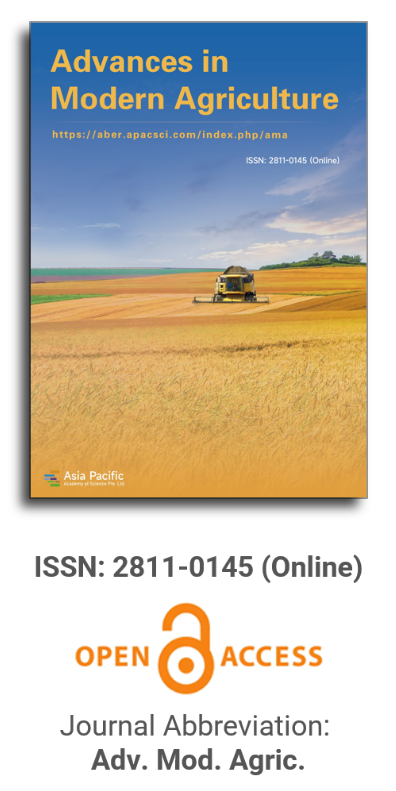
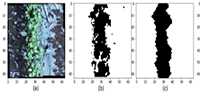
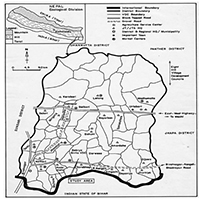
Development and evaluation of smart irrigation system to enhance the water use efficiency
Vol 4, Issue 2, 2023
VIEWS - 2705 (Abstract)
Download PDF
Abstract
For this purpose, a moisture sensor device was designed and constructed in February and March 2019 to determine the appropriate time to stop irrigation in furrow irrigation. Testing the device in the laboratory and its application in the Farm of the Campus of Agriculture and Natural Resources, University of Tehran (Mohammad Shahr), Iran, from April to July 2019. The purpose of this study was to evaluate the performance of a smart sensor of soil moisture to determine the optimum depth of installation and recording of soil moisture at 10, 30, and 50 cm depths and different length ratios in furrow irrigation. Initially, calibration of the device was carried out on field soil, and based on the obtained validation, the device was transferred to the field. To achieve the goals of optimum depth of installation and optimum length, 36-meter furrows with a distance of 0.75 m were created in the field. Sensitive lengths in furrows with 0.5 L, 0.75 L, and 0.85 L ratios were selected as the starting points. The results showed that in the calibration and validation phases, the R2 values were 0.93 and 0.95, respectively, and in the calibration and validation stages, the value of nRMSE was 80 and 13.81%, indicating good model training in the calibration stage. Also, the average RE parameter in estimating soil moisture was 2.74%, indicating the high accuracy of the device in estimating soil moisture. The results also showed that if the device was installed at a depth of 30 cm from the soil surface of the furrow and at 75% from the beginning of the field, the depth and runoff losses would be minimal and irrigation adequacy would be best compared to other depths and lengths. It is expected that with optimal water consumption and timely interruption of irrigation, deep losses and runoff will be avoided, and with low water consumption, the productivity of crops will increase.
Keywords
References
Refbacks
- There are currently no refbacks.
Copyright (c) 2023 Masoud Pourgholam-Amiji, Abdolmajid Liaghat, Farnaz Nozari
License URL: https://creativecommons.org/licenses/by/4.0/
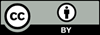
This site is licensed under a Creative Commons Attribution 4.0 International License (CC BY 4.0).
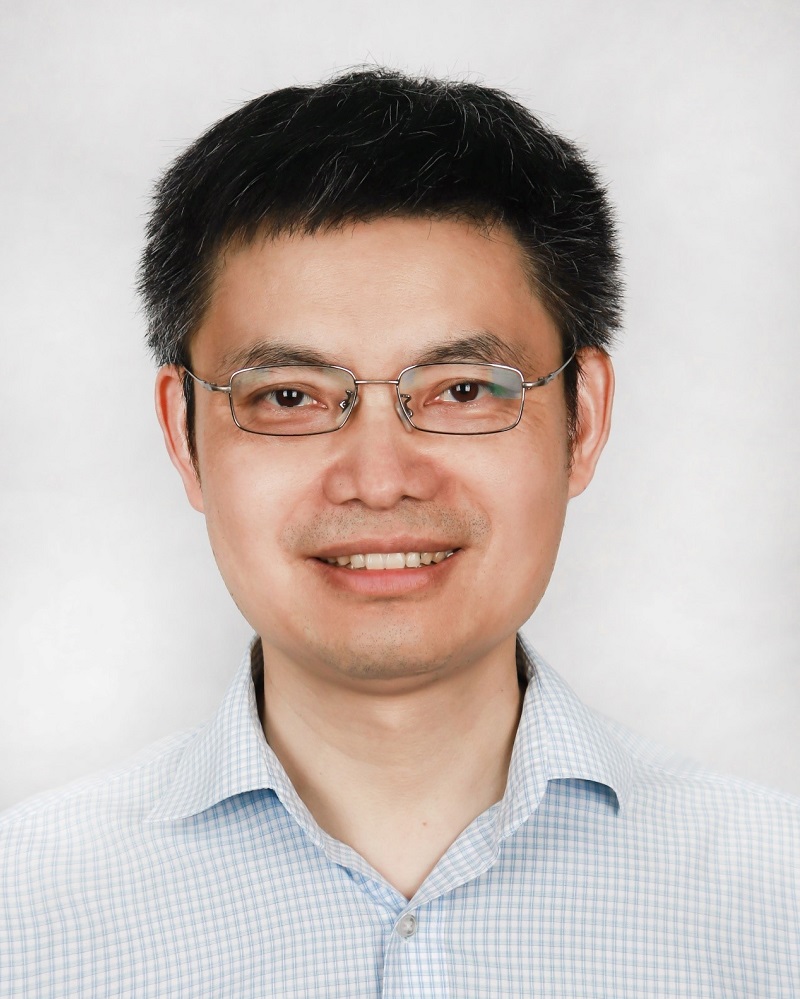
Prof. Zhengjun Qiu
Zhejiang University, China
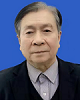
Cheng Sun
Academician of World Academy of Productivity Science; Executive Chairman, World Confederation of Productivity Science China Chapter, China
Indexing & Archiving
In the realm of modern agriculture, the integration of cutting-edge technologies is revolutionizing the way we approach sustainable farming practices. A recent study published in Advances in Modern Agriculture titled "Classification of cotton water stress using convolutional neural networks and UAV-based RGB imagery" has garnered significant attention for its innovative approach to precision irrigation management. Conducted by researchers from Institute of Data Science and the AgriLife Research and Extension Center of Texas A&M University (authors's information is below). This study introduces a novel method for classifying cotton water stress using unmanned aerial vehicles (UAVs) and convolutional neural networks (CNNs), offering a powerful solution for optimizing water use in agriculture.
Modern agricultural technology is evolving rapidly, with scientists collaborating with leading agricultural enterprises to develop intelligent management practices. These practices utilize advanced systems that provide tailored fertilization and treatment options for large-scale land management.
This journal values human initiative and intelligence, and the employment of AI technologies to write papers that replace the human mind is expressly prohibited. When there is a suspicious submission that uses AI tools to quickly piece together and generate research results, the editorial board of the journal will reject the article, and all journals under the publisher's umbrella will prohibit all authors from submitting their articles.
Readers and authors are asked to exercise caution and strictly adhere to the journal's policy regarding the usage of Artificial Intelligence Generated Content (AIGC) tools.
Asia Pacific Academy of Science Pte. Ltd. (APACSCI) specializes in international journal publishing. APACSCI adopts the open access publishing model and provides an important communication bridge for academic groups whose interest fields include engineering, technology, medicine, computer, mathematics, agriculture and forestry, and environment.