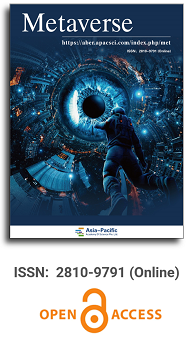
Asia Pacific Academy of Science Pte. Ltd. (APACSCI) specializes in international journal publishing. APACSCI adopts the open access publishing model and provides an important communication bridge for academic groups whose interest fields include engineering, technology, medicine, computer, mathematics, agriculture and forestry, and environment.
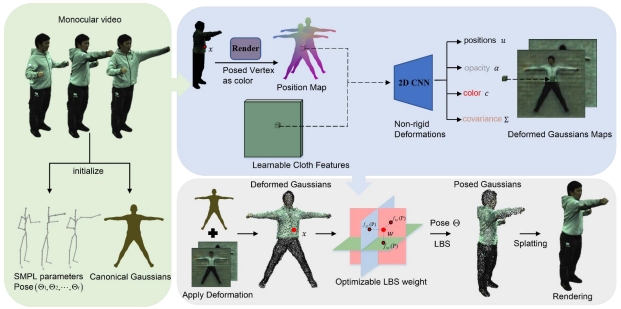
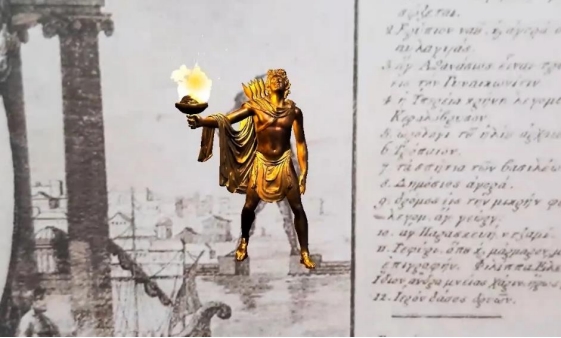
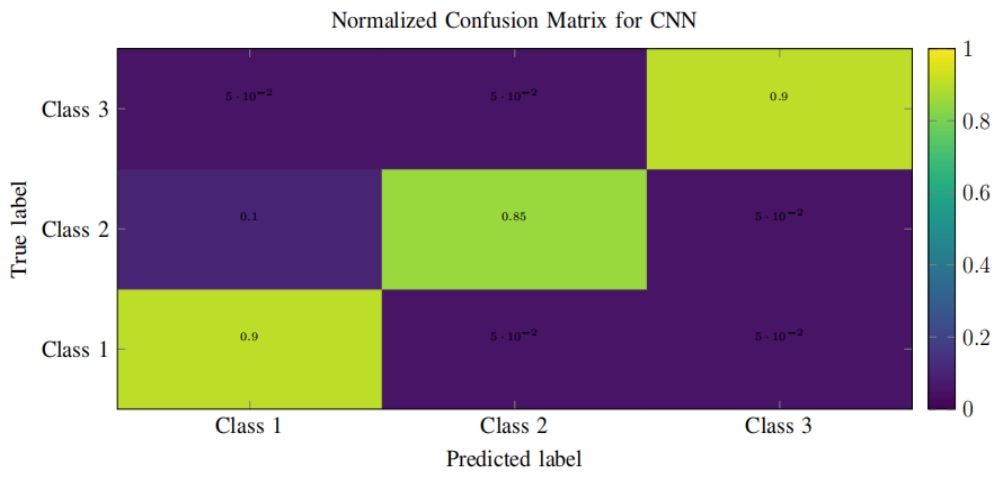
Towards a potential paradigm shift in health data collection and analysis: Contemporary challenges of Human-Machine interaction
Vol 5, Issue 1, 2024
Download PDF
Abstract
Industrial Revolution 4.0 transforms healthcare systems. The first three technological revolutions changed the relationship between human and machine interaction due to the exponential growth of the number of machines. The fourth revolution has placed humans in a scenario where heterogeneous data is generated in unprecedented quantity and quality, not only through traditional methods enhanced by digitization, but also through ubiquitous computing, machine-to-machine interactions, and smart environment. The modern cyber-physical space underlines the role of humans in the expanding context of computerization and big data processing. In healthcare, where data collection and analysis particularly depend on human efforts, the disruptive nature of these developments is evident. Adaptation to this process requires deep scrutiny of the trends and recognition of future medical data technologies` evolution. Significant difficulties arise from discrepancies in requirements by healthcare, administrative and technology stakeholders. Black box and grey box decisions made in medical imaging and diagnostic Decision Support Software are often not transparent enough for the professional, social and medico-legal requirements. While Explainable AI proposes a partial solution for AI applications in medicine, the approach has to be wider and multiplex. LLM potential and limitations are also discussed. This paper lists the most significant issues in these topics and describes possible solutions.
Keywords
References
- Vollset SE, Goren E, Yuan CW, et al. Fertility, mortality, migration, and population scenarios for 195 countries and territories from 2017 to 2100: a forecasting analysis for the Global Burden of Disease Study. The Lancet. 2020; 396(10258): 1285-1306. doi: 10.1016/S0140-6736(20)30677-2
- Lee JY, Lim JY. The Prospect of the Fourth Industrial Revolution and Home Healthcare in Super-Aged Society. Annals of Geriatric Medicine and Research. 2017; 21(3): 95-100. doi: 10.4235/agmr.2017.21.3.95
- Kim G, Lee HK, Lee B, et al. Fourth Industrial Revolution in Japan: Technology to Address Social Challenges. SSRN Electronic Journal. Published online 2021. doi: 10.2139/ssrn.3815376
- Quer G, Radin JM, Gadaleta M, et al. Wearable sensor data and self-reported symptoms for COVID-19 detection. Nature Medicine. 2020; 27(1): 73-77. doi: 10.1038/s41591-020-1123-x
- Dix A. Human-computer interaction. Pearson Education Limited; 2003.
- Shorten C, Khoshgoftaar TM, Furht B. Deep Learning applications for COVID-19. Journal of Big Data. 2021; 8(1). doi: 10.1186/s40537-020-00392-9
- Norman KL, Kirakowski J. The Wiley Handbook of Human Computer Interaction. Published online December 28, 2017. doi: 10.1002/9781118976005
- Ottens J, Baker RA, Newland RF, et al. The Future of the Perfusion Record: Automated Data Collection vs. Manual Recording. The Journal of ExtraCorporeal Technology. 2005; 37(4): 355-359. doi: 10.1051/ject/200537355
- Lee CH, Yoon HJ. Medical big data: promise and challenges. Kidney Research and Clinical Practice. 2017; 36(1): 3-11. doi: 10.23876/j.krcp.2017.36.1.3
- Brown, Philip JB, Yongsheng G, et al. What is the abdomen? Rationalising clinical and anatomical perspectives using formal semantics. Journal of Anatomy. 2021; 238(6): 1472-1491. doi: 10.1111/joa.13384
- Kendler KS. An historical framework for psychiatric nosology. Psychological Medicine. 2009; 39(12): 1935-1941. doi: 10.1017/s0033291709005753
- Kendler KS. Reflections on the Relationship Between Psychiatric Genetics and Psychiatric Nosology. American Journal of Psychiatry. 2006; 163(7): 1138-1146. doi: 10.1176/ajp.2006.163.7.1138
- Rodrigues JM, Schulz S, Mizen B, et al. Is the application of SNOMED CT concept model sufficiently quality assured? AMIA Annual Symposium Proceedings. American Medical Informatics Association. 2018; 16: 1488-1497.
- Sutton RT, Pincock D, Baumgart DC, et al. An overview of clinical decision support systems: benefits, risks, and strategies for success. npj Digital Medicine. 2020; 3(1). doi: 10.1038/s41746-020-0221-y
- Hoffman S, Podgurski A. The Use and Misuse of Biomedical Data: Is Bigger Really Better? American Journal of Law & Medicine. 2013; 39(4): 497-538. doi: 10.1177/009885881303900401
- Dash S, Shakyawar SK, Sharma M, et al. Big data in healthcare: management, analysis and future prospects. Journal of Big Data. 2019; 6(1). doi: 10.1186/s40537-019-0217-0
- Kitchin R, McArdle G. What makes Big Data, Big Data? Exploring the ontological characteristics of 26 datasets. Big Data & Society. 2016; 3(1): 2053951716631130. doi: 10.1177/2053951716631130
- Batko K, Slezak A. The use of Big Data Analytics in healthcare. Journal of Big Data. 2022; 9(1): 3. doi: 10.1186/s40537-021-00553-4
- Caliebe A, Leverkus F, Antes G, et al. Does big data require a methodological change in medical research? BMC Medical Research Methodology. 2019; 19(1). doi: 10.1186/s12874-019-0774-0
- Apgar D. The False Promise of Big Data: Can Data Mining Replace Hypothesis-Driven Learning in the Identification of Predictive Performance Metrics? Systems Research and Behavioral Science. 2013; 32(1): 28-49. doi: 10.1002/sres.2219
- Machado LMO, Souza RR, da Graça Simões M. Semantic web or web of data? a diachronic study (1999 to 2017) of the publications of tim berners‐lee and the world wide web consortium. Journal of the Association for Information Science and Technology. 2019; 70(7): 701-714. doi: 10.1002/asi.24111
- Odigie E, Lacson R, Raja A, et al. Fast Healthcare Interoperability Resources, Clinical Quality Language, and Systematized Nomenclature of Medicine—Clinical Terms in Representing Clinical Evidence Logic Statements for the Use of Imaging Procedures: Descriptive Study. JMIR Medical Informatics. 2019; 7(2): e13590. doi: 10.2196/13590
- Hvolbek AP, Nilsson PM, Sanguedolce F, et al. A prospective study of the effect of video games on robotic surgery skills using the high-fidelity virtual reality RobotiX simulator. Advances in Medical Education and Practice. 2019; 10: 627-634. doi: 10.2147/amep.s199323
- Rosser JC, Gentile DA, Hanigan K, et al. The Effect of Video Game “Warm-up” on Performance of Laparoscopic Surgery Tasks. JSLS: Journal of the Society of Laparoendoscopic Surgeons. 2012; 16(1): 3-9. doi: 10.4293/108680812x13291597715664
- Pasquale F. The Black Box Society. Published online January 5, 2015. doi: 10.4159/harvard.9780674736061
- Pintelas E, Livieris IE, Pintelas P. A Grey-Box Ensemble Model Exploiting Black-Box Accuracy and White-Box Intrinsic Interpretability. Algorithms. 2020; 13(1): 17. doi: 10.3390/a13010017
- Holzinger A, Saranti A, Molnar C, et al. Explainable AI methods-a brief overview. Available online: https://link.springer.com/chapter/10.1007/978-3-031-04083-2_2 (accessed on 7 February 2024).
- Pedreschi D, Giannotti F, Guidotti R, et al. Meaningful Explanations of Black Box AI Decision Systems. Proceedings of the AAAI Conference on Artificial Intelligence. 2019; 33(01): 9780-9784. doi: 10.1609/aaai.v33i01.33019780
- Sebastian B, von Luxburg U. From Shapley values to generalized additive models and back. Available online: https://arxiv.org/abs/2209.04012 (accessed on 6 February 2024).
- Cascella M, Montomoli J, Bellini V, et al. Evaluating the Feasibility of ChatGPT in Healthcare: An Analysis of Multiple Clinical and Research Scenarios. Journal of Medical Systems. 2023; 47(1). doi: 10.1007/s10916-023-01925-4
- Le Q, Lavingia KS, Amendola M. The performance of large language models on fictional consult queries indicates favorable potential for AI-assisted vascular surgery consult handling. JVS-Vascular Insights. 2024; 2: 100052. doi: 10.1016/j.jvsvi.2023.100052
- Li R, Wang X, Yu H. Two Directions for Clinical Data Generation with Large Language Models: Data-to-Label and Label-to-Data. Findings of the Association for Computational Linguistics: EMNLP 2023. Published online 2023. doi: 10.18653/v1/2023.findings-emnlp.474
- Montagna S, Ferretti S, Klopfenstein LC, et al. Data Decentralisation of LLM-Based Chatbot Systems in Chronic Disease Self-Management. In: Proceedings of the 2023 ACM Conference on Information Technology for Social Good; 6 September 2023. doi: 10.1145/3582515.3609536
- Goyal S, Rastogi E, Rajagopal SP, et al. HealAI: A Healthcare LLM for Effective Medical Documentation. In: Proceedings of the 17th ACM International Conference on Web Search and Data Mining; 4 March 2024. doi: 10.1145/3616855.3635739
- Yuan J, Tang R, Jiang X, et al. Large language models for healthcare data augmentation: An example on patient-trial matching. Available online: https://arxiv.org/abs/2303.16756 (accessed on 21 January 2024).
- Panagoulias DP, Virvou M. Tsihrintzis GA. Evaluating LLM--Generated Multimodal Diagnosis from Medical Images and Symptom Analysis. Available online: https://arxiv.org/abs/2402.01730 (accessed on 16 January 2024).
- Eggmann F, Weiger R, Zitzmann NU, et al. Implications of large language models such as ChatGPT for dental medicine. Journal of Esthetic and Restorative Dentistry. 2023; 35(7): 1098-1102. doi: 10.1111/jerd.13046
- Iftikhar L, Iftikhar MF, Hanif MI. Docgpt: Impact of chatgpt-3 on health services as a virtual doctor. EC Paediatrics. 2023; 12(1): 45-55.
- Berenguer A, Morejón A, Tomás D, et al. Using Large Language Models to Enhance the Reusability of Sensor Data. Sensors. 2024; 24(2): 347. doi: 10.3390/s24020347
- Zhang X, Vemulapalli S, Talukdar N, et al. Large Language Models in Medical Term Classification and Unexpected Misalignment Between Response and Reasoning. Available online: https://arxiv.org/abs/2312.14184 (accessed on 16 January 2024).
- Yao JY, Ning KP, Liu ZH, et al. Llm lies: Hallucinations are not bugs, but features as adversarial examples. Available online: https://arxiv.org/abs/2310.01469 (accessed on 6 January 2024).
- Ji Z, Yu T, Xu Y, et al. Towards Mitigating LLM Hallucination via Self Reflection. Findings of the Association for Computational Linguistics: EMNLP 2023. Published online 2023. doi: 10.18653/v1/2023.findings-emnlp.123
Refbacks
- There are currently no refbacks.
Copyright (c) 2024 David J. Herzog, Nitsa J. Herzog
License URL: https://creativecommons.org/licenses/by/4.0/
This site is licensed under a Creative Commons Attribution 4.0 International License (CC BY 4.0).
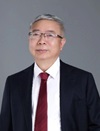
Prof. Zhigeng Pan
Director, Institute for Metaverse, Nanjing University of Information Science & Technology, China
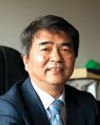
Prof. Jianrong Tan
Academician, Chinese Academy of Engineering, China
Conference Time
December 15-18, 2025
Conference Venue
Hong Kong Convention and Exhibition Center (HKCEC)
...
Metaverse Scientist Forum No.3 was successfully held on April 22, 2025, from 19:00 to 20:30 (Beijing Time)...
We received the Scopus notification on April 19th, confirming that the journal has been successfully indexed by Scopus...
We are pleased to announce that we have updated the requirements for manuscript figures in the submission guidelines. Manuscripts submitted after April 15, 2025 are required to strictly adhere to the change. These updates are aimed at ensuring the highest quality of visual content in our publications and enhancing the overall readability and impact of your research. For more details, please find it in sumissions...