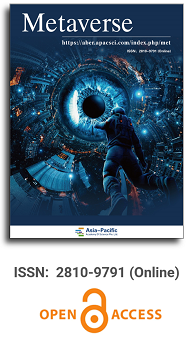
Asia Pacific Academy of Science Pte. Ltd. (APACSCI) specializes in international journal publishing. APACSCI adopts the open access publishing model and provides an important communication bridge for academic groups whose interest fields include engineering, technology, medicine, computer, mathematics, agriculture and forestry, and environment.
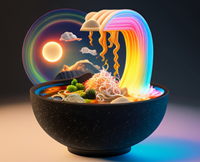
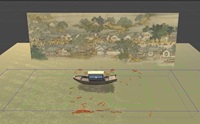
Deciphering avian emotions: A novel AI and machine learning approach to understanding chicken vocalizations
Vol 5, Issue 2, 2024
Download PDF
Abstract
In this groundbreaking study, we present a novel approach to interspecies communication, focusing on the understanding of chicken vocalizations. Leveraging advanced mathematical models in artificial intelligence (AI) and machine learning, we have developed a system capable of interpreting various emotional states in chickens, including hunger, fear, anger, contentment, excitement, and distress. Our methodology employs a cutting-edge AI technique we call Deep Emotional Analysis Learning (DEAL), a highly mathematical and innovative approach that allows for the nuanced understanding of emotional states through auditory data. DEAL is rooted in complex mathematical algorithms, enabling the system to learn and adapt to new vocal patterns over time. We conducted our study with a sample of 80 chickens, meticulously recording and analyzing their vocalizations under various conditions. To ensure the accuracy of our system’s interpretations, we collaborated with a team of eight animal psychologists and veterinary surgeons, who provided expert insights into the emotional states of the chickens. Our system demonstrated an impressive accuracy rate of close to 80%, marking a significant advancement in the field of animal communication. This research not only opens up new avenues for understanding and improving animal welfare but also sets a precedent for further studies in AI-driven interspecies communication. The novelty of our approach lies in its application of sophisticated AI techniques to a largely unexplored area of study. By bridging the gap between human and animal communication, we believe our research will pave the way for more empathetic and effective interactions with the animal kingdom.
Keywords
References
1. Hauser MD, Konishi M. The design of animal communication. MIT press; 1999.
2. Soltis J, Leong K, Savage A. African elephant vocal communication ii: rumble variation reflects the individual identity and emotional state of callers. Animal Behaviour. 2005; 70(3): 589–599.
3. Marino L, Colvin CM. Thinking chickens: a review of cognition, emotion, and behavior in the domestic chicken. Animal Cognition. 2017; 20(2): 127–147, 2017.
4. Russell AJ. Artificial intelligence a modern approach. Pearson Education, Inc.; 2010.
5. LeCun Y, Bengio Y, Hinton G. Deep learning. Nature. 2015; 521(7553): 436–444.
6. Odom KJ, Araya-Salas M, Morano JL, et al. Comparative bioacoustics: a roadmap for quantifying and comparing animal sounds across diverse taxa. Biological Reviews. 2021; 96(4): 1135–1159.
7. Quinlan JR. Induction of decision trees. Machine Learning. 1986; 1(1): 81–106.
8. Fong T, Nourbakhsh I, Dautenhahn K. A survey of socially interactive robots. Robotics and Autonomous Systems. 2003; 42(3–4): 143–166.
9. Morley J, Floridi L, Kinsey L, Elhalal A. From what to how: An initial review of publicly available ai ethics tools, methods and research to translate principles into practices. Science and Engineering Ethics. 2020; 26: 2141–2168.
10. Wittemyer G, Northrup JM, Bastille-Rousseau G. Behavioural valuation of landscapes using movement data. Philosophical Transactions of the Royal Society B. 2019; 374(1781): 20180046.
11. Bas Y, Bas D, Julien JF. Tadarida: A toolbox for animal detection on acoustic recordings. Journal of open Research Software. 2017; 5(1).
12. Stowell D, Wood M, Pamuła H, et al. Automatic acoustic detection of birds through deep learning: The first bird audio detection challenge. Methods in Ecology and Evolution. 2019; 10(3): 368–380.
13. Bermant PC, Bronstein MM, Wood RJ, et al. Deep machine learning techniques for the detection and classification of sperm whale bioacoustics. Scientific Reports. 2019; 9(1): 12588.
14. Qian K, Zhang Z, Ringeval F, Schuller B. Bird sounds classification by large scale acoustic features and extreme learning machine. In: Proceedings of the 2015 IEEE Global Conference on Signal and Information Processing (GlobalSIP); 14–16 December 2015; Pistacaway NJ, USA. pp. 1317–1321.
15. Hershey S, Chaudhuri S, Ellis DP, et al. Cnn architectures for large-scale audio classification. In: Proceedings of the 2017 ieee international conference on acoustics, speech and signal processing (icassp); 5–9 March 2017; New Orleans, LA, USA. pp. 131–135.
Refbacks
- There are currently no refbacks.
Copyright (c) 2024 Adrian David Cheok, Jun Cai, Ying Yan
License URL: https://creativecommons.org/licenses/by/4.0/
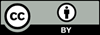
This site is licensed under a Creative Commons Attribution 4.0 International License (CC BY 4.0).
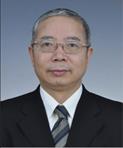
Prof. Zhigeng Pan
Director, Institute for Metaverse, Nanjing University of Information Science & Technology, China
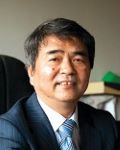
Prof. Jianrong Tan
Academician, Chinese Academy of Engineering, China