BY 4.0).
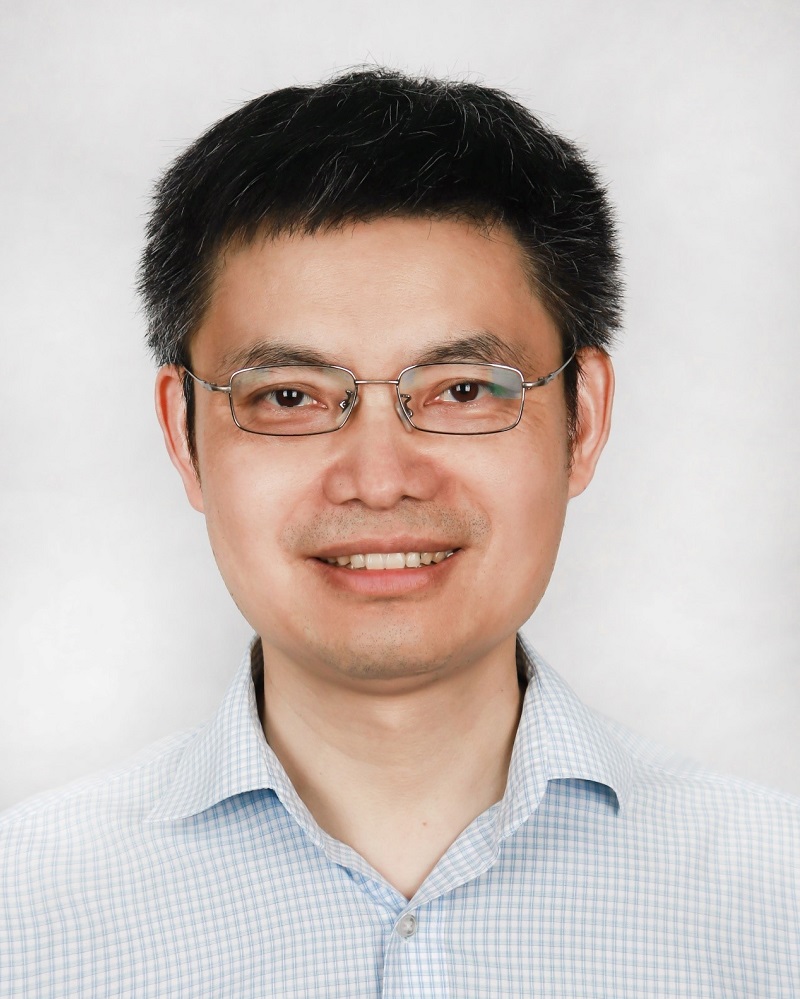
Prof. Zhengjun Qiu
Zhejiang University, China
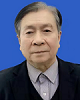
Cheng Sun
Academician of World Academy of Productivity Science; Executive Chairman, World Confederation of Productivity Science China Chapter, China
Processing Speed
-
-
-
- <5 days from submission to initial review decision;
- 64% acceptance rate
-
-
Modern agricultural technology is evolving rapidly, with scientists collaborating with leading agricultural enterprises to develop intelligent management practices. These practices utilize advanced systems that provide tailored fertilization and treatment options for large-scale land management.
This journal values human initiative and intelligence, and the employment of AI technologies to write papers that replace the human mind is expressly prohibited. When there is a suspicious submission that uses AI tools to quickly piece together and generate research results, the editorial board of the journal will reject the article, and all journals under the publisher's umbrella will prohibit all authors from submitting their articles.
Readers and authors are asked to exercise caution and strictly adhere to the journal's policy regarding the usage of Artificial Intelligence Generated Content (AIGC) tools.
Asia Pacific Academy of Science Pte. Ltd. (APACSCI) specializes in international journal publishing. APACSCI adopts the open access publishing model and provides an important communication bridge for academic groups whose interest fields include engineering, technology, medicine, computer, mathematics, agriculture and forestry, and environment.