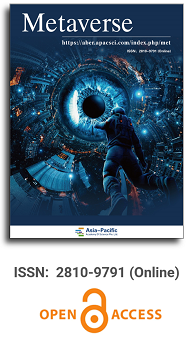
Asia Pacific Academy of Science Pte. Ltd. (APACSCI) specializes in international journal publishing. APACSCI adopts the open access publishing model and provides an important communication bridge for academic groups whose interest fields include engineering, technology, medicine, computer, mathematics, agriculture and forestry, and environment.
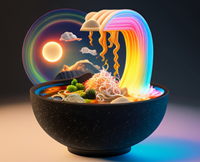
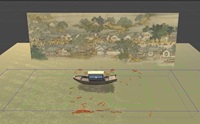
Automatic detection of colorectal polyps using artificial intelligence techniques
Vol 2, Issue 1, 2021
Download PDF
Abstract
Colorectal cancer (CRC) is one of the most prevalent malignant tumors in Colombia and the world. These neoplasms originate in adenomatous lesions or polyps that must be resected to prevent the disease, which can be done with a colonoscopy. It has been reported that during colonoscopy polyps are detected in 40% of men and 30% of women (hyperplastic, adenomatous, serrated, among others), and, on average, 25% of adenomatous polyps (main quality indicator in colonoscopy). However, these lesions are not easy to observe due to the multiplicity of blind spots in the colon and the human error associated with the examination. Objective: to create a computational method for the automatic detection of colorectal polyps using artificial intelligence in recorded videos of real colonoscopy procedures. Methodology: public databases with colorectal polyps and a data collection built in a University Hospital were used. Initially, all the frames of the videos were normalized to reduce the high variability between databases. Subsequently, the polyp detection task is done with a deep learning method using a convolutional neural network. This network is initialized with weights learned on millions of national images from the ImageNet database. The weights of the network are updated using colonoscopy images, following the tuning technique.
Keywords
References
1. Bray F, Ferlay J, Soerjomataram I, et al. Global cancer statistics 2018: Globocan estimates of incidence and mortality worldwide for 36 cancers in 185 countries. CA: A Cancer Journal for Clinicians 2018; 68(6): 394–424.
2. Data I. Method L. Globocan Colombia 2018; 2018(380): 1–2.
3. Samadder NJ, Curtin K, Tuohy TM, et al. Characteristics of missed or interval colorectal cancer and patient survival: A population-based study. Gastroenterology 2014; 146(4): 950–960.
4. Sano Y, Friedland S, Soetikno R. American Gastroenterological Association (AGA) Institute technology assessment on image-enhanced endoscopy. Gastroenterology 2008; 134(1): 327–340.
5. Brown SR, Baraza W, Din S, et al. Chromoscopy versus conventional endoscopy for the detection of polyps in the colon and rectum. Cochrane Database of Systematic Reviews 2016; 4: 6439–6448.
6. Lambert R. The Paris endoscopic classification of superficial neoplastic lesions: esophagus, stomach, and colon: November 30 to December 1, 2002. Gastrointestinal Endoscopy 2003; 58(6Suppl): S3–43.
7. Dinesen L, Chua TJ, Kaffes AJ. Meta-analysis of narrowband imaging versus conventional colonoscopy for adenoma detection. Gastrointestinal Endoscopy 2012; 75(3): 604–611.
8. Nagorni A, Bjelakovic G, Petrovic B. Narrow band imaging versus conventional white light colonoscopy for the detection of colorectal polyps. Cochrane Database of Systematic Reviews 2012; 1: 8361–8372.
9. Jin X, Chai T, Shi J, et al. Meta-analysis for evaluating the accuracy of endoscopy with narrow band imaging in detecting colorectal adenomas. Journal of Gastroenterology and Hepatology 2012; 27(5): 882–897.
10. Komeda Y, Suzuki N, Sarah M, et al. Factors associated with failed polyp retrieval at screening colonoscopy. Gastrointestinal Endoscopy 2013; 77(3): 395–400.
11. Choi HN, Kim HH, Oh JS, et al. Factors influencing the miss rate of polyps in a tandem colonoscopy study. The Korean Journal of Gastroenterology 2014; 64(1): 24–30.
12. Rijn JC, Reitsma JB, Stoker J, et al. Polyp miss rate determined by tandem colonoscopy: A systematic review. American Journal of Gastroenterology 2006; 101(2): 343–350.
13. Bernal J, Sánchez J, Vilarino F. Towards automatic polyp detection with a polyp appearance model. Pattern Recognition 2012; 45(9): 3166–3182.
14. Younghak S, Balasingham I. Comparison of hand-craft feature based SVM and CNN based deep learning framework for automatic polyp classification. Annual International Conference of the IEEE Engineering in Medicine and Biology Society (EMBC) 2017; 20(1): 3277–3280.
15. Urban G, Tripathi P, Alkayali M, et al. Deep learning localizes and identifies polyps in real time with 96% accuracy in screening colonoscopy. Gastroenterology 2018; 155(4): 1069–1078.
16. Taha B, Werghi N, Dias J. Automatic polyp detection in endoscopy videos: A survey. BioMedical Engineering 2017; 3: 852-861.
17. Szegedy C, Vanhoucke V, Ioffe S, et al. Rethinking the inception architecture for computer vision. Proceedings of the IEEE Computer Society Conference on Computer Vision and Pattern Recognition 2016; 28: 18–26.
18. Simonyan K, Zisserman A. Very deep convolutional networks for large-scale image recognition. ICLR 2015; 2: 1–14.
19. He K, Zhang X, Ren S, et al. Deep residual learning for image recognition. 2016 IEEE Conference on Computer Vision and Pattern Recognition. 2016 Dec 12; Las Vegas. New York: IEEE; 2016. p. 770–778.
20. Tajbakhsh N, Gurudu SR, Liang J. Automated polyp detection in colonoscopy videos using shape and context information. IEEE Transactions on Medical Imaging 2016; 35(2): 630–644.
21. Bernal J, Sánchez FJ, Fernández-Esparrach G, et al. WM-DOVA maps for accurate polyp highlighting in colonoscopy: Validation vs. saliency maps from physicians. Computerized Medical Imaging and Graphics 2015; 43: 99–111.
22. Silva J, Histace A, Romain O, et al. Toward embedded detection of polyps in WCE images for early diagnosis of colorectal cancer. International Journal of Computer Assisted Radiology and Surgery) 2014; 9(2): 283–293.
23. Freedman JS, Harari DY, Bamji ND, et al. The detection of premalignant colon polyps during colonoscopy is stable throughout the workday. Gastrointestinal Endoscopy 2011; 73(6): 1197–1206.
Refbacks
- There are currently no refbacks.
Copyright (c) 2021 Martín Alonso Gómez-Zuleta, Diego Fernando Cano-Rosales, Diego Fernando Bravo-Higuera, Josué André Ruano-Balseca, Eduardo Romero-Castro

This work is licensed under a Creative Commons Attribution 4.0 International License.
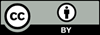
This site is licensed under a Creative Commons Attribution 4.0 International License (CC BY 4.0).
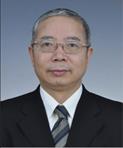
Prof. Zhigeng Pan
Director, Institute for Metaverse, Nanjing University of Information Science & Technology, China
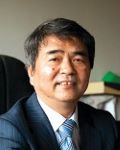
Prof. Jianrong Tan
Academician, Chinese Academy of Engineering, China
Platform: VooV Meeting Meeting ID: 688-583-586...