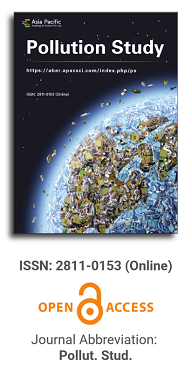
Asia Pacific Academy of Science Pte. Ltd. (APACSCI) specializes in international journal publishing. APACSCI adopts the open access publishing model and provides an important communication bridge for academic groups whose interest fields include engineering, technology, medicine, computer, mathematics, agriculture and forestry, and environment.
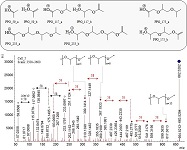
The (partial) replacement of synthetic polymers with bioplastics is due to increased production of conventional packaging plastics causing for severe environmental pollution with plastics waste. The bioplastics, however, represent complex mixtures of known and unknown (bio)polymers, fillers, plasticizers, stabilizers, flame retardant, pigments, antioxidants, hydrophobic polymers such as poly(lactic acid), polyethylene, polyesters, glycol, or poly(butylene succinate), and little is known of their chemical safety for both the environment and the human health. Polymerization reactions of bioplastics can produce no intentionally added chemicals to the bulk material, which could be toxic, as well. When polymers are used to food packing, then the latter chemicals could also migrate from the polymer to food. This fact compromises the safety for consumers, as well. The scarce data on chemical safety of bioplastics makes a gap in knowledge of their toxicity to humans and environment. Thus, development of exact analytical protocols for determining chemicals of bioplastics in environmental and food samples as well as packing polymers can only provide warrant for reliable conclusive evidence of their safety for both the human health and the environment. The task is compulsory according to legislation Directives valid to environmental protection, food control, and assessment of the risk to human health. The quantitative and structural determination of analytes is primary research task of analysis of polymers. The methods of mass spectrometry are fruitfully used for these purposes. Methodological development of exact analytical mass spectrometric tools for reliable structural analysis of bioplastics only guarantees their safety, efficacy, and quality to both humans and environment. This study, first, highlights innovative stochastic dynamics equations processing exactly mass spectrometric measurands and, thus, producing exact analyte quantification and 3D molecular and electronic structural analyses. There are determined synthetic polymers such as poly(ethylenglycol), poly(propylene glycol), and polyisoprene as well as biopolymers in bags for foodstuffs made from renewable cellulose and starch, and containing, in total within the 20,416–17,495 chemicals per sample of the composite biopolymers. Advantages of complementary employment in mass spectrometric methods and Fourier transform infrared spectroscopy is highlighted. The study utilizes ultra-high resolution electrospray ionization mass spectrometric and Fourier transform infrared spectroscopic data on biodegradable plastics bags for foodstuffs; high accuracy quantum chemical static methods, molecular dynamics; and chemometrics. There is achieved method performance |r| = 0.99981 determining poly(propylene glycol) in bag for foodstuff containing 20,416 species and using stochastic dynamics mass spectrometric formulas. The results highlight their great capability and applicability to the analytical science as well as relevance to both the fundamental research and to the industry.
Analysis of air quality pollution in Chengdu utilizing logistic regression for multi-class classification based on the distance metric between classes
Vol 2, Issue 1, 2021
Download PDF
Abstract
The health of individuals is intimately linked to air quality, making it crucial to investigate the pollutants that influence it. A sequential Logistic multi-classification approach, which utilizes the distance between classes, was applied to analyze the air quality data of Chengdu spanning from May 2019 to April 2020. By leveraging the inter-class distance metric, the multi-class classification challenge was converted into a series of binary classification tasks. Using a sequential strategy, binary Logistic regression was then employed for each task. The accuracy rate post-stepwise regression was utilized to assess the impact of various pollutants on air quality. The findings indicate that PM2.5, PM10, NO2, and O3 are the four primary pollutants with the most significant collective influence on Chengdu's air quality. Consequently, the government should enhance the joint monitoring of these pollutants and develop targeted policies to mitigate their levels.
Keywords
References
- Zhang B, Li J, Zhao J, et al. Effects of aeolian dust on urban air quality in Inner Mongolia grassland. Science Technology and Engineering. 2018; 18(3): 123-131.
- Liu L, Zhao Q, Wang J, et al. Analysis of a winter-time heavily polluted weather process in the Yangtze river Delta urban agglomeration. Science Technology and Engineering. 2019; 19(26): 376-383.
- Xu M, Zhang L, Zheng N, et al. Analysis on the change trend and pollution characteristics of urban air quality after revision of ambient air quality standards: take Jinan City as an example. Science Technology and Engineering. 2020; 20(13): 5422-5428.
- Li N, Wei X, Zhou Y, et al. Source analysis and health risk assessment of polycyclic aromatic hydrocarbons in atmospheric environment PM2.5 in Changchun City. Science Technology and Engineering. 2021; 21(1): 410-416.
- Xie S, Zhou Z, Li G. Relationship between PM2.5 concentration and meteo-logical factors in Nanning. Science Technology and Engineering. 2020; 20(1): 460-466.
- Zhang X, Yu L, Sun M, et al. Distribution and elemental analysis of particulate matter PM2.5, PM10 in the atmosphere of spring in Jinan City. Science Technology and Engineering. 2018; 18(25): 278-285.
- Tian H. Linear regression model estimation method with ordinal multi-category explanatory variables and its application research. Chengdu: Southwest Jiaotong University; 2019.
- Li J. Multi-classification research based on Logistic regression model and support vector machine (SVM) model. Wuhan: Central China Normal University; 2014.
- Murphy PM, Ahad W. UCI repository of machine learning database. Available online: https://archive.ics.uci.edu/ (accessed on 13 April 2020).
- Wang Y. PM2.5 air quality data, historical data and meteorological data - data network. Available online: http:// www.shu-ju. net/ (accessed on 13 April 2020).
- Wu Y. Weather report. Available online: http://www.tianqihoubao.com (accessed on 13 April 2020).
- Chengdu Bureau of Statistics. Chengdu statistical yearbook 2018. Beijing: China Statistics Press; 2018.
- Urban Planning Newsletter. Baidu Maps released the China Urban transport research report for the Second Quarter of 2018. Urban Planning Newsletter. 2018(14): 14.
- Cao Y, Wang C, Zhao X, et al. Analysis of the characteristics of PM2.5 pollution in Chengdu and its relationship with surface meteorological elements. Mid-low Latitude Mountain Meteorology. 2020; 44(4): 59-64.
- Li P, Luo B, Zhang W, et al. Analysis of temporal and spatial distribution characteristics and pollution characteristics of ozone in Sichuan Province. Environmental Science and Technology. 2018; 41(S1): 293-298.
- Yang X, Yi J, Lü Y, et al. Analysis of the causes of severe ozone pollution in Chengdu and surrounding areas. China Environmental Science. 2020; 40(5): 2000-2009.
Supporting Agencies
Copyright (c) 2021 Ting-Ting Li, Wang-Yong Lv, Jiao Zhou, Shi-Jing Zeng
License URL: http://creativecommons.org/licenses/by/4.0/
This site is licensed under a Creative Commons Attribution 4.0 International License (CC BY 4.0).
.jpg)
Beijing University of Technology, China