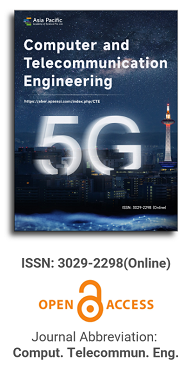
Automated leaderboard system for hackathon evaluation using large language models
Vol 3, Issue 1, 2025
Download PDF
Abstract
Keywords
References
- Gama K, Valença G, Alessio P, et al. The Developers’ Design Thinking Toolbox in Hackathons: A Study on the Recurring Design Methods in Software Development Marathons. Software Engineering. 2022.
- Steglich C, Marczak S, Guerra L, et al. An Online Educational Hackathon to Foster Professional Skills and Intense Collaboration on Software Engineering Students. In: Proceedings of the XXXV Brazilian Symposium on Software Engineering; 27 September–1 October 2021; Joinville, Brazil. pp. 388–397.
- Porras J, Khakurel J, Ikonen J, et al. Hackathons in Software Engineering Education: Lessons Learned from a Decade of Events. In: Proceedings of the 2nd International Workshop on Software Engineering Education for Millennials; 27 May–3 June 2018; Gothenburg, Sweden. pp. 40–47.
- Kumalakov B, Kim A, Mukhtarova S, et al. Hackathon as a Project-Based Teaching Tool: Employing Programming Challenge in the Class. In: Proceedings of the 2018 IEEE 12th International Conference on Application of Information and Communication Technologies (AICT); 17–19 October 2018; Almaty, Kazakhstan. pp. 1–5.
- Sadovykh A, Beketova M, Khazeev M. Hackathons as a Part of Software Engineering Education: Case in Tools Example. In: Proceedings of the Frontiers in Software Engineering Education: First International Workshop; 11–13 November 2019; Villebrumier, France. pp. 232–245.
- Steglich C, Salerno L, Fernandes T, et al. Hackathons as a Pedagogical Strategy to Engage Students to Learn and to Adopt Software Engineering Practices. In: Proceedings of the XXXIV Brazilian Symposium on Software Engineering; 21–23 October 2020; Natal, Brazil. pp. 670–679.
- Gama K, Alencar B, Calegario F, et al. A Hackathon Methodology for Undergraduate Course Projects. In: Proceedings of the 2018 IEEE Frontiers in Education Conference (FIE); 3–6 October 2018; San Jose, CA, USA. pp. 1–9.
- Farazouli A. Automation and Assessment: Exploring Ethical Issues of Automated Grading Systems from a Relational Ethics Approach. Springer; 2024.
- Lagakis P, Demetriadis S, Psathas G. Automated Grading in Coding Exercises Using Large Language Models. Springer; 2024.
- Yousef M, Mohamed K, Medhat W, et al. BeGrading: Large Language Models for Enhanced Feedback in Programming Education. Neural Computing and Applications. 2024.
- Mosqueira-Rey E, Hernández-Pereira E, Alonso-Ríos D, et al. Human-in-the-Loop Machine Learning: A State of the Art. Artificial Intelligence Review. 2023; 56; 3005–3054.
- RMIT GenAI and Cyber Security Hackathon. Available online: https://www.kaggle.com/competitions/rmit-gen-ai-and-cyber-security-hackathon (accessed on 2 December 2024).
- Kaggle. Available online: https://www.kaggle.com/ (accessed on 2 December 2024).
- González-Carrillo CD, Restrepo-Calle F, Ramírez-Echeverry JJ, González FA. Automatic Grading Tool for Jupyter Notebooks in Artificial Intelligence Courses. Sustainability. 2021; 13(21): 12050.
- Kaggle. Kaggle API Documentation. Available online: https://www.kaggle.com/docs/api (accessed on 2 December 2024).
- Amazon Bedrock. Amazon Bedrock Documentation. Available online: https://docs.aws.amazon.com/bedrock/ (accessed on 4 December 2024).
- Amazon Web Services. AWS Certified Cloud Practitioner. Available online: https://aws.amazon.com/certification/certified-cloud-practitioner/ (accessed on 4 December 2024).
- Guskey TR. Special Topic/The Case Against Percentage Grades. Educational Leadership. 2013; 71(1): 68–72.
- National Center for Education Statistics, 2025. Scale Scores and NAEP Achievement Levels. Available online: https://nces.ed.gov/nationsreportcard/guides/scores_achv.aspx (accessed on 4 December 2024).
- Bland JM, Altman DG. Statistical methods for assessing agreement between two methods of clinical measurement. The Lancet. 1986; 327(8476): 307–310.
Refbacks
- There are currently no refbacks.
Copyright (c) 2025 Author(s)
License URL: https://creativecommons.org/licenses/by/4.0/
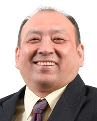
Prof. Maode Ma
Qatar University, Qatar
-
-
-
-
-
-
-
-
- J-Gate
-
-
-
-
-
-
The field of computer and telecommunications engineering is rapidly advancing, with the following being some of the latest developments.
more
We are pleased to congratulate the first anniversiry of the journal of Computer and Telecommunication Engineering (CTE).
more
Owing to the tireless dedication of the editor-in-chief, editorial board members, and the in-house editorial team, we are proud to announce the successful online launch of the first issue of Computer and Telecommunication Engineering.
Asia Pacific Academy of Science Pte. Ltd. (APACSCI) specializes in international journal publishing. APACSCI adopts the open access publishing model and provides an important communication bridge for academic groups whose interest fields include engineering, technology, medicine, computer, mathematics, agriculture and forestry, and environment.