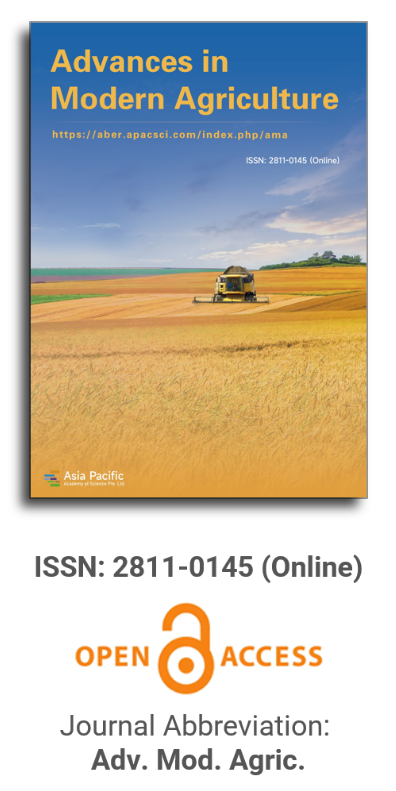
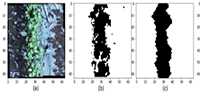
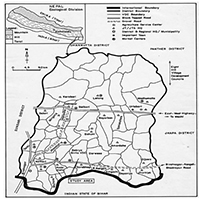
Operation performance test and analysis of 4GQ–1C sugar-cane harvester
Vol 2, Issue 1, 2021
VIEWS - 6113 (Abstract)
Download PDF
Abstract
4GQ-1C sugarcane harvester was designed to solve the current problems that large and medium-sized sugarcane harvesters had low adaptability in sloping, small row spacing, and small plots of sugarcane harvesting. In order to verify the adaptability and reliability of 4GQ-1C sugarcane harvester, field tests were carried out and compared with existing models. Results showed that the 4GQ-1C sugarcane harvester has good operation performance with a lower impurity rate and loss rate of sugarcane, stronger harvesting adaptability in small row spacing areas, and is more convenient in collecting sugarcane compared with sugarcane harvesters with power of 132 and 194 kw. Furthermore, 4GQ-1C sugarcane harvester is compact and flexible, with good adaptability and a good harvest effect in sugarcane growing areas with small and medium-sized planting scales, making it worth popularizing and applying to sugarcane harvest.
Keywords
References
Refbacks
- There are currently no refbacks.
Copyright (c) 2021 Weifeng Huang, Bosheng Zeng, Jianlin Mo, Zizeng Yang, Dejun Meng

This work is licensed under a Creative Commons Attribution 4.0 International License.
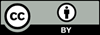
This site is licensed under a Creative Commons Attribution 4.0 International License (CC BY 4.0).
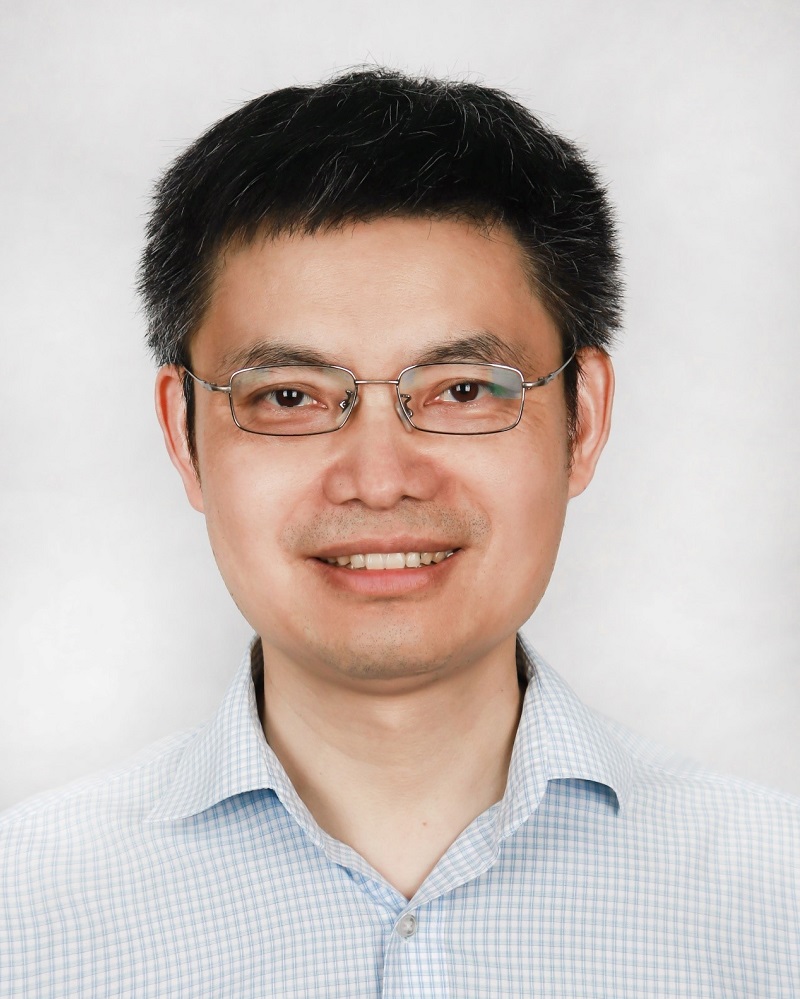
Prof. Zhengjun Qiu
Zhejiang University, China
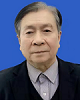
Cheng Sun
Academician of World Academy of Productivity Science; Executive Chairman, World Confederation of Productivity Science China Chapter, China
Indexing & Archiving
In the realm of modern agriculture, the integration of cutting-edge technologies is revolutionizing the way we approach sustainable farming practices. A recent study published in Advances in Modern Agriculture titled "Classification of cotton water stress using convolutional neural networks and UAV-based RGB imagery" has garnered significant attention for its innovative approach to precision irrigation management. Conducted by researchers from Institute of Data Science and the AgriLife Research and Extension Center of Texas A&M University (authors's information is below). This study introduces a novel method for classifying cotton water stress using unmanned aerial vehicles (UAVs) and convolutional neural networks (CNNs), offering a powerful solution for optimizing water use in agriculture.
Modern agricultural technology is evolving rapidly, with scientists collaborating with leading agricultural enterprises to develop intelligent management practices. These practices utilize advanced systems that provide tailored fertilization and treatment options for large-scale land management.
This journal values human initiative and intelligence, and the employment of AI technologies to write papers that replace the human mind is expressly prohibited. When there is a suspicious submission that uses AI tools to quickly piece together and generate research results, the editorial board of the journal will reject the article, and all journals under the publisher's umbrella will prohibit all authors from submitting their articles.
Readers and authors are asked to exercise caution and strictly adhere to the journal's policy regarding the usage of Artificial Intelligence Generated Content (AIGC) tools.
Asia Pacific Academy of Science Pte. Ltd. (APACSCI) specializes in international journal publishing. APACSCI adopts the open access publishing model and provides an important communication bridge for academic groups whose interest fields include engineering, technology, medicine, computer, mathematics, agriculture and forestry, and environment.