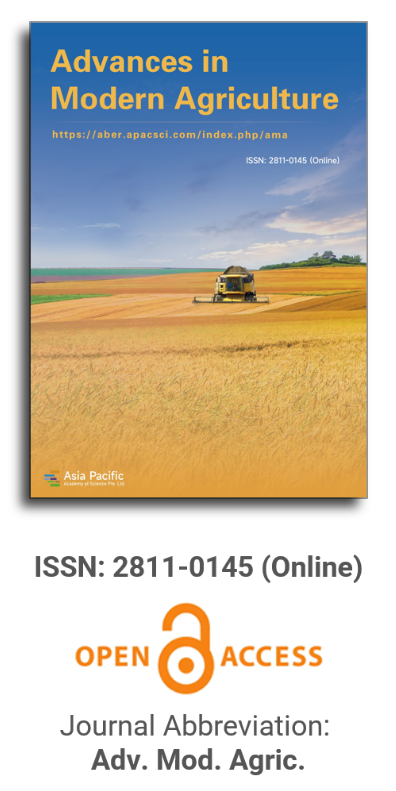
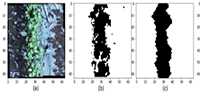
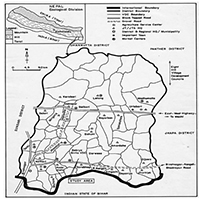
Identification of citrus orchard under vegetation indexes using multi-temporal remote sensing
Vol 3, Issue 1, 2022
VIEWS - 4521 (Abstract)
Download PDF
Abstract
Citrus is widely planted in southern China. Due to cloudy and rainy weather, complex planting types, and other factors, it is difficult to use spectral information to directly identify citrus orchard information. Based on the unique phenological characteristics of citrus, this study put forward the hypothesis that “the vegetation information of citrus orchards may be weakened during the growth and expansion of citrus fruit”. According to this feature, a method of citrus orchard information identification is proposed, and the threshold of the key time window is determined. Taking Wuming District, Nanning City, and Guangxi Zhuang Autonomous Region as the research area, an empirical study on remote sensing identification of citrus orchard information is carried out. First, multi-temporal Sentinel-2 remote sensing images of the study area in 2018 were obtained, and a normalized difference vegetation index was constructed. NDVI, Green Normalized Difference Vegetation Index (GNDVI), Difference Vegetation Index (DVI), Sentinel-derived red-edge spectral indices (RESI), and other vegetation spectral indices Secondly, according to the ground sample point information, the difference in remote sensing vegetation information of different vegetation types in different periods was compared, and then the optimal features of citrus orchard identification were determined. The results showed that there was no significant difference in spectral characteristics between citrus orchards and other major crop types in the study area (such as sugarcane, banana, corn, rice, etc.), but the multi-temporal remote sensing vegetation index of the study area showed that the NDVI of citrus orchards in October was 0.47 lower than that of November, which was significantly lower than that of other crop types. In October, the GNDVI of the citrus orchard also showed a low value of 0.43, but the difference was not obvious compared with other months. However, the dispersion degree of citrus orchard DVI was low, and the separation was not strong. According to the crop phenological calendar, the period of rapid expansion of citrus fruits was from September to October, which verified the scientific hypothesis proposed in this study that the vegetation information of citrus orchards would be weakened during this period. The dispersion degree of different vegetation indexes in the citrus fruit expansion stage was obviously different, and the dispersion degree of NDVI was the highest, and the difference was the strongest. According to the phenological characteristics of the citrus orchard NDVI in October, to further build the normalized index, by using the threshold value method to identify the spatial distribution of the citrus orchard, the identification method had an overall accuracy of 82.75%, better than other identification results of vegetation index. The results of the study for citrus orchard information and remote sensing identification research provide better support for theory and practice.
Keywords
References
1. Zhao T. The Study on Agricultural Climate Regionalization of Citrus in Chongqing Province Based on GIS (Chinese) [Master’s thesis]. Chongqing Normal University; 2018.
2. Bai Q, Huo Z, Wang J, et al. Progress in research on meteorological disaster indicators of major fruit trees in China (Chinese). Journal of Fruit Science 2019; 36(9): 1229–1243.
3. Lan H, Deng X. Industrial poverty alleviation mode supported by research team of pomology discipline-Based on the cases by citrus team of Huazhong Agricultural University (Chinese). Journal of Agricultural Science and Technology 2019; 21(4): 1–7.
4. Liu Y. Study on the Information Extraction of Citrus Field in South of Jiangxi Province and the Estimation of Chlorophyll by Remote Sensing (Chinese) [Master’s thesis]. Jiangxi University of Science and Technology; 2020.
5. Qi C, Gu Y, Zeng Y. Process of citrus industry economy in China (Chinese). Journal of Huazhong Agricultural University 2021; 40(1): 58–69.
6. Xiang Y, Liang X, Lu Q. Comparative advantages and influencing factors of citrus production in Guangxi: An empirical analysis based on the data of 29 major citrus producing counties/districts (Chinese). Chinese Journal of Tropical Agriculture 2020; 40(11): 126–134.
7. Zhu Y, Yang G, Yang H, et al. Identification of apple orchard planting year based on spatiotemporally fused satellite images and clustering analysis of foliage phenophase. Remote Sensing 2020; 12(7): 1199. doi: 10.3390/rs12071199
8. Reis S, Taşdemir K. Identification of hazelnut fields using spectral and Gabor textural features. ISPRS Journal of Photogrammetry and Remote Sensing 2011; 66(5): 652–661. doi: 10.1016/j.isprsjprs.2011.04.006
9. Shrivastava RJ, Gebelein JL. Land cover classification and economic assessment of citrus groves using remote sensing. ISPRS Journal of Photogrammetry and Remote Sensing 2007; 61(5): 341–353. doi: 10.1016/j.isprsjprs.2006.10.003
10. Wang S. Study on the Information Extraction Method of Citrus Orchard Based on Object-Oriented (Chinese) [Master’s thesis]. Jiangxi University of Science and Technology; 2017.
11. Zhang Q, Xu H, Jiang F, Qi S. Spatial distribution characters of citrus orchards in the south of Jiangxi Province (Chinese). Jiangxi Science 2018; 36(4): 591–598.
12. Chen Y, Hu J, Wang Z, Wang Z. An analysis of the dynamic changes in the area of orange orchard in south Jiangxi Province: A case study in Xinfeng County (Chinese). Acta Agriculturae Universitatis Jiangxiensis 2017; 39(5): 884–894.
13. Chen Y, Hu J, Wang Z, Lian W. Research on dynamic changes of Gannan citrus orchard areas: A case study in Xunwu County (Chinese). Journal of Jiangxi University of Science and Technology 2017; 38(5): 19–25.
14. Xu H, Liu C, Qi S, Zhao G. The detection of citrus orchards in southern Jiangxi Province with Landsat images using random forest classifier (Chinese). Journal of Jiangxi Normal University (Natural Science Edition) 2018; 42(4): 434–440.
15. Ren C, Huang W, Ye H, Cui B. Extraction of banana orchards based on GF-2 satellite imagery and its accuracy analysis (Chinese). Remote Sensing of Information 2017; 32(6): 78–84.
16. Song R, Ning J, Chang Q, et al. Kiwifruit orchard mapping based on wavelet textures and random forest (Chinese). Transactions of the Chinese Society for Agricultural Machinery 2018; 49(4): 222–231.
17. Song Q, Hu Q, Zhou Q, et al. In-season crop mapping with GF-1/WFV data by combining object-based image analysis and random forest. Remote Sensing 2017; 9(11): 1184. doi: 10.3390/rs9111184
18. Talema T, Hailu BT. Mapping rice crop using sentinels (1 SAR and 2 MSI) images in tropical area: A case study in Fogera wereda, Ethiopia. Remote Sensing Applications: Society and Environment 2020; 18: 100290. doi: 10.1016/j.rsase.2020.100290
19. Dong J, Xiao X, Menarguez MA, et al. Mapping paddy rice planting area in northeastern Asia with Landsat 8 images, phenology-based algorithm and Google Earth Engine. Remote Sensing of Environment 2016; 185: 142–154. doi: 10.1016/j.rse.2016.02.016
20. d’Andrimont R, Taymans M, Lemoine G, et al. Detecting flowering phenology in oil seed rape parcels with Sentinel-1 and -2 time series. Remote Sensing of Environment 2020; 239: 111660. doi: 10.1016/j.rse.2020.111660
21. Liang S, Chen J, Wu B. Extracting rubber plantation with decision tree model based on object-oriented method. National Remote Sensing Bulletin 2015; 19(3): 485–494. doi: 10.11834/jrs.20153337
22. Li L Li N, Lu D. Mapping tea gardens spatial distribution in northwestern Zhejiang Province using multi-temporal Sentinel-2 imagery (Chinese). Journal of Zhejiang A&F University 2019; 36(5): 841–848.
23. Gong P, Liu H, Zhang M, et al. Stable classification with limited sample: transferring a 30-m resolution sample set collected in 2015 to mapping 10-m resolution global land cover in 2017. Science Bulletin 2019; 64(6): 370–373. doi: 10.1016/j.scib.2019.03.002
24. He Z, Zhang M, Wu B, et al. Extraction of summer crop in Jiangsu based on Google Earth Engine (Chinese). Journal of Geo-information Science 2019; 21(5): 126–140.
25. Zhong Q, Luo J, Qi S. Sample sensitivity analysis of citrus orchards extracted by random forest classification algorithm (Chinese). Jiangxi Science 2019; 37(5): 664–669.
26. Silva Junior CA da, Leonel-Junior AHS, Rossi FS, et al. Mapping soybean planting area in Midwest Brazil with remotely sensed images and phenology-based algorithm using the Google Earth Engine platform. Computers and Electronics in Agriculture 2020; 169: 105194. doi: 10.1016/j.compag.2019.105194
27. Xu B, Ma H, Li J, et al. Remote sensing dynamic estimation of grass production in Xilinguole, Inner Mongolia. Scientia Sinica Vitae 2011; 41(12): 1185–1195. doi: 10.1360/052011-228
28. Xin X, Zhang B, Li G, et al. Variation in spatial pattern of grassland biomass in China from 1982 to 2003 (Chinese). Journal of Natural Resources 2009; 24(9): 1582–1592.
29. Yang H, Tong X. Distribution information extraction of rubber woods using remote sensing images with high resolution (Chinese). Geomatics and Information Science of Wuhan University 2014; 39(4): 411–416+421.
30. Regniers O, Bombrun L, Guyon D, et al. Wavelet-based texture features for the classification of age classes in a maritime pine forest. IEEE Geoscience and Remote Sensing Letters 2015; 12(3): 621–625. doi: 10.1109/lgrs.2014.2353656
31. Qin A. Research on Rural Land Management Mode in Wuming District of Nannnign City Under the Background of Agricultural Industrialization (Chinese) [Master’s thesis]. Guangxi University; 2019.
32. Hu P, Li Z, Li C, et al. The retrieval of citrus spatial distribution in Yidu City using remote sensing (Chinese). Hubei Agricultural Sciences 2014; 53(4): 795–798.
33. Wang L, Kong Y, Yang X, et al. Classification of land use in farming areas based on feature optimization random forest algorithm (Chinese). Transactions of the Chinese Society of Agricultural Engineering 2020; 36(4): 244–250.
34. Han W, Peng X, Zhang L, et al. Summer maize yield estimation based on vegetation index derived from multi-temporal UAV remote sensing (Chinese). Transactions of the Chinese Society for Agricultural Machinery 2020; 51(1): 148–155.
35. Deng J, Gu H, Wang Z, et al. Estimation and validation of above-ground biomass of cotton during main growth period using Unmanned Aerial Vehicle (UAV) (Chinese). Agricultural Research in the Arid Areas 2019; 37(5): 55–61.
36. Xiao C, Li P, Feng Z, et al. Sentinel-2 red-edge spectral indices (RESI) suitability for mapping rubber boom in Luang Namtha Province, northern Lao PDR. International Journal of Applied Earth Observation and Geoinformation 2020; 93: 102176. doi: 10.1016/j.jag.2020.102176
37. Meng L, Zhong S, Mo J, et al. Research on eucalyptus information extraction based on GF-1 object-oriented (Chinese). Geospatial Information 2019; 17(8): 24–29.
38. Li Y, Zhang J, Xue Y, Zhang P. Remote sensing image extraction for rubber forest distribution in the border regions of China, Laos and Myanmar based on Google Earth Engine platform (Chinese). Transactions of the Chinese Society of Agricultural Engineering 2020; 36(8): 174–181.
39. Xu H, Liu C, Wang J, et al. Study on extraction of citrus orchard in Gannan region based on Google Earth Engine platform (Chinese). Journal of Geo-Information Science 2018; 20(3): 396–404.
Refbacks
- There are currently no refbacks.
Copyright (c) 2022 Chenxin Liang, Qiting Huang, Si Wang, Cong Wan, Qiangyi Yu, Wenbin Wu

This work is licensed under a Creative Commons Attribution 4.0 International License.
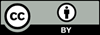
This site is licensed under a Creative Commons Attribution 4.0 International License (CC BY 4.0).
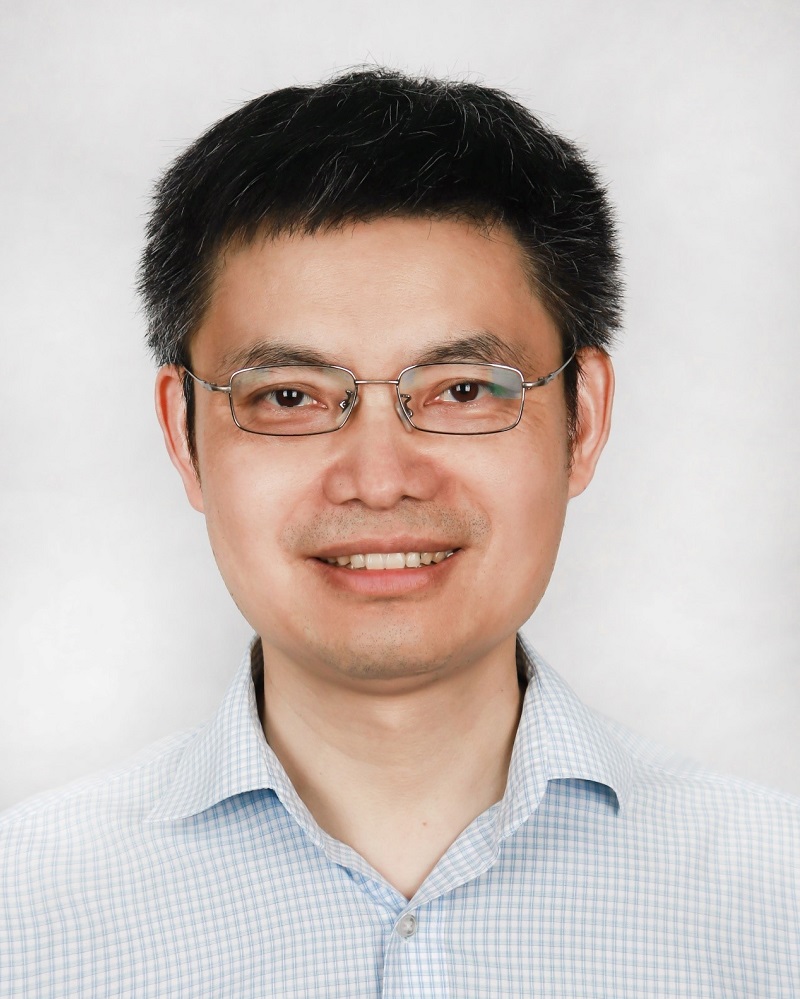
Prof. Zhengjun Qiu
Zhejiang University, China
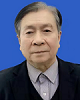
Cheng Sun
Academician of World Academy of Productivity Science; Executive Chairman, World Confederation of Productivity Science China Chapter, China
Indexing & Archiving
In the realm of modern agriculture, the integration of cutting-edge technologies is revolutionizing the way we approach sustainable farming practices. A recent study published in Advances in Modern Agriculture titled "Classification of cotton water stress using convolutional neural networks and UAV-based RGB imagery" has garnered significant attention for its innovative approach to precision irrigation management. Conducted by researchers from Institute of Data Science and the AgriLife Research and Extension Center of Texas A&M University (authors's information is below). This study introduces a novel method for classifying cotton water stress using unmanned aerial vehicles (UAVs) and convolutional neural networks (CNNs), offering a powerful solution for optimizing water use in agriculture.
Modern agricultural technology is evolving rapidly, with scientists collaborating with leading agricultural enterprises to develop intelligent management practices. These practices utilize advanced systems that provide tailored fertilization and treatment options for large-scale land management.
This journal values human initiative and intelligence, and the employment of AI technologies to write papers that replace the human mind is expressly prohibited. When there is a suspicious submission that uses AI tools to quickly piece together and generate research results, the editorial board of the journal will reject the article, and all journals under the publisher's umbrella will prohibit all authors from submitting their articles.
Readers and authors are asked to exercise caution and strictly adhere to the journal's policy regarding the usage of Artificial Intelligence Generated Content (AIGC) tools.
Asia Pacific Academy of Science Pte. Ltd. (APACSCI) specializes in international journal publishing. APACSCI adopts the open access publishing model and provides an important communication bridge for academic groups whose interest fields include engineering, technology, medicine, computer, mathematics, agriculture and forestry, and environment.