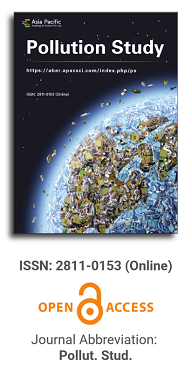
Asia Pacific Academy of Science Pte. Ltd. (APACSCI) specializes in international journal publishing. APACSCI adopts the open access publishing model and provides an important communication bridge for academic groups whose interest fields include engineering, technology, medicine, computer, mathematics, agriculture and forestry, and environment.
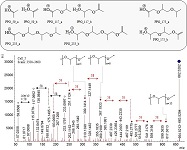
The (partial) replacement of synthetic polymers with bioplastics is due to increased production of conventional packaging plastics causing for severe environmental pollution with plastics waste. The bioplastics, however, represent complex mixtures of known and unknown (bio)polymers, fillers, plasticizers, stabilizers, flame retardant, pigments, antioxidants, hydrophobic polymers such as poly(lactic acid), polyethylene, polyesters, glycol, or poly(butylene succinate), and little is known of their chemical safety for both the environment and the human health. Polymerization reactions of bioplastics can produce no intentionally added chemicals to the bulk material, which could be toxic, as well. When polymers are used to food packing, then the latter chemicals could also migrate from the polymer to food. This fact compromises the safety for consumers, as well. The scarce data on chemical safety of bioplastics makes a gap in knowledge of their toxicity to humans and environment. Thus, development of exact analytical protocols for determining chemicals of bioplastics in environmental and food samples as well as packing polymers can only provide warrant for reliable conclusive evidence of their safety for both the human health and the environment. The task is compulsory according to legislation Directives valid to environmental protection, food control, and assessment of the risk to human health. The quantitative and structural determination of analytes is primary research task of analysis of polymers. The methods of mass spectrometry are fruitfully used for these purposes. Methodological development of exact analytical mass spectrometric tools for reliable structural analysis of bioplastics only guarantees their safety, efficacy, and quality to both humans and environment. This study, first, highlights innovative stochastic dynamics equations processing exactly mass spectrometric measurands and, thus, producing exact analyte quantification and 3D molecular and electronic structural analyses. There are determined synthetic polymers such as poly(ethylenglycol), poly(propylene glycol), and polyisoprene as well as biopolymers in bags for foodstuffs made from renewable cellulose and starch, and containing, in total within the 20,416–17,495 chemicals per sample of the composite biopolymers. Advantages of complementary employment in mass spectrometric methods and Fourier transform infrared spectroscopy is highlighted. The study utilizes ultra-high resolution electrospray ionization mass spectrometric and Fourier transform infrared spectroscopic data on biodegradable plastics bags for foodstuffs; high accuracy quantum chemical static methods, molecular dynamics; and chemometrics. There is achieved method performance |r| = 0.99981 determining poly(propylene glycol) in bag for foodstuff containing 20,416 species and using stochastic dynamics mass spectrometric formulas. The results highlight their great capability and applicability to the analytical science as well as relevance to both the fundamental research and to the industry.
Seasonal dynamics of trace metal concentrations and hydrological parameters in the Balu River, Bangladesh
Vol 5, Issue 2, 2024
Download PDF
Abstract
This study investigates the seasonal dynamics of trace metal concentrations and hydrological parameters in the Balu River, Bangladesh, to inform sustainable water management practices and support efforts to meet environmental standards. Water level, discharge, rainfall, and groundwater data were collected from February to August 2019, alongside sediment samples analyzed for Zn, Pb, Cu, Cr, and Cd concentrations. Statistical analyses, including correlation studies, time series modeling, and Kolmogorov-Smirnov tests, revealed significant seasonal variations. Water levels and discharge rates increased dramatically during the wet season, with upstream levels rising by 862.68% and downstream by 752.92%. Trace metal concentrations showed diverse responses: Cd increased by 554.52%, Zn by 11.86%, while Pb, Cu, and Cr decreased by 44.15%, 5.16%, and 32.72% respectively. Strong correlations were observed between certain metals, particularly in wet periods, with notable relationships between Cr-Cu (r = 0.870) and Zn-Cr (r = 0.742). The integrated analysis of hydrological parameters and metal concentrations, using Gower coefficient and partial correlation analyses, suggests complex interactions between seasonal changes and pollutant dynamics. These findings highlight the need for season-specific water management strategies and more frequent monitoring to better understand and mitigate the environmental impacts of trace metal pollution in the Balu River system. The study contributes valuable insights into the interplay between hydrological conditions and trace metal behavior, essential for developing effective pollution control measures and achieving sustainable river management in Bangladesh.
Keywords
References
1. Venkata Mohan S, Nithila P, Jayarama RS. Estimation of heavy metals in drinking water and development of heavy metal pollution index. J Environ Sci Heal Part A Toxic/Hazardous Subst Environ Eng. 1996; 31(2): 283–289. doi: 10.1080/10934529609376357
2. Nasehi F, Monavari M, Naderi Gh, et al. Investigation of heavy metals accumulation in the sediment and body of carp fish in Aras River. Iran J Fish Sci. 2013; 12(2): 398–410.
3. Islam MS, Ahmed MK, Raknuzzaman M, et al. Heavy metal pollution in surface water and sediment: A preliminary assessment of an urban River in a developing country. Ecol Indic. 2015; 48: 282–291.
4. Mondol MN, Chamon AS, Faiz B, Elahi SF. Seasonal variation of heavy metal concentration in water and plant samples around Tejgaon industrial area of Bangladesh. J Bangladesh Acad Sci. 2011; 35(1): 19–41.
5. Kihampa C. Heavy metal contamination in water and sediment downstream of municipal wastewater treatment plants, Dar es Salaam, Tanzania. Int J Environ Sci. 2013; 3(5): 1407–1415.
6. Zhang F, Yan X, Zeng C, et al. Influence of traffic activity on heavy metal concentrations of roadside farmland soil in mountainous areas. Int J Environ Res Public Health. 2012; 9(5): 1715–1731.
7. Ashraf W. Levels of selected heavy metals in tuna fish. Arab J Sci Eng. 2006; 31(1A): 89–92.
8. Terra BF, Araújo FG, Calza CF, et al. Heavy metal in tissues of three fish species from different trophic levels in a tropical Brazilian River. Water Air Soil Pollut. 2008; 187(1–4): 275–284.
9. United Nations. Goal 6: Clean Water and Sanitation in The Sustainable Development Goals Report. 2017; 60. Available online: https://www.unep.org/topics/sustainable-development-goals/why-do-sustainable-development-goals-matter/goal-6-clean-2 (accessed on 10 September 2024)
10. Paul R, Haq A. Challenges of water quality management: case of peripheral Rivers in Dhaka Mega City. In: Proceedings of the presentation from the world water week in Stockholm; 5–11 September 2010; Stockholm, Sweden.
11. A shocking further delay in tannery relocation. Available online: https://www.newagebd.net/article/94630/a-shocking-further-delay-in-tanneryrelocation?fbclid = IwAR2IuKp1Xm1steI4IM YRmWkqt1Sv3f0dGJOZjtUbvfcflrye8RGXVo6WVJk (accessed on 10 September 2024).
12. dark-flows-the-River-turag-1587946@www.thedailystar.net. Available online: https://www.thedailystar.net/star-weekend/environment/dark-flows-the-River-turag-1587946?amp (accessed on 10 September 2024).
13. Lee CSL, Li XD, Zhang G, et al. Heavy metals and Pb isotopic composition of aerosols in urban and suburban areas of Hong Kong and Guangzhou, South China Evidence of the long-range transport of air contaminants. Atmos Environ, 2007; 41(2): 432–447.
14. Li H, Shi A, Li M, Zhang X. Effect of pH, temperature, dissolved oxygen, and flow rate of overlying water on heavy metals release from storm sewer sediments. J Chem. 2013.
15. Duncan AE, de Vries N, Nyarko KB. Assessment of heavy metal pollution in the sediments of the River Pra and its tributaries. Water Air Soil Pollut. 2018; 229(8): 272.
16. Van-Duijn JRC. Diseases of fishes. In: The Lancet. Narendra Publishing House; 1828. Volume 9. p 651.
17. Jatav SK, Ravikant, Dhruve D, et al. Heavy Metal Toxicity in Fishes and Their Impact on Human’s Health: A Review. The Pharma Innovation Journal, vol. SP-12, no. 10, 2023, pp. 1448–1452.
18. Politis MD, Yao M, Gennings C, et al. Associations of Exposure to Urinary Metals with Kidney Injury Biomarkers among Pre-adolescent Children: A Cross-Sectional Study. In: Proceedings of the 35th Annual Conference of the International Society of Environmental Epidemiology (ISEE 2023); 17–21 September 2023; Taiwan, China.
19. Kitala K, Tanski D, Godlewski J, et al. Copper and Zinc Particles as Regulators of Cardiovascular System Function—A Review. Nutrients. 2023; 15(13): 3040. doi: 10.3390/nu15133040
20. Layek M, Khatun N, Karmakar P, et al. Toxicity of Hexavalent Chromium: Review. In: Kumar N, Walther C, Gupta DK. (editors). Chromium in Plants and Environment. Springer; 2023.
21. Naz S, Chatha AMM, Téllez-Isaías G, et al. A comprehensive review on metallic trace elements toxicity in fishes and potential remedial measures. Water. 2023; 15(16): 3017. doi: 10.3390/w15163017
22. Emon FJ, Rohani MF, Sumaiya N, et al. Bioaccumulation and bioremediation of heavy metals in fishes—A review. Toxics. 2023; 11(6): 510. doi: 10.3390/toxics11060510
23. WHO. World Health Organization. Available online: https://iris.who.int/handle/10665/38551 (accessed on 10 September 2024).
24. Saha PK, Hossain M. Geochemical and ecotoxical approach for evaluation of heavy metal pollution in the Buriganga River sediment. In: Proceedings of the Bangladesh Geotechnical Conference; 03–06 November 2010; Dhaka, Bangladesh.
25. Islam MS, Chowdhury MAH, Billah MMS, et al. Investigation of effluent quality discharged from the textile industry of Purbani Group, Gazipur, Bangladesh and it’s textile industry of Purbani Group, Gazipur, Bangladesh and it’s management. Bangladesh J Environ Sci. 2012; 23: 123–130.
26. Yahaya M, Mohammad S, Abdullahi B. Seasonal variations of heavy metals concentration in abattoir dumping site soil in Nigeria. J Appl Sci Environ Manag. 2010; 13(4): 9–13.
27. Mohiuddin KM, Otomo K, Ogawa Y, Shikazono N. Seasonal and spatial distribution of trace elements in the water and sediments of the Tsurumi River in Japan. Environ Monit Assess. 2012; 184(1): 265–279.
28. Close tanneries at Hazaribagh_The Daily Star. Available online: https://www.thedailystar.net/frontpage/close-tanneries-hazaribagh-1372036 (accessed on 10 September 2024).
29. Buriganga River. Available online: https://en.wikipedia.org/wiki/Buriganga_River (accessed on 10 September 2024).
30. Savar tannery ETP stuck by a string_The Business Standard. Available online: https://www.tbsnews.net/economy/savar-cetp-stuck-98-two-years (accessed on 10 September 2024)
31. Islam MS, Ahmed MK, Habibullah-Al-Mamun M, Hoque MF. Preliminary assessment of heavy metal contamination in surface sediments from a River in Bangladesh. Environ Earth Sci. 2015; 73(4): 1837–1848.
32. Whitehead PG, Bussi G, Peters R, et al. Modelling heavy metals in the Buriganga River System, Dhaka, Bangladesh: impacts of tannery pollution control. Sci Total Environ. 2019; 697: 134090.
33. Nürnberg HW. The voltammetric approach in trace metal chemistry of natural waters and atmospheric precipitation. Anal Chim Acta. 1984; 164(C): 1–21.
34. Abdullah F, Mamoon WB, Islam AS, et al. Hydrological responses of the Brahmaputra river basin using CMIP6 GCM projections for supporting climate resilient infrastructure design. Sustainable and Resilient Infrastructure. 2024; 1–27. doi: 10.1080/23789689.2024.2371741
35. Ramabrahmam K, and Venkatareddy K. Trend Analysis of Climate Variables and Extremes Over Maner River Basin, India. In: International Virtual Conference on Developments and Applications of Geomatics. Springer Nature Singapore; 2022. pp. 509–517.
36. Singhal P, Goel MK, Bronstert A, et al. Understanding the Flood Seasonality in the Himalayan River Basin under Changing Climate. 2024; Preprint (Version 1). doi: 10.21203/rs.3.rs-4550138/v1
37. Salviya LL, Taryana D, Masruroh H, Aristin NF. Estimation of erosion using the modified universal soil loss equation (musle) method at SUB das bale. Journal of Innovative Integration and Harmony of Social Sciences. 2023; 3(3): 313–334. doi: 10.17977/um063v3i3p313–334
38. Banu M, Jahan B, Mondal MN, Hossain MA. Toxicity Effects of Metals Bioaccumulation in Water and Fishes of the Balu River, Bangladesh. Egyptian Journal of Aquatic Biology & Fisheries. 2023; 27(3). doi: 10.21608/ejabf.2023.301087
39. Rahman MM, Islam MA, Khan MB. Status of heavy metal pollution of water and fishes in Balu and Brahmaputra rivers. Progressive Agriculture. 2016; 27(4): 444–452. doi: 10.3329/pa.v27i4.32129
40. Odora AT, Aysha S, Sultan MB, Bhuiyan MAR. Evaluating the sources of microplastic contamination and quantifying its abundance in the Balu River, Dhaka, Bangladesh. Environmental Monitoring and Assessment. 2024; 196(9): 867. doi: 10.1007/s10661-024-13051-w
41. Islam MS, Karim MR, Islam MT, et al. Abundance, characteristics, and ecological risks of microplastics in the riverbed sediments around Dhaka city. Science of The Total Environment. 2023a; 877: 162866. doi: 10.1016/j.scitotenv.2023.162866
42. Hasan MK, Happy MA, Nesha MK, Karim KR. Pollution status of Balu river due to industrial input at Dhaka, Bangladesh. Open Journal of water pollution and treatment. 2014; 1(1): 34–42. doi: 10.15764/wpt.2014.01004
43. Soil Survey Staff. Soil Taxonomy: A Basic System of Soil Classification for Making and Interpreting Soil Surveys, 2nd ed. In: Agriculture Handbook, No. 436, Natural Resources Conservation Service, United States Department of Agriculture. U.S. Government Printing Office Washington; 1999. p. 886.
44. Richardson S, Mayes WM, Faetsch S, et al. Mixtures of sediment chemical contaminants at freshwater sampling sites across Europe with different contaminant burdens. Science of The Total Environment. 2023; 891: 164280. doi: 10.1016/j.scitotenv.2023.164280
45. Risacher FF, Schneider H, Drygiannaki I, et al. A review of peeper passive sampling approaches to measure the availability of inorganics in sediment porewater. Environmental Pollution. 2023; 328: 121581. doi: 10.1016/j.envpol.2023.121581
46. Quirino DF, Lima NS, Palma MN, et al. Evaluation of Heating Times for Loss on Drying at 105℃ for Estimation of Laboratory Dry Matter in Animal Feeds. Journal of AOAC International. 2023; 106(2): 261–266. doi: 10.1093/jaoacint/qsad004
47. Binder GA, Metcalf R, Atlas Z, Daniel KG. Evaluation of digestion methods for analysis of trace metals in mammalian tissues and NIST 1577c. Analytical biochemistry. 2018; 543: 37–42. doi: 10.1016/j.ab.2017.11.016
48. Hossain MM, Jahan I, Al Nahian A, et al. Immediate health risk: Concentration of heavy metals in contaminated freshwater fishes from the river channel of Turag‐Tongi‐Balu. Environmental Toxicology. 2024; 39(1): 120–34. doi: 10.1002/tox.23959
49. Atiq AR, Rahaman MM, Sojib RM, Shil SC. Water quality changes in Balu River during non-monsoon and monsoon period. Journal of Water Resource Engineering and Management. 2015; 2: 38–49.
50. Islam MZ, Noori A, Islam R, et al. Assessment of the contamination of trace metal in Balu River water, Bangladesh. Journal of Environmental Chemistry and Ecotoxicology. 2012; 4(14): 242–249. doi: 10.5897/JECE10.025
51. Hasan MK, Khan MRI, Nesha MK, Happy MA. Analysis of water quality using chemical parameters and metal status of Balu River at Dhaka, Bangladesh. Open J. Water Pollut. Treat. 2014; 1(2): 58–74. doi: 10.15764/WPT.2014.02007
52. Oruko RO, Edokpayi JN, Msagati TA, et al. Investigating the chromium status, heavy metal contamination, and ecological risk assessment via tannery waste disposal in sub-Saharan Africa (Kenya and South Africa). Environmental Science and Pollution Research. 2021; 28: 42135–42149. doi: 10.1007/s11356-021-13703-1
53. Murtaza G, Usman M. Assessment of various heavy metals level in groundwater and soil at tannery manufacturing areas of three mega cities (Sialkot, Lahore and Karachi) of Pakistan. Desalination and Water Treatment. 2022; 266: 121–130. doi: 10.5004/dwt.2022.28640
54. Lerat-Hardy A, Coynel A, Schäfer J, et al. Impacts of highway runoff on metal contamination including rare earth elements in a small urban watershed: case study of Bordeaux Metropole (SW France). Archives of Environmental Contamination and Toxicology. 2021; 1–21. doi: 10.1007/s00244-021-00816-4
55. Charters FJ, O’Sullivan AD, Cochrane TA. Influences of zinc loads in urban catchment runoff: Roof type, land use type, climate and management strategies. Journal of Environmental Management. 2022; 322: 116076. doi: 10.1016/j.jenvman.2022.116076
56. Gómez-Alvarez A, Meza-Figueroa D, Valenzuela-García JL, et al. Behavior of Metals Under Different Seasonal Conditions: Effects on the Quality of a Mexico–USA Border River. Water, Air, & Soil Pollution. 2014; 225: 1–13. doi: 10.1007/s11270-014-2138-z
57. O’Connor AE, Canuel EA, Beck AJ. Drivers and seasonal variability of redox-sensitive metal chemistry in a shallow subterranean estuary. Frontiers in Environmental Science. 2022; 9: 613191. doi: 10.3389/fenvs.2021.613191
58. da Silva EP, Simões TR, Antoniosi Filho NR, et al. Effect of rainfall characteristics on the transport of trace metals in suspended particles during rainfall events. Journal of Hydrology. 2024; 634: 131062. doi: 10.1016/j.jhydrol.2024.131062
59. Roy S, Banna LN, Hossain M, Rahman H. Water quality of Narai canal and Balu river of Dhaka City: An impact of industrialization. Journal of the Bangladesh Agricultural University. 2014; 12(2): 285–290. doi: 10.22004/ag.econ.211242
60. Sami MG, Sikder SP. Healing Balu River: Restoration of river eco-system through revitalizing the riparian zone of Balu River, Dhaka. ResearchGate, 2020. doi: 10.13140/RG.2.2.25999.51367
61. Bhuiyan NZ., Hossain MB, Ali MM, et al. Seasonal variation of trace elements in water and sediment of the Turag and Balu Rivers, Bangladesh. Egypt. J. Aquat. Biol. Fish. 2022; 26.
Refbacks
- There are currently no refbacks.
Copyright (c) 2024 Md. Khairul Amin, Maiesha Nujhat, Abu Hena Mostafa Kamal, Sheikh Md. Ruhul Amin, Wahida Khatun, Joynab Akther, Mir Mohtadi Ibtesum
License URL: https://creativecommons.org/licenses/by/4.0/
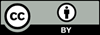
This site is licensed under a Creative Commons Attribution 4.0 International License (CC BY 4.0).
.jpg)
Beijing University of Technology, China