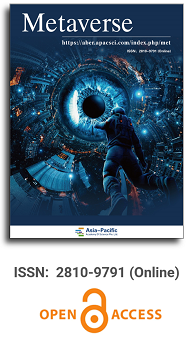
Asia Pacific Academy of Science Pte. Ltd. (APACSCI) specializes in international journal publishing. APACSCI adopts the open access publishing model and provides an important communication bridge for academic groups whose interest fields include engineering, technology, medicine, computer, mathematics, agriculture and forestry, and environment.
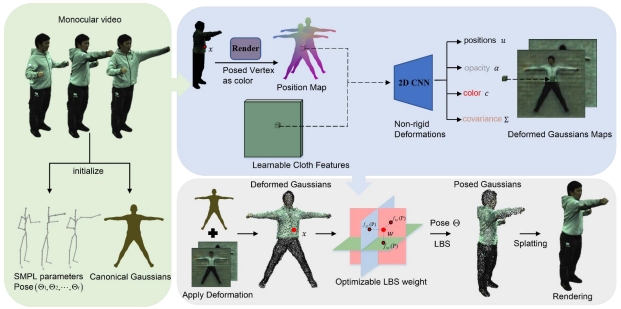
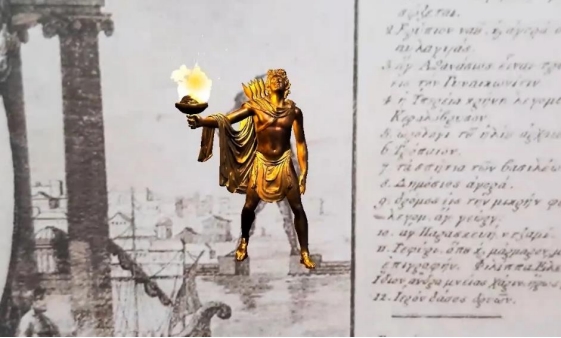
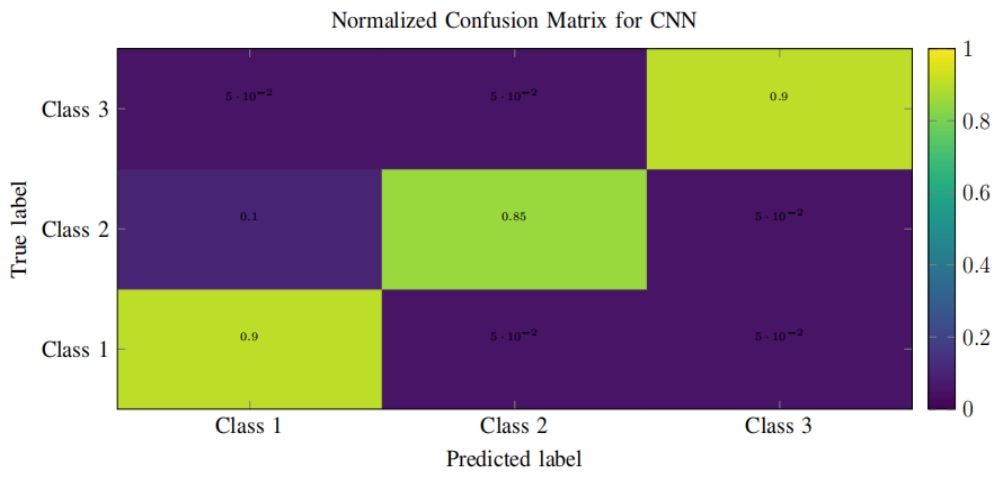
Integration of Leap Motion sensor with camera for better performance in hand tracking
Vol 5, Issue 2, 2024
Download PDF
Abstract
In this paper, we propose a framework for hand tracking in human-computer interaction applications. Leap Motion is used today as a popular interface in virtual reality and computer games. In this study, we evaluated the merits and drawbacks of this device. The limitations of this device restrict the user’s free movement. The purpose of this study is to find an optimal solution for using Leap Motion. We propose a framework to estimate the pose of the hand in a bigger space around Leap Motion. Our framework uses an integration of Leap Motion with a camera that are placed in two different places to capture the information of the hand from various views. The experiments are designed based on the common tasks in the human-computer interaction applications. The finding of this study demonstrates that the proposed framework increases the precise interaction space.
Keywords
References
- Mahdikhanlou K, Ebrahimnezhad H. Multimodal 3D American sign language recognition for static alphabet and numbers using hand joints and shape coding. Multimedia Tools and Applications. 2020; 79(31–32): 22235–22259. doi: 10.1007/s11042-020-08982-8
- Elakkiya R, Selvamani K. Subunit sign modeling framework for continuous sign language recognition. Computers & Electrical Engineering. 2019; 74: 379–390. doi: 10.1016/j.compeleceng.2019.02.012
- Mahdikhanlou K, Ebrahimnezhad H. Object manipulation and deformation using hand gestures. Journal of Ambient Intelligence and Humanized Computing. 2021; 14(7): 8115–8133. doi: 10.1007/s12652-021-03582-2
- Feng Z, Yang B, Tang H, et al. Behavioral-model-based freehand tracking in a Selection-Move-Release system. Computers & Electrical Engineering. 2014; 40(6): 1827–1837. doi: 10.1016/j.compeleceng.2014.05.014
- Chattaraj R, Khan S, Roy DG, et al. Vision-based human grasp reconstruction inspired by hand postural synergies. Computers & Electrical Engineering. 2018; 70: 702–721. doi: 10.1016/j.compeleceng.2017.10.018
- Yongda D, Fang L, Huang X. Research on multimodal human-robot interaction based on speech and gesture. Computers & Electrical Engineering. 2018; 72: 443–454. doi: 10.1016/j.compeleceng.2018.09.014
- Cui Y, Song X, Hu Q, et al. Human-robot interaction in higher education for predicting student engagement. Computers and Electrical Engineering. 2022; 99: 107827. doi: 10.1016/j.compeleceng.2022.107827
- Gupta R, Kumar A. Indian sign language recognition using wearable sensors and multi-label classification. Computers & Electrical Engineering. 2021; 90: 106898. doi: 10.1016/j.compeleceng.2020.106898
- Połap D, Kęsik K, Winnicka A, et al. Strengthening the perception of the virtual worlds in a virtual reality environment. ISA Transactions. 2020; 102: 397–406. doi: 10.1016/j.isatra.2020.02.023
- Curiel-Razo YI, Icasio-Hernández O, Sepúlveda-Cervantes G, et al. Leap motion controller three dimensional verification and polynomial correction. Measurement. 2016; 93: 258–264. doi: 10.1016/j.measurement.2016.07.017
- Oropesa I, de Jong TL, Sánchez-González P, et al. Feasibility of tracking laparoscopic instruments in a box trainer using a Leap Motion Controller. Measurement. 2016; 80: 115–124. doi: 10.1016/j.measurement.2015.11.018
- Quesada L, López G, Guerrero L. Automatic recognition of the American sign language fingerspelling alphabet to assist people living with speech or hearing impairments. Journal of Ambient Intelligence and Humanized Computing. 2017; 8(4): 625–635. doi: 10.1007/s12652-017-0475-7
- Ponraj G, Ren H. Sensor Fusion of Leap Motion Controller and Flex Sensors Using Kalman Filter for Human Finger Tracking. IEEE Sensors Journal. 2018; 18(5): 2042–2049. doi: 10.1109/jsen.2018.2790801
- Guna J, Jakus G, Pogačnik M, et al. An Analysis of the Precision and Reliability of the Leap Motion Sensor and Its Suitability for Static and Dynamic Tracking. Sensors. 2014; 14(2): 3702–3720. doi: 10.3390/s140203702
- Ameur S, Ben Khalifa A, Bouhlel MS. Chronological pattern indexing: An efficient feature extraction method for hand gesture recognition with Leap Motion. Journal of Visual Communication and Image Representation. 2020; 70: 102842. doi: 10.1016/j.jvcir.2020.102842
- Jiang X, Xiao ZG, Menon C. Virtual grasps recognition using fusion of Leap Motion and force myography. Virtual Reality. 2018; 22(4): 297–308. doi: 10.1007/s10055-018-0339-2
- Wang Y, Wu Y, Jung S, et al. Enlarging the Usable Hand Tracking Area by Using Multiple Leap Motion Controllers in VR. IEEE Sensors Journal. 2021; 21(16): 17947–17961. doi: 10.1109/jsen.2021.3082988
- Jawahar CV, Li H, Mori G, et al. Computer Vision—ACCV 2018. Springer International Publishing; 2019.
- Mueller F, Bernard F, Sotnychenko O, et al. GANerated Hands for Real-Time 3D Hand Tracking from Monocular RGB. In: Proceedings of 2018 IEEE/CVF Conference on Computer Vision and Pattern Recognition; 18–23 June 2018; Salt Lake City, USA. pp. 49–59.
- Zhang J, Jiao J, Chen M, et al. A hand pose tracking benchmark from stereo matching. In: Proceedings of 2017 IEEE International Conference on Image Processing (ICIP); 17–20 September 2017; Beijing, China.
- Zimmermann C, Brox T. Learning to Estimate 3D Hand Pose from Single RGB Images. In: Proceedings of 2017 IEEE International Conference on Computer Vision (ICCV); 22–29 October 2017; Venice, Italy.
- Garcia-Hernando G, Yuan S, Baek S, et al. First-Person Hand Action Benchmark with RGB-D Videos and 3D Hand Pose Annotations. In: Proceedings of 2018 IEEE/CVF Conference on Computer Vision and Pattern Recognition; 18–23 June 2018; Salt Lake City, USA.
- Bambach S, Lee S, Crandall DJ, et al. Lending A Hand: Detecting Hands and Recognizing Activities in Complex Egocentric Interactions. In: Proceedings of 2015 IEEE International Conference on Computer Vision (ICCV); 7–13 December 2015; Santiago, Chile. pp. 1949–1957.
- Rogez, G., Khademi, M., Supančič, J.S., et al. 3D Hand Pose Detection in Egocentric RGB-D Images. In: Agapito, L., Bronstein, M., Rother, C. Computer Vision - ECCV 2014 Workshops. Lecture Notes in Computer Science, vol 8925. Springer International Publishing; 2015.
- Yuan S, Ye Q, Stenger B, et al. BigHand2.2M Benchmark: Hand Pose Dataset and State of the Art Analysis. In: Proceedings of 2017 IEEE Conference on Computer Vision and Pattern Recognition (CVPR); 21–26 July 2017; Honolulu, USA.
- Cai, Y., Ge, L., Cai, J., et al. Weakly-Supervised 3D Hand Pose Estimation from Monocular RGB Images. In: Ferrari, V., Hebert, M., Sminchisescu, C., et al. Computer Vision – ECCV 2018. Lecture Notes in Computer Science, vol 11210. Springer International Publishing; 2018.
- Bengio Y, Goodfellow I, Courville A. Deep learning. MIT press; 2017.
Refbacks
- There are currently no refbacks.
Copyright (c) 2024 Khadijeh Mahdikhanlou, Hossein Ebrahimnezhad
License URL: https://creativecommons.org/licenses/by/4.0/
This site is licensed under a Creative Commons Attribution 4.0 International License (CC BY 4.0).
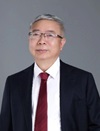
Prof. Zhigeng Pan
Director, Institute for Metaverse, Nanjing University of Information Science & Technology, China
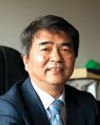
Prof. Jianrong Tan
Academician, Chinese Academy of Engineering, China
Conference Time
December 15-18, 2025
Conference Venue
Hong Kong Convention and Exhibition Center (HKCEC)
...
Metaverse Scientist Forum No.3 was successfully held on April 22, 2025, from 19:00 to 20:30 (Beijing Time)...
We received the Scopus notification on April 19th, confirming that the journal has been successfully indexed by Scopus...
We are pleased to announce that we have updated the requirements for manuscript figures in the submission guidelines. Manuscripts submitted after April 15, 2025 are required to strictly adhere to the change. These updates are aimed at ensuring the highest quality of visual content in our publications and enhancing the overall readability and impact of your research. For more details, please find it in sumissions...