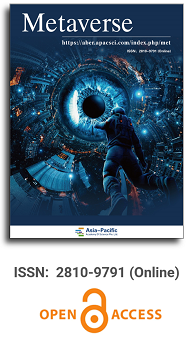
Asia Pacific Academy of Science Pte. Ltd. (APACSCI) specializes in international journal publishing. APACSCI adopts the open access publishing model and provides an important communication bridge for academic groups whose interest fields include engineering, technology, medicine, computer, mathematics, agriculture and forestry, and environment.
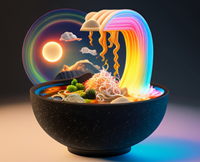
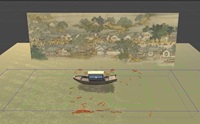
Automatic labeling of 3D facial acupoint landmarks
Vol 5, Issue 1, 2024
VIEWS - 3253 (Abstract)
Download PDF
Abstract
As special marks on a human face, facial landmarks reflect the facial features of various parts of the face, which is crucial in biomedicine and medical imaging. In addition, facial landmarks are also important features in computer vision such as face detection, face recognition, facial pose estimation, and facial animation. In this paper, we construct a 3D facial acupoint annotated dataset by labeling 37 facial acupoints on 846 neutral face triangle mesh on the FaceScape dataset. Based on these annotated data, we use a feature template matching method to realize the automatic annotation of 37 acupoints on triangle meshes. We used 40 meshes as the training set to extract the geometric patterns of 3D acupoints and then measured the performance of the automatic labeling algorithm on 20 meshes and 806 meshes as the test sets. In the training process, we extract the tangent plane for each landmark, project the neighbor vertices of the landmark to the tangent plane, and construct the feature image with R × R resolution through the bounding box of the projected points. In the testing process, we use the pattern images extracted during training to find the average features and use them as a guide to optimize the predicted landmarks. The experimental results show that our automatic acupoint labeling method has achieved good results.
Keywords
References
1. Vrochidou E, Papić V, Kalampokas T, Papakostas GA. Automatic Facial Palsy Detection—From Mathematical Modeling to Deep Learning. Axioms. 2023; 12(12): 1091. doi: 10.3390/axioms12121091
2. Kim S, An H, Song M, Lee S, et al. Automated Marker-Less Patient-to-Preoperative Medical Image Registration Approach Using RGB-D Images and Facial Landmarks for Potential Use in Computed-Aided Surgical Navigation of the Paranasal Sinus. In: Advances in Computer Graphics. Cham: Springer Nature Switzerland; 2024. pp. 135–145.
3. Mercan E, Atmosukarto I, Wu J, et al. Craniofacial Image Analysis. In: Health Monitoring and Personalized Feedback using Multimedia Data. Cham: Springer International Publishing; 2015. pp. 9–29.
4. Liu F, Zhao Q, Liu X, Zeng D. Joint Face Alignment and 3D Face Reconstruction with Application to Face Recognition. IEEE Transactions on Pattern Analysis and Machine Intelligence. 2020; 42(3): 664-678. doi: 10.1109/tpami.2018.2885995
5. Sharma U, Faisal KN, Sharma RR, Arya KV. Facial Landmark-Based Human Emotion Recognition Technique for Oriented Viewpoints in the Presence of Facial Attributes. SN Computer Science. 2023; 4(3). doi: 10.1007/s42979-023-01727-y
6. Gupta A, Thakkar K, Gandhi V, Narayanan PJ. Nose, eyes and ears: Head pose estimation by locating facial keypoints. arXiv. 2018.
7. Cootes TF, Taylor CJ, Cooper DH, Graham J. Active Shape Models-Their Training and Application. Computer Vision and Image Understanding. 1995; 61(1): 38-59. doi: 10.1006/cviu.1995.1004
8. Cootes TF, Edwards GJ, Taylor CJ. Active appearance models. IEEE Transactions on Pattern Analysis and Machine Intelligence. 2001; 23(6): 681-685. doi: 10.1109/34.927467
9. Yang H, Zhu H, Wang Y, et al. FaceScape: A Large-Scale High Quality 3D Face Dataset and Detailed Riggable 3D Face Prediction. 2020 IEEE/CVF Conference on Computer Vision and Pattern Recognition (CVPR). Published online June 2020. doi: 10.1109/cvpr42600.2020.00068
10. Farkas LG. Anthropometry of the head and face, 2nd ed. Available online: https://api.semanticscholar.org/CorpusID:57441646 (accessed on 23 March 2023).
11. Qiao H, Tan J, Wen S, et al. De Novo Dissecting the Three-Dimensional Facial Morphology of 2379 Han Chinese Individuals. Phenomics. Published online June 8, 2023. doi: 10.1007/s43657-023-00109-x
12. Liang Y, Liu H, Gao Z, et al. Ocular phenotype related SNP analysis in Southern Han Chinese population from Guangdong province. Gene. 2022; 826: 146458. doi: 10.1016/j.gene.2022.146458
13. Ercan I, Ozdemir ST, Etoz A, et al. Facial asymmetry in young healthy subjects evaluated by statistical shape analysis. Journal of Anatomy. 2008; 213(6): 663-669. doi: 10.1111/j.1469-7580.2008.01002.x
14. Sforza C, Rosati R, De Menezes M, et al. Three-Dimensional Computerized Anthropometry of the Nose. In: Handbook of Anthropometry. New York, NY: Springer New York; 2012. pp. 927–942.
15. Agbolade O, Nazri A, Yaakob R, et al. Morphometric approach to 3D soft-tissue craniofacial analysis and classification of ethnicity, sex, and age. Wilkinson C, ed. PLOS ONE. 2020; 15(4): e0228402. doi: 10.1371/journal.pone.0228402
16. Deutsch CK, Shell AR, Francis RW, Bird BD. The Farkas System of Craniofacial Anthropometry: Methodology and Normative Databases. In: Handbook of Anthropometry. New York, NY: Springer New York; 2012. pp. 561–573.
17. Zhuang W, Chen L, Hong C, et al. FT-GAN: Face Transformation with Key Points Alignment for Pose-Invariant Face Recognition. Electronics. 2019; 8(7): 807. doi: 10.3390/electronics8070807
18. Wang X, Wang Y, Li W, et al. Facial Expression Animation by Landmark Guided Residual Module. IEEE Transactions on Affective Computing. 2023; 14(2): 878-894. doi: 10.1109/taffc.2021.3100352
19. Otberdout N, Daoudi M, Kacem A, et al. Dynamic Facial Expression Generation on Hilbert Hypersphere With Conditional Wasserstein Generative Adversarial Nets. IEEE Transactions on Pattern Analysis and Machine Intelligence. 2022; 44(2): 848-863. doi: 10.1109/tpami.2020.3002500
20. Kostinger M, Wohlhart P, Roth PM, Bischof H. Annotated Facial Landmarks in the Wild: A large-scale, real-world database for facial landmark localization. In: 2011 IEEE International Conference on Computer Vision Workshops (ICCV Workshops), Barcelona, Spain, Nov. 2011, pp. 2144–2151. doi: 10.1109/ICCVW.2011.6130513
21. Sagonas C, Antonakos E, Tzimiropoulos G, et al. 300 Faces In-The-Wild Challenge: database and results. Image and Vision Computing. 2016; 47: 3-18. doi: 10.1016/j.imavis.2016.01.002
22. Zhu X,Ramanan D. Face detection, pose estimation, and landmark localization in the wild. In: 2012 IEEE Conference on Computer Vision and Pattern Recognition, Providence, RI, Jun. 2012, pp. 2879–2886. doi: 10.1109/CVPR.2012.6248014
23. Le V, Brandt J, Lin Z, et al. Interactive Facial Feature Localization. In Computer Vision—ECCV 2012. Berlin, Heidelberg: Springer Berlin Heidelberg; 2012. pp. 679–692. doi: 10.1007/978-3-642-33712-3_49
24. Deng J, Roussos A, Chrysos G, et al. The Menpo Benchmark for Multi-pose 2D and 3D Facial Landmark Localisation and Tracking. International Journal of Computer Vision. 2018; 127(6-7): 599-624. doi: 10.1007/s11263-018-1134-y
25. Zhu X, Lei Z, Liu X, et al. Face Alignment Across Large Poses: A 3D Solution. 2016 IEEE Conference on Computer Vision and Pattern Recognition (CVPR). Published online June 2016. doi: 10.1109/cvpr.2016.23
26. Wu Y, Ji Q. Facial Landmark Detection: A Literature Survey. International Journal of Computer Vision. 2018; 127(2): 115-142. doi: 10.1007/s11263-018-1097-z
27. Baker S, Matthews I. Lucas-Kanade 20 Years On: A Unifying Framework. Int. J. Comput. Vis. 2004; 56(3): 221–255. doi: 10.1023/B:VISI.0000011205.11775.fd
28. Matthews I, Baker S. Active Appearance Models Revisited. Int. J. Comput. Vis. 2004; 60(2): 135–164. doi: 10.1023/B:VISI.0000029666.37597.d3
29. Gross R, Matthews I, Baker S. Appearance-based face recognition and light-fields. IEEE Transactions on Pattern Analysis and Machine Intelligence. 2004; 26(4): 449-465. doi: 10.1109/tpami.2004.1265861
30. Gross R, Matthews I, Baker S. Generic vs. person specific active appearance models. Image and Vision Computing. 2005; 23(12): 1080-1093. doi: 10.1016/j.imavis.2005.07.009
31. Cristinacce D, Cootes TF. Feature Detection and Tracking with Constrained Local Models. In: Proceedings of the British Machine Vision Conference 2006, Edinburgh, 2006, pp. 95.1-95.10. doi: 10.5244/C.20.95
32. Dantone M, Gall J, Fanelli G, Van Gool L. Real-time facial feature detection using conditional regression forests. In: 2012 IEEE Conference on Computer Vision and Pattern Recognition, Providence, RI, Jun. 2012, pp. 2578–2585. doi: 10.1109/CVPR.2012.6247976
33. Zhang J, Hu H, Feng S. Robust Facial Landmark Detection via Heatmap-Offset Regression. IEEE Transactions on Image Processing. 2020; 29: 5050-5064. doi: 10.1109/tip.2020.2976765
34. Jeong M, Ko BC, Kwak S, Nam JY. Driver Facial Landmark Detection in Real Driving Situations. IEEE Transactions on Circuits and Systems for Video Technology. 2018; 28(10): 2753-2767. doi: 10.1109/tcsvt.2017.2769096
35. Deng Z, Liu H. Geometry-attentive relational reasoning for robust facial landmark detection. Neurocomputing. 2021; 453: 790-800. doi: 10.1016/j.neucom.2020.06.126
36. Wu Y, Ji Q. Discriminative Deep Face Shape Model for Facial Point Detection. International Journal of Computer Vision. 2014; 113(1): 37-53. doi: 10.1007/s11263-014-0775-8
37. Salazar A, Wuhrer S, Shu C, Prieto F. Fully automatic expression-invariant face correspondence. Machine Vision and Applications. 2013; 25(4): 859-879. doi: 10.1007/s00138-013-0579-9
38. Papazov C, Marks TK, Jones M. Real-time 3D head pose and facial landmark estimation from depth images using triangular surface patch features. In: 2015 IEEE Conference on Computer Vision and Pattern Recognition (CVPR), Boston, MA, USA, Jun. 2015, pp. 4722–4730. doi: 10.1109/CVPR.2015.7299104
39. Berends B, Bielevelt F, Schreurs R, et al. Fully automated landmarking and facial segmentation on 3D photographs. arXiv. 2023.
40. Perakis P, Passalis G, Theoharis T, Kakadiaris IA. 3D Facial Landmark Detection under Large Yaw and Expression Variations. IEEE Transactions on Pattern Analysis and Machine Intelligence. 2013; 35(7): 1552-1564. doi: 10.1109/tpami.2012.247
41. Wang J, Fang S, Fang M, et al. Automatic Landmark Placement for Large 3D Facial Image Dataset. In: 2019 IEEE International Conference on Big Data (Big Data), Los Angeles, CA, USA, Dec. 2019, pp. 5088–5093. doi: 10.1109/BigData47090.2019.9006310
42. Wang Z, Simoncelli EP, Bovik AC. Multiscale structural similarity for image quality assessment. In: The Thrity-Seventh Asilomar Conference on Signals, Systems & Computers, 2003, Pacific Grove, CA, USA, 2003, pp. 1398–1402. doi: 10.1109/ACSSC.2003.1292216
43. Zhou QY, Park J, Koltun V. Open3D: A Modern Library for 3D Data Processing. arXiv. 2018.
44. Ingale AK, Leema AA, Kim H, Udayan JD. Automatic 3D Facial Landmark-Based Deformation Transfer on Facial Variants for Blendshape Generation. Arabian Journal for Science and Engineering. 2022; 48(8): 10109-10123. doi: 10.1007/s13369-022-07403-2
Refbacks
- There are currently no refbacks.
Copyright (c) 2024 Junjie Yin, Meie Fang, Weiyin Ma
License URL: https://creativecommons.org/licenses/by/4.0/
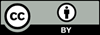
This site is licensed under a Creative Commons Attribution 4.0 International License (CC BY 4.0).
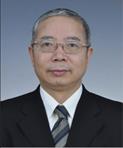
Prof. Zhigeng Pan
Director, Institute for Metaverse, Nanjing University of Information Science & Technology, China
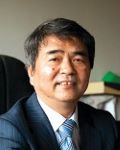
Prof. Jianrong Tan
Academician, Chinese Academy of Engineering, China