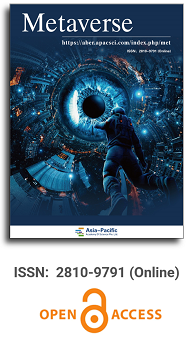
Asia Pacific Academy of Science Pte. Ltd. (APACSCI) specializes in international journal publishing. APACSCI adopts the open access publishing model and provides an important communication bridge for academic groups whose interest fields include engineering, technology, medicine, computer, mathematics, agriculture and forestry, and environment.
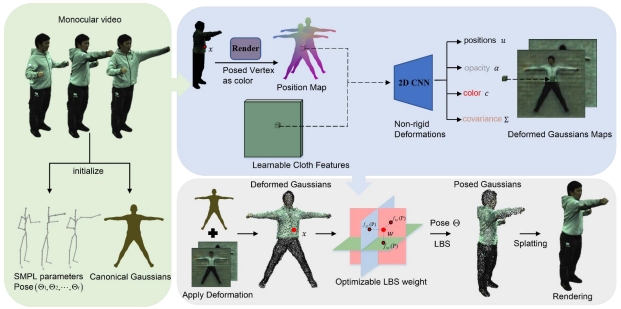
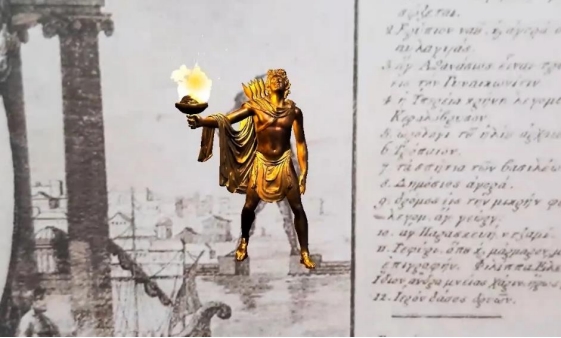
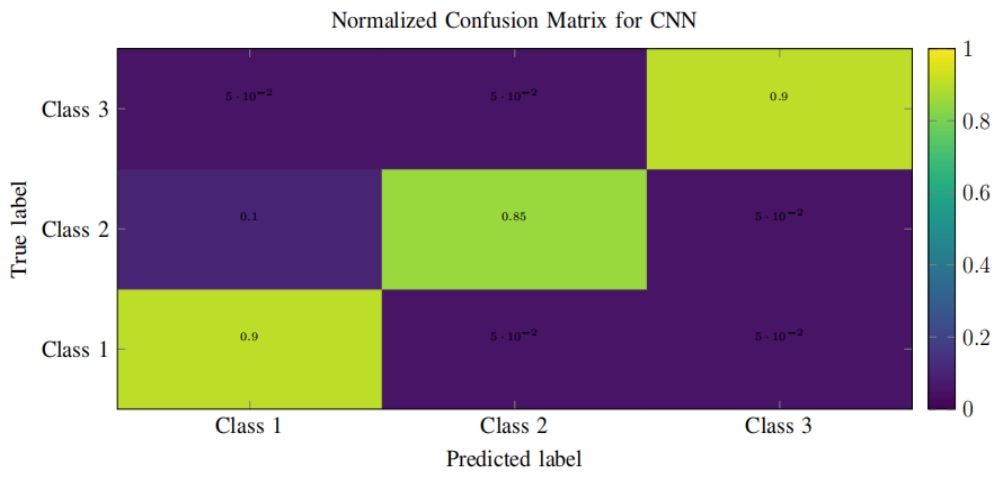
Multi-task super resolution method for vector field critical points enhancement
Vol 3, Issue 1, 2022
Download PDF
Abstract
It is a challenging task to handle the vector field visualization at local critical points. Generally, topological based methods firstly divide critical regions into different categories, and then process the different types of critical regions to improve the effect, which pipeline is complex. In the paper, a learning based multi-task super resolution (SR) method is proposed to improve the refinement of vector field, and enhance the visualization effect, especially at the critical region. In detail, the multi-task model consists of two important designs on task branches: one task is to simulate the interpolation of discrete vector fields based on an improved super-resolution network; and the other is a classification task to identify the types of critical vector fields. It is an efficient end-to-end architecture for both training and inferencing stages, which simplifies the pipeline of critical vector field visualization and improves the visualization effect. In experiment, we compare our method with both traditional interpolation and pure SR network on both simulation data and real data, and the reported results indicate our method lower the error and improve PSNR significantly.
Keywords
References
- Chen W, Shen Z, Tao Y. Big data series: Data visualization. 2nd ed. Beijing: Publishing House of Electronics Industry; 2019.
- Tang B, Shi H. Intelligent vector field visualization based on line integral convolution. Cognitive Systems Research 2018; 52: 828–842.
- Zhang J, Guo H, Hong F, et al. Dynamic load balancing based on constrained k-d tree decomposition for parallel particle tracing. IEEE Transactions on Visualization and Computer Graphics 2018; 24(1): 954–963.
- Engelke W, Lawonn K, PREIM B, et al. Autonomous particles for interactive flow visualization. Computer Graphics Forum 2019; 38(1): 248–259.
- Cabral B, Leedom LC. Imaging vector fields using line integral convolution. Association for Computing Machinery 1993; 6: 263–270.
- Günther T, Rojo I. Introduction to Vector field topology. In: Hotz I, Masood TB, Sadlo F, Tierny J (editors). Topological methods in data analysis and visualization VI. Switzerland: Springer International Publishing; 2021.
- Ba Z, Dan G, Liu J, et al. A feature-based seeding method for multi-level flow visualization. Journal of Computer-Aided Design & Computer Graphics 2016; 28(1): 32–40.
- Hohman F, Kahng M, Pienta R, et al. Visual analytics in deep learning: An interrogative survey for the next frontiers. IEEE Transactions on Visualization and Computer Graphics 2019; 25(8): 2674–2693.
- Yang C, Li Y, Liu C, et al. Deep learning-based viewpoint recommendation in volume visualization. Journal of Visualization 2019; 22(5): 991–1003.
- Han J, Tao J, Zheng H, et al. Flow field reduction via reconstructing vector data from 3-D streamlines using deep learning. IEEE Computer Graphics and Applications 2019; 39(4): 54–67.
- Xia J, Li J, Chen S, et al. A survey on interdisciplinary research of visualization and artificial intelligence. Scientia Sinica (Informationis) 2021; 51: 1777–1801.
- Dong C, Loy C, He K, et al. Learning a deep convolutional network for image super-resolution. European Conference on Computer Vision; 2014 Sep 6. Springer International Publishing; 2014. p. 184–199.
- Dong C, Loy C, Tang X. Accelerating the super-resolution convolutional neural network. arXiv; 2016.
- Kim J, Lee J, Lee K. Accurate image super-resolution using very deep convolutional networks. IEEE Conference on Computer Vision & Pattern Recognition; 2015.
- Shi W, Caballero J, Huszár F, et al. Real-time single image and video super-resolution using an efficient sub-pixel convolutional neural network. arXiv; 2016.
- Zhou Z, Hou Y, Wang Q, et al. Volume upscaling with convolutional neural networks. Proceedings of the Computer Graphics International Conference. New York: Association for Computing Machinery; 2017. p. 1–6.
- Jakob J, Gross M, Gunther T. A fluid flow data set for machine learning and its application to neural flow map interpolation. IEEE Transactions on Visualization and Computer Graphics 2021; 27(2): 1279–1289.
- Guo L, Ye S, Han J, et al. SSR-VFD: Spatial super-resolution for vector field data analysis and visualization. 2020 IEEE Pacific Visualization Symposium (Pacific Vis); 2020.
- Kim B, Günther T. Robust reference frame extraction from unsteady 2D vector fields with convolutional neural networks. Computer Graphics Forum 2019; 38(3): 285–295.
Refbacks
- There are currently no refbacks.
Copyright (c) 2022 Yilun Yang, Zhou Hao, Weilong Peng, Keke Tang, Meie Fang

This work is licensed under a Creative Commons Attribution 4.0 International License.
This site is licensed under a Creative Commons Attribution 4.0 International License (CC BY 4.0).
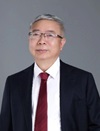
Prof. Zhigeng Pan
Director, Institute for Metaverse, Nanjing University of Information Science & Technology, China
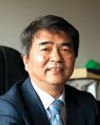
Prof. Jianrong Tan
Academician, Chinese Academy of Engineering, China
Conference Time
December 15-18, 2025
Conference Venue
Hong Kong Convention and Exhibition Center (HKCEC)
...
Metaverse Scientist Forum No.3 was successfully held on April 22, 2025, from 19:00 to 20:30 (Beijing Time)...
We received the Scopus notification on April 19th, confirming that the journal has been successfully indexed by Scopus...
We are pleased to announce that we have updated the requirements for manuscript figures in the submission guidelines. Manuscripts submitted after April 15, 2025 are required to strictly adhere to the change. These updates are aimed at ensuring the highest quality of visual content in our publications and enhancing the overall readability and impact of your research. For more details, please find it in sumissions...