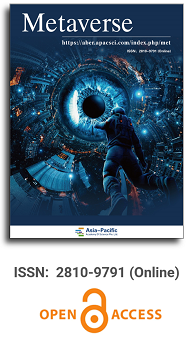
Asia Pacific Academy of Science Pte. Ltd. (APACSCI) specializes in international journal publishing. APACSCI adopts the open access publishing model and provides an important communication bridge for academic groups whose interest fields include engineering, technology, medicine, computer, mathematics, agriculture and forestry, and environment.
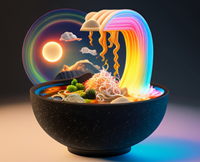
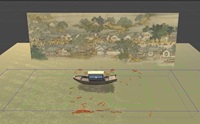
Opportunities for the digital transformation of the banana sector supply chain based on software with artificial intelligence
Vol 2, Issue 1, 2021
Download PDF
Abstract
Artificial intelligence offers great opportunities for the supply chain, being this a competitive advantage for today’s changing market. This article aims to identify the impacts and opportunities that artificial intelligence software can offer to facilitate the operation and improve the performance of the supply chain in the banana sector in Colombia. The work methodology consists of six steps in which a total of 72 investigations were obtained. The sources of information were four databases. As a main conclusion, the supply chain of the banana sector has everything necessary for intelligent software based solutions to be implemented in order to achieve adaptation, flexibility and sensitivity to the context and domain of execution.
Keywords
References
1. Dellino G, Laudadio T, Mari R, et al. A reliable decision support system for fresh food supply chain management. International Journal of Production Research 2018; 56(4): 1458–1485.
2. Muñoz Pinzón DS, Polo Roa A, Sierra Mantilla EJ, et al. Mathematical modeling in agrochain studies: A literature review. Revista Politécnica 2020; 16(31): 110–137.
3. Nasiri M, Ukko J, Saunila M, et al. Managing the digital supply chain: The role of smart technologies. Technovation 2020; 15: 102–121.
4. Wu L, Yue X, Jin A, et al. Smart supply chain management: A review and implications for future research. The International Journal of Logistics Management 2016; 27(2): 395–417.
5. Zhao J, Ji M, Feng B. Smarter supply chain: A literature review and practices. Journal of Data, Information and Management 2020; 3: 1–16.
6. Büyüközkan G, Göçer F. Digital supply chain: Literature review and a proposed framework for future research. Computers in Industry 2018; 97: 157–177.
7. Ehie I, Ferreira L. Conceptual development of supply chain digitalization framework. IFAC-Papers on Line 2019; 52(13): 2338–2342.
8. Min Agriculture. Banana chain indicators and instruments 2018 [Internet]. 2018. Available from: https://www.minagricultura.gov.co/paginas/default.aspx
9. Plinere D, Merkurvev Y. Designing a multi-agent system for improving supply chain performance. 2019 IEEE 7th IEEE Workshop on Advances in Information, Electronic and Electrical Engineering (AIEEE); 2019 Nov 15-16; Liepaja, Latvia. New York: Institute of Electrical and Electronics Engineers (IEEE); 2019. p. 1–7.
10. Shalev Shwartz S, Ben David S. Understanding machine learning: From theory to algorithms. Cambridge: Cambridge University Press; 2014.
11. Wooldridge M. An introduction to multiagent systems. Hoboken, New Jersey: John Wiley & Sons; 2009.
12. Korpela K, Hallikas J, Dahlberg T. Digital supply chain transformation toward blockchain integration. In proceedings of the 50th Hawaii international conference on system sciences. 2017 [cited 2017 Jan]. Available from: http://hdl.handle.net/10125/41666
13. Kamble SS, Gunasekaran A, Gawankar SA. Achieving sustainable performance in a data-driven agriculture supply chain: A review for research and applications. International Journal of Production Economics 2020; 219: 179–194.
14. Lezoche M, Hernandez JE, Diaz M, et al. Agri-food 4.0: A survey of the supply chains and technologies for the future agriculture. Computers in Industry 2020; 117: 103–127.
15. Yin L. Enterprise logistics cost research based on optimized supply chain model. In 2015 6th International Conference on Intelligent Systems Design and Engineering Applications (ISDEA); 2015 Aug 18-19; Washington D.C. New York: IEEE; 2015. p. 380–383.
16. Zhang Y, Liu S, Zhang, X. An optimized supply chain network model based on modified genetic algorithm. Chinese Journal of Electronics 2017; 26(3): 468–476.
17. Yang H, Chung JK, Chen Y, et al. Ordering strategy analysis of prefabricated component manufacturer in construction supply chain. Mathematical Problems in Engineering 2018; 10: 2871–2887.
18. Zhang Y, Jiang Y, Zhong M, et al. Robust optimization on regional WCO-for-Biodiesel supply chain under supply and demand uncertainties. Scientific Programming 2016; 63: 984–996.
19. Akinade OO, Oyedele LO. Integrating construction supply chains within a circular economy: An ANFIS-based waste analytics system (A-WAS). Journal of Cleaner Production 2019; 229: 863–873.
20. Guo F, Liu Q, Liu D, et al. On production and green transportation coordination in a sustainable global supply chain. Sustainability 2017; 9(11): 20–35.
21. Kusolpuchong S, Chusap K, Alhawari O, et al. A genetic algorithm approach for multi objective cross dock scheduling in supply chains. Procedia Manufacturing 2019; 39: 1139–1148.
22. Bank M, Mazdeh M, Heydari M. Applying meta-heuristic algorithms for an integrated production-distribution problem in a two-level supply chain. Uncertain Supply Chain Management 2020; 8(1): 77–92.
23. Hamontree C, Prompakdee S, Koiwanit J. Resource scheduling problem in distribution center. IOP Conference Series: Materials Science and Engineering 2019; 639(1): 12–17.
24. Ahmadizar F, Zeynivand M, Arkat J. Two-level vehicle routing with cross-docking in a three-echelon supply chain: A genetic algorithm approach. Applied Mathematical Modelling 2015; 39(22): 7065–7081.
25. Boru A, Dosdoğru AT, Göçken M, et al. A novel hybrid artificial intelligence based methodology for the inventory routing problem. Symmetry 2019; 11(5): 7–17.
26. Rabbani M, Navazi F, Farrokhi-Asl H, et al. A sustainable transportation-location-routing problem with soft time windows for distribution systems. Uncertain Supply Chain Management 2018; 6(3): 229–254.
27. Moncayo Martinez LA. Supply chain design using a modified IWD algorithm. Revista Facultad de Ingeniería Universidad de Antioquia 2017; (84): 9–16.
28. Gong G, Deng Q, Gong X, et al. A bee evolutionary algorithm for multi objective vehicle routing problem with simultaneous pickup and delivery. Mathematical Problems in Engineering 2018; 2018.
29. Rahman A, Shahruddin NS, Ishak I. Solving the goods transportation problem using genetic algorithm with nearest-node pairing crossover operator. Journal of Physics: Conference Series 2019; 1366(1): 120–137.
30. Fitriana R, Moengin P, Kusumaningrum U. Improvement route for distribution solutions mdvrp (multi depot vehicle routing problem) using genetic algorithm. IOP Conference Series: Materials Science and Engineering 2019; 528(1): 12–42.
31. Frazzon EM, Albrecht A, Pires M, et al. Hybrid approach for the integrated scheduling of production and transport processes along supply chains. International Journal of Production Research 2018; 56(5): 2019–2035.
32. Zhou L, Wang X, Ni L, et al. Location-routing problem with simultaneous home delivery and customer’s pickup for city distribution of online shopping purchases. Sustainability 2019; 8(8): 828–839.
33. Gooran A, Rafiei H, Rabani M. Modeling risk and uncertainty in designing reverse logistics problem. Decision Science Letters 2018; 7(1): 13–24.
34. Soleimani H, Kannan G. A hybrid particle swarm optimization and genetic algorithm for closed-loop supply chain network design in large-scale networks. Applied Mathematical Modelling 2015; 39(14): 3990–4012.
35. Zhou Y, Chan CK, Wong KH, et al. Intelligent optimization algorithms: A stochastic closed-loop supply chain network problem involving oligopolistic competition for multi-products and their product flow routings. Mathematical Problems in Engineering 2015.
36. Afrouzy ZA, Paydar MM, Nasseri SH, et al. A meta-heuristic approach supported by NSGA-II for the design and plan of supply chain networks considering new product development. Journal of Industrial Engineering International 2018; 14(1): 95–109.
37. Yun Y, Chuluunsukh A, Gen M. Sustainable closed-loop supply chain design problem: A hybrid genetic algorithm approach. Mathematics 2020; 8(1): 84–98.
38. Huang RH, Yu TH, Lee CY. Rolling supply chain scheduling considering suppliers, production, and delivery lot-size. Mathematical Problems in Engineering 2018.
39. Huang M, Yi P, Guo L, et al. A modal interval based genetic algorithm for closed-loop supply chain network design under uncertainty. IFAC-Papers on Line 2016; 49(12): 616–621.
40. Mohammadi M, Tavakkoli-Moghaddam R, Siadat A, et al. Design of a reliable logistics network with hub disruption under uncertainty. Applied Mathematical Modelling 2016; 40(9–10): 5621–5642.
41. Kumar RS, Choudhary A, Babu SAK, et al. Designing multi-period supply chain network considering risk and emission: A multi-objective approach. Annals of Operations Research 2017; 250(2): 427–461.
42. Xu Y, Liu X. A new genetic type method with integrated gradient-based algorithm method for storage optimization of supply chain. 2015 International Conference on Computational Intelligence and Communication Networks (CICN); 2015 Dec 12-14; Jabalpur, India. New York: IEEE; 2015. p. 724–729.
43. Dabibi M, Moghaddam B, Kazemi M. Locating distribution/service centers based on multi objective decision-making using set covering and proximity to stock market. International Journal of Industrial Engineering Computations 2016; 7(4): 635–648.
44. Wang Y, Yuan Y, Assogba K, et al. Design and profit allocation in two-echelon heterogeneous cooperative logistics network optimization. Journal of Advanced Transportation 2018; (2): 513–533.
45. Saif-Eddine AS, El-Beheiry MM, El-Kharbotly AK. An improved genetic algorithm for optimizing total supply chain cost in inventory location routing problem. Ain Shams Engineering Journal 2019; 10(1): 63–76.
46. Wang Y, Geng X, Zhang F, et al. An immune genetic algorithm for multi-echelon inventory cost control of IOT based supply chains. IEEE Access 2018; 6: 8547–8555.
47. Jahani H, Alavifard F, Ivanov D, et al. Managing the risk of supply chain bankruptcy in supply chain network redesign. IFAC-PapersOnLine 2019; 52(13): 2431–2436.
48. Shen L, Li F, Li C, et al. Inventory optimization of fresh agricultural products supply chain based on agricultural superdocking. Journal of Advanced Transportation 2020.
49. Simić D, Svirčević V, Simić S. A hybrid evolutionary model for supplier assessment and selection in inbound logistics. Journal of Applied Logic 2015; 13(2): 138–147.
50. Mahmud S, Rahman M, Hasan M, et al. Minimizing the bullwhip effect in a single product multistage supply chain using genetic algorithm. Uncertain Supply Chain Management 2016; 4(2): 137–146.
51. Nakhjirkan S, Mokhatab Rafiei F. An integrated multi-echelon supply chain network design considering stochastic demand: A genetic algorithm-based solution. Promet-Traffic & Transportation 2017; 29(4): 391–400.
52. Jing Y, Li W. Integrated recycling-integrated production-distribution planning for decentralized closed-loop supply chain. Journal of Industrial & Management Optimization 2018; 14(2): 511–539.
53. Agrawal AK, Yadav S. Price and profit structuring for single manufacturer multi-buyer integrated inventory supply chain under price-sensitive demand condition. Computers & Industrial Engineering 2020; 139: 2113–2127.
54. Gonçalo TE, Morais DC. Agent-based negotiation protocol for selecting transportation providers in a retail company. 2015 IEEE International Conference on Systems, Man, and Cybernetics; 2015 Oct 9-12; Hong Kong. New York: IEEE; 2015. p. 263–267.
55. Hsieh FS. Scheduling sustainable supply chains based on multi-agent systems and workflow models. 2015 10th International Conference on Intelligent Systems and Knowledge Engineering (ISKE); 2015 Nov 24-27; Taiwan. New York: IEEE; 2015. p. 252–259.
56. Saoud A, Bellabdaoui A. Model of distributed hierarchical framework for carrier collaboration. 2017 International Colloquium on Logistics and Supply Chain Management (LOGISTIQUA); 2017 Apr 26-27; Rabat. NewYork: IEEE; 2017. p. 160–165.
57. Fu D, Zhang HT, Dutta A, et al. A cooperative distributed model predictive control approach to supply chain management. IEEE Transactions on Systems, Man, and Cybernetics: Systems 2019.
58. Fang D, Pu W. Simulating the structural evolution in agri-food supply chain: An agent-based model. 2015 7th International Conference on Intelligent Human-Machine Systems and Cybernetics; 2015 August 26-27; Hangzhou. New York: IEEE; 2015. p. 214–219.
59. Du J, Sugumaran V, Gao B. RFID and multi-agent-based architecture for information sharing in prefabricated component supply chain. IEEE Access 2017; 5: 4132–4139.
60. Slimani I, Farissi I, Achchab S. Artificial neural networks for demand forecasting: Application using Moroccan supermarket data. 2015 15th International Conference on Intelligent Systems Design and Applications (ISDA); 2015 Dec 14-16; Marrakech, Morocco. New York: IEEE; 2015. p. 266–271.
61. Bousqaoui H, Achchab S, Tikito K. Machine learning applications in supply chains: An emphasis on neural network applications. 2017 3rd International Conference of Cloud Computing Technologies and Applications (CloudTech); 2017 Oct 24-26; Rabat. New York: IEEE; 2017. p. 1–7.
62. Li Z, Li G, Zhang Y, et al. Risk early warning model for distribution network material supply chain of electric power enterprises. 2019 12th International Conference on Intelligent Computation Technology and Automation (ICICTA); 2019 Oct 26-27; Xiangtian, Hunan. New York: IEEE; 2019. p. 700–707.
63. Yang Z, Zhong C, Quan M, et al. Research on supplier selection method based on bp neural network. 2019 IEEE 1st International Conference on Civil Aviation Safety and Information Technology (ICCASIT); 2019 Oct 17-19; Kunming, China. New York: IEEE; 2019. p. 344–347.
64. Lin T, Chuang H, Yu F. Tracking supply chain process variability with unsupervised cluster traversal. 2018 IEEE 16th Intl Conf on Dependable, Autonomic and Secure Computing, 16th Intl Conf on Pervasive Intelligence and Computing, 4th Intl Conf on Big Data Intelligence and Computing and Cyber Science and Technology Congress; 2018 Aug 12-15; Orlando. New York: IEEE; 2018. p. 966–973.
65. He X, Zhang C, Jing Y, et al. Risk evaluation of agricultural product supply chain based on BP neural network. 2019 16th International Conference on Service Systems and Service Management (ICSSSM); 2019 July 13-15; Shenzhen, China. New York: IEEE; 2019. p. 1–8.
66. Cheng Y, Peng J, Gu X, et al. An intelligent supplier evaluation model based on data-driven support vector regression in global supply chain. Computers & Industrial Engineering 2020; 139: 105–134.
67. Liu P, Yi S. New algorithm for evaluating the green supply chain performance in an uncertain environment. Sustainability 2016; 8(10): 960–975.
68. Pereira MM, Frazzon EM. Towards a predictive approach for omni-channel retailing supply chains. IFAC-PapersOnLine 2019; 52(13): 844–850.
69. Kara ME, Fırat SÜO, Ghadge A. A data mining-based framework for supply chain risk management. Computers & Industrial Engineering 2018; 25: 1055–1070.
70. Besheli SF, Keshteli RN, Emami S, et al. A fuzzy dynamic multi-objective multi-item model by considering customer satisfaction in a supply chain. Scientia Iranica. Transaction E, Industrial Engineering 2017; 24(5): 2623–2639.
71. Ammar OB, Marian H, Dolgui A. Supply planning for multi-levels assembly system under random lead times. Manufacturing-INCOM 2015; 48(3): 249–254.
72. Yahia WB, Ayadi O, Masmoudi F. A fuzzy-based negotiation approach for collaborative planning in manufacturing supply chains. Journal of Intelligent Manufacturing 2017; 28(8): 1987–2006.
73. Govindan K, Mina H, Esmaeili A, et al. An integrated hybrid approach for circular supplier selection and closed loop supply chain network design under uncertainty. Journal of Cleaner Production 2020; 242: 1183–1197.
Refbacks
- There are currently no refbacks.
Copyright (c) 2021 Arango Palacio Isabel Cristina

This work is licensed under a Creative Commons Attribution 4.0 International License.
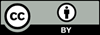
This site is licensed under a Creative Commons Attribution 4.0 International License (CC BY 4.0).
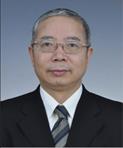
Prof. Zhigeng Pan
Director, Institute for Metaverse, Nanjing University of Information Science & Technology, China
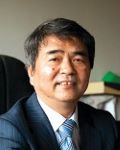
Prof. Jianrong Tan
Academician, Chinese Academy of Engineering, China