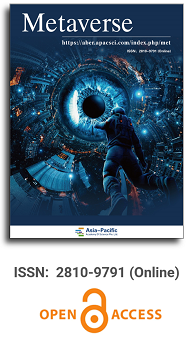
Asia Pacific Academy of Science Pte. Ltd. (APACSCI) specializes in international journal publishing. APACSCI adopts the open access publishing model and provides an important communication bridge for academic groups whose interest fields include engineering, technology, medicine, computer, mathematics, agriculture and forestry, and environment.
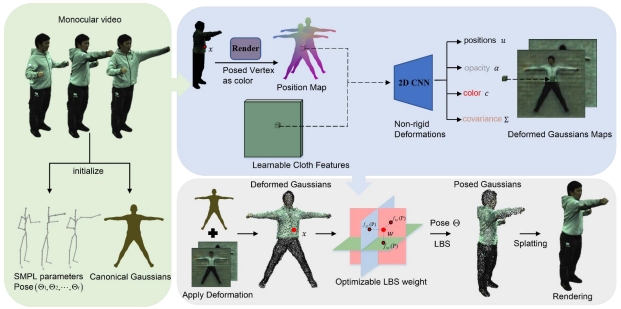
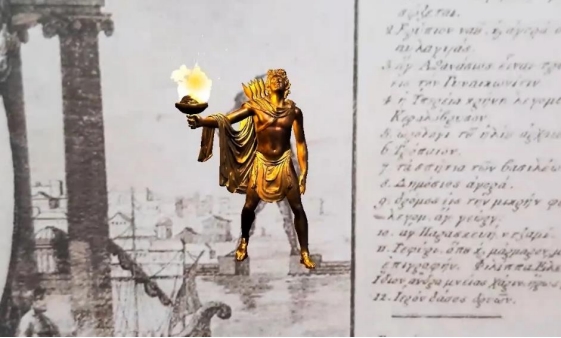
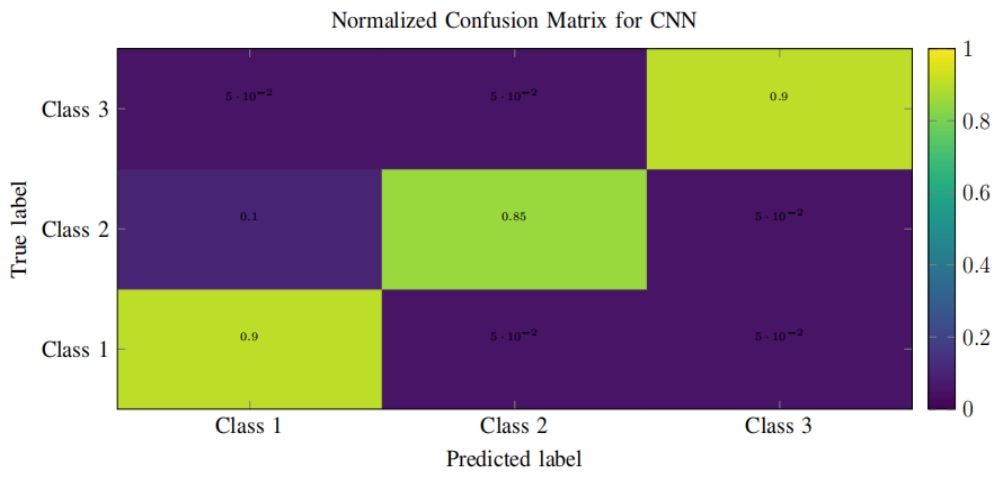
Garment-aware gaussian for clothed human modeling from monocular video
Vol 6, Issue 2, 2025
Download PDF
Abstract
Reconstructing the human body from monocular video input presents significant challenges, including a limited field of view and difficulty in capturing non-rigid deformations, such as those associated with clothing and pose variations. These challenges often compromise motion editability and rendering quality. To address these issues, we propose a cloth-aware 3D Gaussian splatting approach that leverages the strengths of 2D convolutional neural networks (CNNs) and 3D Gaussian splatting for high-quality human body reconstruction from monocular video. Our method parameterizes 3D Gaussians anchored to a human template to generate posed position maps that capture pose-dependent non-rigid deformations. Additionally, we introduce Learnable Cloth Features, which are pixel-aligned with the posed position maps to address cloth-related deformations. By jointly modeling cloth and pose-dependent deformations, along with compact, optimizable linear blend skinning (LBS) weights, our approach significantly enhances the quality of monocular 3D human reconstructions. We also incorporate carefully designed regularization techniques for the Gaussians, improving the generalization capability of our model. Experimental results demonstrate that our method outperforms state-of-the-art techniques for animatable avatar reconstruction from monocular inputs, delivering superior performance in both reconstruction fidelity and rendering quality.
Keywords
References
1. Casas D, Volino M, Collomosse J, et al. 4D video textures for interactive character appearance. Computer Graphics Forum. 2014; 33(2): 371-380. doi: 10.1111/cgf.12296
2. Dong Z, Guo C, Song J, et al. PINA: Learning a Personalized Implicit Neural Avatar from a Single RGB-D Video Sequence. In: Proceedings of the 2022 IEEE/CVF Conference on Computer Vision and Pattern Recognition (CVPR); 2022. pp. 20438-20448.
3. Guo K, Lincoln P, Davidson P, et al. The relightables. ACM Transactions on Graphics. 2019; 38(6): 1-19. doi: 10.1145/3355089.3356571
4. Peng S, Zhang Y, Xu Y, et al. Neural Body: Implicit Neural Representations with Structured Latent Codes for Novel View Synthesis of Dynamic Humans. In: Proceedings of the 2021 IEEE/CVF Conference on Computer Vision and Pattern Recognition (CVPR); 2021. pp. 9050-9059.
5. Weng CY, Curless B, Srinivasan PP, et al. HumanNeRF: Free-viewpoint Rendering of Moving People from Monocular Video. In: Proceedings of the 2022 IEEE/CVF Conference on Computer Vision and Pattern Recognition (CVPR); 2022. pp. 16189-16199.
6. Mildenhall B, Srinivasan PP, Tancik M, et al. NeRF: Representing Scenes as Neural Radiance Fields for View Synthesis. In: Proceedings of the European Conference on Computer Vision; 2020. pp. 405–421.
7. Tancik M, Srinivasan P, Mildenhall B, et al. Fourier Features Let Networks Learn High Frequency Functions in Low Dimensional Domains. In: Proceedings of the 34th International Conference on Neural Information Processing Systems; 2020. pp. 7537–7547.
8. Kerbl B, Kopanas G, Leimkuehler T, et al. 3D Gaussian Splatting for Real-Time Radiance Field Rendering. ACM Transactions on Graphics. 2023; 42(4): 1-14. doi: 10.1145/3592433
9. Zielonka W, Bagautdinov T, Saito S, et al. Drivable 3D Gaussian Avatars. arXiv. 2023; arXiv:2311.08581.
10. Li Z, Zheng Z, Wang L, et al. Animatable Gaussians: Learning Pose-Dependent Gaussian Maps for High-Fidelity Human Avatar Modeling. In: Proceedings of the 2024 IEEE/CVF Conference on Computer Vision and Pattern Recognition (CVPR); 2024. pp. 19711-19722.
11. Xu Y, Chen B, Li Z, et al. Gaussian Head Avatar: Ultra High-Fidelity Head Avatar via Dynamic Gaussians. In: Proceedings of the 2024 IEEE/CVF Conference on Computer Vision and Pattern Recognition (CVPR); 2024. pp. 1931-1941.
12. Jiang Y, Shen Z, Wang P, et al. HiFi4G: High-Fidelity Human Performance Rendering via Compact Gaussian Splatting. In: Proceedings of the 2024 IEEE/CVF Conference on Computer Vision and Pattern Recognition (CVPR). pp. 19734-19745. doi: 10.1109/cvpr52733.2024.01866
13. Wen J, Zhao X, Ren Z, et al. GoMAvatar: Efficient Animatable Human Modeling from Monocular Video Using Gaussians-on-Mesh. In: Proceedings of the 2024 IEEE/CVF Conference on Computer Vision and Pattern Recognition (CVPR); 2024. pp. 2059-2069.
14. Hu S, Hu T, Liu Z. GauHuman: Articulated Gaussian Splatting from Monocular Human Videos. In: Proceedings of the 2024 IEEE/CVF Conference on Computer Vision and Pattern Recognition (CVPR); 2024. pp. 20418-20431.
15. Wang L, Zhao X, Sun J, et al. StyleAvatar: Real-time Photo-realistic Portrait Avatar from a Single Video. In: Proceedings of the Special Interest Group on Computer Graphics and Interactive Techniques Conference Proceedings; 2023. pp. 1-10.
16. Snavely N, Seitz SM, Szeliski R. Photo tourism. ACM Transactions on Graphics. 2006; 25(3): 835-846. doi: 10.1145/1141911.1141964
17. Schönberger JL, Zheng E, Frahm JM, et al. Pixelwise View Selection for Unstructured Multi-View Stereo. In: Proceedings of the European Conference on Computer Vision; 2016. pp. 501–518.
18. Li R, Tanke J, Vo M, et al. TAVA: Template-free Animatable Volumetric ActorsS. In: Proceedings of the European Conference on Computer Vision; 2022. pp. 419–436.
19. Niemeyer M, Mescheder L, Oechsle M, et al. Differentiable Volumetric Rendering: Learning Implicit 3D Representations Without 3D Supervision. In: Proceedings of the 2020 IEEE/CVF Conference on Computer Vision and Pattern Recognition (CVPR); 2020. pp. 3501-3512.
20. Varol G, Ceylan D, Russell B, et al. BodyNet: Volumetric Inference of 3D Human Body Shapes. In: Proceedings of the 2018 IEEE/CVF Conference on Computer Vision and Pattern Recognition (CVPR); 2018. pp. 20–36.
21. Garbin SJ, Kowalski M, Johnson M, et al. FastNeRF: High-Fidelity Neural Rendering at 200FPS. In: Proceedings of the 2021 IEEE/CVF International Conference on Computer Vision (ICCV). 2021; 14326-14335.
22. Chen A, Xu Z, Geiger A, et al. TensoRF: Tensorial Radiance Fields. In: Proceedings of the European Conference on Computer Vision; 2022. pp. 333–350.
23. Fridovich-Keil S, Yu A, Tancik M, et al. Plenoxels: Radiance Fields without Neural Networks. In: Proceedings of the 2022 IEEE/CVF Conference on Computer Vision and Pattern Recognition (CVPR); 2022. pp. 5491-5500.
24. Chen A, Xu Z, Zhao F, et al. MVSNeRF: Fast Generalizable Radiance Field Reconstruction from Multi-View Stereo. In: Proceedings of the 2021 IEEE/CVF International Conference on Computer Vision (ICCV); 2021. pp. 14104-14113.
25. Deng K, Liu A, Zhu JY, et al. Depth-supervised NeRF: Fewer Views and Faster Training for Free. In: Proceedings of the 2022 IEEE/CVF Conference on Computer Vision and Pattern Recognition (CVPR). doi: 10.1109/cvpr52688.2022.01254
26. Park K, Sinha U, Hedman P, et al. HyperNeRF: a higher-dimensional representation for topologically varying neural radiance fields. ACM Transactions on Graphics. 2021; 40(6): 1–12.
27. Park K, Sinha U, Barron JT, et al. Nerfies: Deformable Neural Radiance Fields. In: Proceedings of the 2021 IEEE/CVF International Conference on Computer Vision (ICCV); 2021. pp. 5845-5854.
28. Hu L, Zhang H, Zhang Y, et al. GaussianAvatar: Towards Realistic Human Avatar Modeling from a Single Video via Animatable 3D Gaussians. In: Proceedings of the 2024 IEEE/CVF Conference on Computer Vision and Pattern Recognition (CVPR); 2024. pp. 634-644.
29. Yang Z, Gao X, Zhou W, et al. Deformable 3D Gaussians for High-Fidelity Monocular Dynamic Scene Reconstruction. In: Proceedings of the 2024 IEEE/CVF Conference on Computer Vision and Pattern Recognition (CVPR); pp. 20331-20341.
30. Sun J, Jiao H, Li G, et al. 3DGStream: On-the-Fly Training of 3D Gaussians for Efficient Streaming of Photo-Realistic Free-Viewpoint Videos. In: Proceedings of the 2024 IEEE/CVF Conference on Computer Vision and Pattern Recognition (CVPR); 2024. pp. 20675-20685.
31. Wu G, Yi T, Fang J, et al. 4D Gaussian Splatting for Real-Time Dynamic Scene Rendering. In: Proceedings of the 2024 IEEE/CVF Conference on Computer Vision and Pattern Recognition (CVPR); 2024. pp. 20310-20320.
32. Alldieck T, Pons-Moll G, Theobalt C, et al. Tex2Shape: Detailed Full Human Body Geometry from a Single Image. In: Proceedings of the 2019 IEEE/CVF International Conference on Computer Vision (ICCV); 2019. pp. 2293-2303.
33. Alldieck T, Magnor M, Bhatnagar BL, et al. Learning to Reconstruct People in Clothing from a Single RGB Camera. In: Proceedings of the 2019 IEEE/CVF Conference on Computer Vision and Pattern Recognition (CVPR); 2019. pp. 1175-1186. doi: 10.1109/cvpr.2019.00127
34. Loper M, Mahmood N, Romero J, et al. SMPL: a skinned multi-person linear model. ACM Transactions on Graphics. 2015; 34(6): 1–16.
35. Zheng Z, Yu T, Liu Y, et al. PaMIR: Parametric Model-Conditioned Implicit Representation for Image-Based Human Reconstruction. IEEE Transactions on Pattern Analysis and Machine Intelligence. 2022; 44(6): 3170-3184. doi: 10.1109/tpami.2021.3050505
36. Saito S, Huang Z, Natsume R, et al. PIFu: Pixel-Aligned Implicit Function for High-Resolution Clothed Human Digitization. In: Proceedings of the 2019 IEEE/CVF International Conference on Computer Vision (ICCV); 2019. pp. 2304-2314.
37. Saito S, Simon T, Saragih J, et al. PIFuHD: Multi-Level Pixel-Aligned Implicit Function for High-Resolution 3D Human Digitization. In: Proceedings of the 2020 IEEE/CVF Conference on Computer Vision and Pattern Recognition (CVPR); 2020. pp. 81-90.
38. Jiang B, Zhang J, Hong Y, et al. BCNet: Learning Body and Cloth Shape from a Single Image. In: Proceedings of the European Conference on Computer Vision; 2020. pp. 18–35.
39. Xiu Y, Yang J, Tzionas D, et al. ICON: Implicit Clothed humans Obtained from Normals. In: Proceedings of the 2022 IEEE/CVF Conference on Computer Vision and Pattern Recognition (CVPR); 2022. pp. 13286-13296.
40. Pavlakos G, Choutas V, Ghorbani N, et al. Expressive Body Capture: 3D Hands, Face, and Body from a Single Image. In: Proceedings of the 2019 IEEE/CVF Conference on Computer Vision and Pattern Recognition (CVPR). 2019. pp. 10967-10977.
41. Weng C-Y, Curless B, Kemelmacher-Shlizerman I. Vid2Actor: Free-viewpoint Animatable Person Synthesis from Video in the Wild. arXiv. 2020; arXiv:2012.12884.
42. Weng CY, Srinivasan PP, Curless B, et al. PersonNeRF: Personalized Reconstruction from Photo Collections. In: Proceedings of the 2023 IEEE/CVF Conference on Computer Vision and Pattern Recognition (CVPR); 2023. pp. 524-533.
43. Noguchi A, Sun X, Lin S, et al. Neural Articulated Radiance Field. In: Proceedings of the 2021 IEEE/CVF International Conference on Computer Vision (ICCV); 2021. pp. 5742-5752.
44. Li Z, Zheng Z, Liu Y, et al. PoseVocab: Learning Joint-structured Pose Embeddings for Human Avatar Modeling. In: Proceedings of the Special Interest Group on Computer Graphics and Interactive Techniques Conference Proceedings; 2023. pp. 1-11.
45. Lei J, Wang Y, Pavlakos G, et al. GART: Gaussian Articulated Template Models. In: Proceedings of the 2024 IEEE/CVF Conference on Computer Vision and Pattern Recognition (CVPR); 2024. pp. 19876-19887.
46. Qian Z, Wang S, Mihajlovic M, et al. 3DGS-Avatar: Animatable Avatars via Deformable 3D Gaussian Splatting. In: Proceedings of the 2024 IEEE/CVF Conference on Computer Vision and Pattern Recognition (CVPR); 2024. pp. 5020-5030.
Refbacks
- There are currently no refbacks.
Copyright (c) 2025 Author(s)
License URL: https://creativecommons.org/licenses/by/4.0/
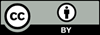
This site is licensed under a Creative Commons Attribution 4.0 International License (CC BY 4.0).
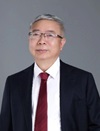
Prof. Zhigeng Pan
Director, Institute for Metaverse, Nanjing University of Information Science & Technology, China
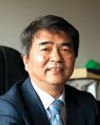
Prof. Jianrong Tan
Academician, Chinese Academy of Engineering, China
Date and Time: April 13, 2025 16:00-17:30pm (Beijing Time)
#VooV Meeting VooV ID: 771-932-597...