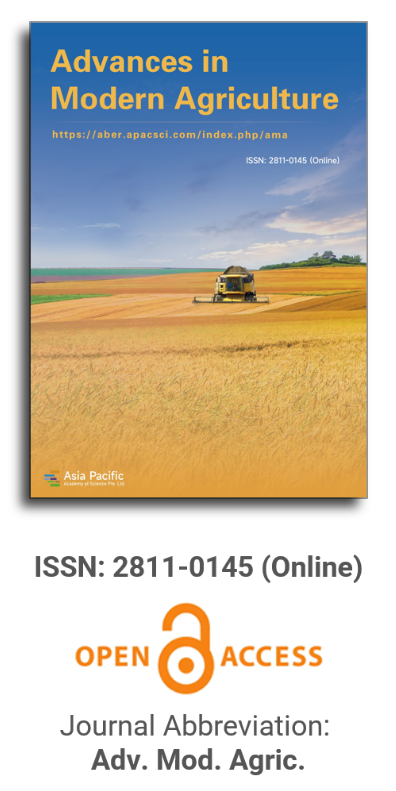
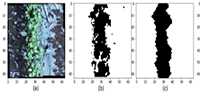
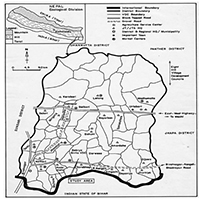
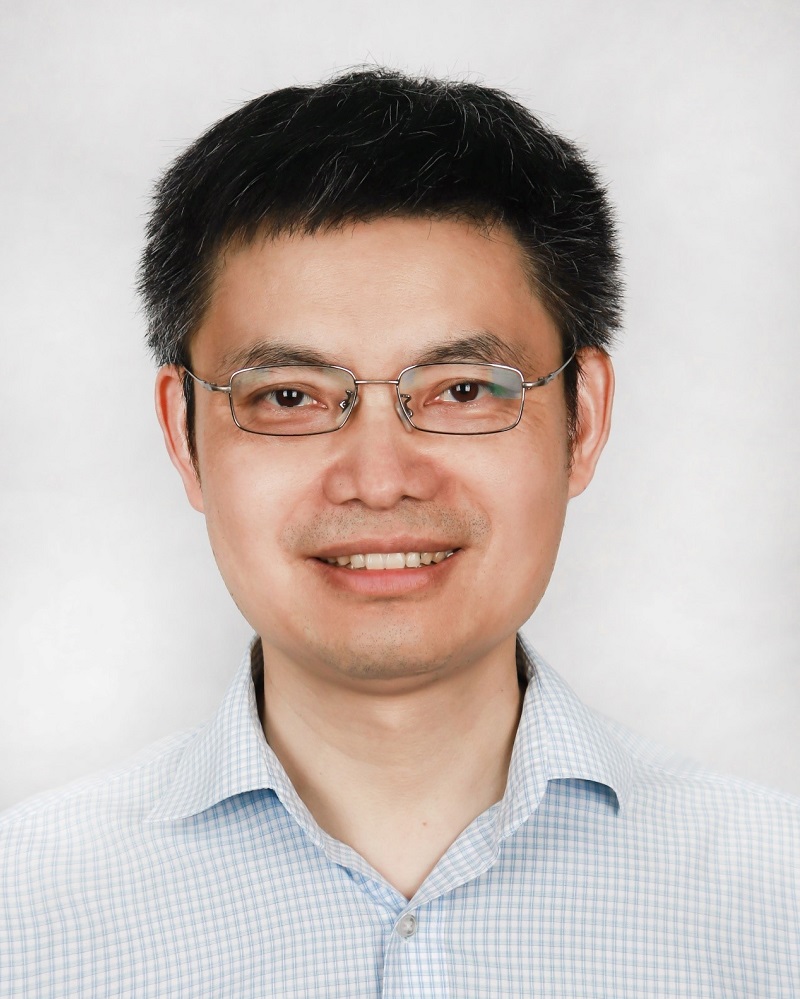
Prof. Zhengjun Qiu
Zhejiang University, China
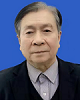
Cheng Sun
Academician of World Academy of Productivity Science; Executive Chairman, World Confederation of Productivity Science China Chapter, China
Indexing & Archiving
In the realm of modern agriculture, the integration of cutting-edge technologies is revolutionizing the way we approach sustainable farming practices. A recent study published in Advances in Modern Agriculture titled "Classification of cotton water stress using convolutional neural networks and UAV-based RGB imagery" has garnered significant attention for its innovative approach to precision irrigation management. Conducted by researchers from Institute of Data Science and the AgriLife Research and Extension Center of Texas A&M University (authors's information is below). This study introduces a novel method for classifying cotton water stress using unmanned aerial vehicles (UAVs) and convolutional neural networks (CNNs), offering a powerful solution for optimizing water use in agriculture.
Modern agricultural technology is evolving rapidly, with scientists collaborating with leading agricultural enterprises to develop intelligent management practices. These practices utilize advanced systems that provide tailored fertilization and treatment options for large-scale land management.
This journal values human initiative and intelligence, and the employment of AI technologies to write papers that replace the human mind is expressly prohibited. When there is a suspicious submission that uses AI tools to quickly piece together and generate research results, the editorial board of the journal will reject the article, and all journals under the publisher's umbrella will prohibit all authors from submitting their articles.
Readers and authors are asked to exercise caution and strictly adhere to the journal's policy regarding the usage of Artificial Intelligence Generated Content (AIGC) tools.
Asia Pacific Academy of Science Pte. Ltd. (APACSCI) specializes in international journal publishing. APACSCI adopts the open access publishing model and provides an important communication bridge for academic groups whose interest fields include engineering, technology, medicine, computer, mathematics, agriculture and forestry, and environment.