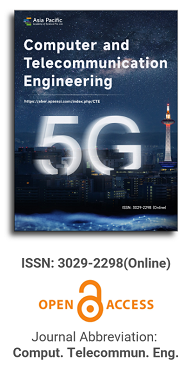
Event-triggered reinforcement learning-based internet data bandwidth allocation technique as a metric for balanced QoS and QoE
Vol 2, Issue 4, 2024
Download PDF
Abstract
Keywords
References
1. Adeel A, Gogate M, Farooq S, et al. A Survey on the Role of Wireless Sensor Networks and IoT in Disaster Management. In: Geological Disaster Monitoring Based on Sensor Networks. Springer, Singapore; 2019. pp. 57–66.
2. Abusitta A, de Carvalho GHS, Wahab OA, et al. Deep learning-enabled anomaly detection for IoT systems. Internet of Things. 2023; 21: 100656. doi: 10.1016/j.iot.2022.100656
3. Showail A, Tahir R, Zaffar MF, et al. An internet of secure and private things: A service-oriented architecture. Computers & Security. 2022; 120: 102776. doi: 10.1016/j.cose.2022.102776
4. Suliman AT, Kadadha M, Mizouni R, et al. Blockcheck: A consortium blockchain-based conformance checking framework for business processes. Internet of Things. 2023; 21: 100652. doi: 10.1016/j.iot.2022.100652
5. Nasrallah A, Balasubramanian V, Thyagaturu AS, et al. Reconfiguration algorithms for high precision communications in time sensitive networks: Time-aware shaper configuration with IEEE 802.1Qcc. ITU Journal on Future and Evolving Technologies. 2021; 2(1): 13-34. doi: 10.52953/sivv2522
6. Tzavaras A, Mainas N, Petrakis EGM. OpenAPI framework for the Web of Things. Internet of Things. 2023; 21: 100675. doi: 10.1016/j.iot.2022.100675
7. Perez AJ, Siddiqui F, Zeadally S, et al. A review of IoT systems to enable independence for the elderly and disabled individuals. Internet of Things. 2023; 21: 100653. doi: 10.1016/j.iot.2022.100653
8. Shakil A, Khalighi MA, Pudlo P, et al. Outlier detection in non-stationary time series applied to sewer network monitoring. Internet of Things. 2023; 21: 100654. doi: 10.1016/j.iot.2022.100654
9. Amichai-Hamburger Y, Fine A, Goldstein A. The Impact of Internet Interactivity and Need for Closure on Consumer Preference. Computers in Human Behaviour. 2004; 20(1): 103-117. doi: 10.1016/S0747-5632(03)00041-4
10. Garnaev A, Trappe W, Mandayam NB, Poor HV. A multi-link communication connectivity game under hostile interference. ITU Journal on Future and Evolving Technologies. 2021; 2(1): 101-112. doi: 10.52953/swhp5412
11. Sinha A, Chowdhury D, Sharma S, et al. nCare: Fault-aware edge intelligence for rendering viable sensor nodes. Internet of Things. 2023; 21: 100643. doi: 10.1016/j.iot.2022.100643
12. Dwivedi AD, Srivastava G. Security analysis of lightweight IoT encryption algorithms: SIMON and SIMECK. Internet of Things. 2023; 21: 100677. doi: 10.1016/j.iot.2022.100677
13. Balasubramaniam S, Vijesh Joe C, Sivakumar TA, et al. Optimization Enabled Deep Learning‐Based DDoS Attack Detection in Cloud Computing. Qi L, ed. International Journal of Intelligent Systems. 2023; 2023(1). doi: 10.1155/2023/2039217
14. Bian J, Arafat AA, Xiong H, et al. Machine Learning in Real-Time Internet of Things (IoT) Systems: A Survey. IEEE Internet of Things Journal. 2022; 9(11): 8364-8386. doi: 10.1109/jiot.2022.3161050
15. Mitton N, Foubert B. RODENT: a flexible TOPSIS based routing protocol for multi-technology devices in wireless sensor networks. ITU Journal on Future and Evolving Technologies. 2021; 2(1): 89-100. doi: 10.52953/fkro8431
16. Lampson B, Srinivasan V, Varghese G. IP lookups using multiway and multicolumn search. IEEE/ACM Transactions on Networking. 1999; 7(3): 324-334. doi: 10.1109/90.779199
17. Bzai J, Alam F, Dhafer A, et al. Machine Learning-enabled Internet of Things (IoT): Data, Applications, and Industry Perspective. Electronics Journal. 2022; 11(17): 2676.
18. Cai Z, Shu Y, Su X, et al. A traffic data interpolation method for IoT sensors based on spatio-temporal dependence. Internet of Things. 2023; 21: 100648. doi: 10.1016/j.iot.2022.100648
19. Chatterjee A, Ahmed BS. IoT anomaly detection methods and applications: A survey. Internet of Things. 2022; 19: 100568. doi: 10.1016/j.iot.2022.100568
20. Chauhan N, Banka H, Agrawal R. Adaptive bandwidth adjustment for resource constrained services in fog queueing system. Cluster Computing. 2021; 24(4): 3837-3850. doi: 10.1007/s10586-021-03378-1
21. Chen J, Touati C, Zhu Q. Optimal Secure Two-Layer IoT Network Design. IEEE Transactions on Control of Network Systems. 2020; 7(1): 398-409. doi: 10.1109/tcns.2019.2906893
22. IEEE Transaction Control Networking Systems. Available online: https://ieeexplore.ieee.org/xpl/RecentIssue.jsp?punumber=6509490 (accessed on 16 November 2024).
23. Chen Z, Cheng Z, Luo W, et al. FSMFA: Efficient firmware-secure multi-factor authentication protocol for IoT devices. Internet of Things. 2023; 21: 100685. doi: 10.1016/j.iot.2023.100685
24. Comer DE. Computer Networks and Internets, 2nd ed. Prentice Hall, New Jersey, USA; 1997.
25. Miller D. Data Communications and Networks, 1st ed. McGraw- Hill New York USA; 2006.
26. De A, Roy B, Bhattacharya A, et al. Bandwidth‐Enhanced Ultra‐Wide Band Wearable Textile Antenna for Various WBAN and Internet of Things (IoT) Applications. Radio Science. 2021; 56(11). doi: 10.1029/2021rs007315
27. Chaudhary D, Soni T, Vasudev KL, et al. A modified lightweight authenticated key agreement protocol for Internet of Drones. Internet of Things. 2023; 21: 100669. doi: 10.1016/j.iot.2022.100669
28. Dibal PY, Onwuka EN, Zubair S, et al. Processor power and energy consumption estimation techniques in IoT applications: A review. Internet of Things. 2023; 21: 100655. doi: 10.1016/j.iot.2022.100655
29. Fauzy D, Moussa SM, Badri NI. An IoT-based Resource Utilization Framework Using Data Fusion for Smart Environments. Journal of Internet of Things. 2023; 21: 100645. doi: 10.1016/j.iot.2022.100645
30. Fountoulakis E, Pappas N, Ephremides A. Dynamic power control for time-critical networking with heterogeneous traffic. ITU Journal on Future and Evolving Technologies. 2021; 2(1): 1-12. doi: 10.52953/sagv1643
31. Enjie Liu, Youbing Zhao, Abimbola Efunogbon. Boosting smarter digital health care with 5G and beyond networks. ITU Journal on Future and Evolving Technologies. 2023; 4(1): 157-165. doi: 10.52953/gjnn6958
32. Kadir EA, Shibair R, Rahim SKA, et al. B5G and 6G: Next Generation Wireless Communications Technologies, Demand and Challenges. Journal of IEEE-Xplore; 2021.
33. Ghanbari Z, Jafari Navimipour N, Hosseinzadeh M, et al. Resource allocation mechanisms and approaches on the Internet of Things. Cluster Computing. 2019; 22(4): 1253-1282. doi: 10.1007/s10586-019-02910-8
34. Goncalves, Marcus, Niles, Kitty. IPV6 Networks, 1st ed. McGraw-Hill, New York, USA; 1998.
35. Habeeb F, Alwasel K, Noor A, et al. Dynamic Bandwidth Slicing for Time-Critical IoT Data Streams in the Edge-Cloud Continuum. IEEE Transactions on Industrial Informatics. 2022; 18(11): 8017-8026. doi: 10.1109/tii.2022.3169971
36. Hsu SH, Lin CH, Wang CY, et al. Breaking Bandwidth Limitation for Mission-Critical IoT Using Semisequential Multiple Relays. IEEE Internet of Things Journal. 2018; 5(5): 3316-3329. doi: 10.1109/jiot.2017.2776403
37. Hui J, Gan C, Liu X, et al. A Dynamic Bandwidth Allocation Algorithm Based on Differentiated Service Cycle in Multi-Service Hybrid VPON. Fiber and Integrated Optics. 2022; 41(5-6): 113-130. doi: 10.1080/01468030.2022.2150588
38. Huitema, Christian. Routing in the Internet. 1st ed. Prentice Hall, New Jersey, USA; 1995.
39. Huitema, Christian. IPV6-The New Internet Protocol, 2nd ed. Prentice Hall, New Jersey, USA; 1996.
40. Irfan M, Jawad H, Felix BB, et al. Non-wearable IoT-based Smart Ambient Behaviour Observation System. IEEE Sensors Journal. 2021; 21(18).
41. Islam MS, Islam MT, Ullah MDA, et al. A Modified Meander Line Microstrip Patch Antenna With Enhanced Bandwidth for 2.4 GHz ISM-Band Internet of Things (IoT) Applications. IEEE Access. 2019; 7: 127850-127861. doi: 10.1109/access.2019.2940049
42. Gódor I, Yahia IGB. Topics from networks management and operations. International Journal of Network Management. 2021; 32(1). doi: 10.1002/nem.2182
43. Ito Y, Koga H, Iida K. A Bandwidth Reallocation Scheme to Improve Fairness and Link Utilization in Data Centre Networks. IEEE Xplore; 2016.
44. Kurose JF, Ross KW. Computer Networking: A top down approach featuring the Internet, 1st ed. Addison - Wesley, USA; 2000.
45. Liang J, Chen S, Wei Z, et al. HAGDetector: Heterogeneous DGA domain name detection model. Computers & Security. 2022; 120: 102803. doi: 10.1016/j.cose.2022.102803
46. Kassim M, Ismail M, Jumori K, Yusuf MI. A Survey: Bandwidth Management in a IP-based Network. International Journal of Computer and Information Engineering. 2012; 6.
47. Lakshman TV, Madhow U. Performance Analysis of Window-Based Flow Control using TCP/IP (High Performance Networking V). IEEE/ACM Transaction on Networking; 1994.
48. Lakshman TV, Madhow U, Suter B. TCP/IP performance with random loss and bidirectional congestion. IEEE/ACM Transactions on Networking. 2000; 8(5): 541-555. doi: 10.1109/90.879342
49. Lakshmanna K, Kaluri R, Gundluru N, et al. A Review on Deep Learning Techniques for IoT Data. Electronics. 2022; 11(10): 1604. doi: 10.3390/electronics11101604
50. Loshin P. IPV6 Clearly Explained, 1st ed. Morgan Kaufman, San Francisco, USA; 1999.
51. Ahakonye LAC, Nwakanma CI, Lee JM, et al. SCADA intrusion detection scheme exploiting the fusion of modified decision tree and Chi-square feature selection. Internet of Things. 2023; 21: 100676. doi: 10.1016/j.iot.2022.100676
52. Mamushiane L, Mwangama J, Lysko A. Controller placement optimization for Software Defined Wide Area Networks (SDWAN). ITU Journal on Future and Evolving Technologies. 2021; 2(1): 45-66. doi: 10.52953/puiu5171
53. Ma Q, Cao Z, Gong W, et al. BOND. ACM Transactions on Sensor Networks. 2021; 17(2): 1-21. doi: 10.1145/3439956
54. Ma Z, Zhao Q, Huang J. Optimizing bandwidth allocation for heterogeneous traffic in IoT. Peer-to-Peer Networking and Applications. 2016; 10(3): 610-621. doi: 10.1007/s12083-016-0535-3
55. Putra MAP, Putri AR, Zainudin A, et al. ACS: Accuracy-based client selection mechanism for federated industrial IoT. Internet of Things. 2023; 21: 100657. doi: 10.1016/j.iot.2022.100657
56. Martin M. IP crossroads: Migrate to IPv6 or evolve with IPV4. Packet Magazine Archives; 1998.
57. Medeiros VN, Silvestre B, Borges VCM. Multi-objective routing aware of mixed IoT traffic for low-cost wireless Backhauls. Journal of Internet Services and Applications. 2019; 10(1). doi: 10.1186/s13174-019-0108-9
58. Yao M, Gan Q, Wang X, et al. A key-insulated secure multi-server authenticated key agreement protocol for edge computing-based VANETs. Internet of Things. 2023; 21: 100679. doi: 10.1016/j.iot.2023.100679
59. Al Koutayni MR, Reis G, Stricker D. DeepEdgeSoC: End-to-end deep learning framework for edge IoT devices. Internet of Things. 2023; 21: 100665. doi: 10.1016/j.iot.2022.100665
60. Adaramola MF, Shoewu OO. Research and Applications Towards Mathematics and Computer Science. International Book Publisher; 2023.
61. Adaramola MF, Shoewu OO, Ogundare BA. (2023), Internet Data Bandwidth Optimization and Prediction in Higher Learning Institutions Using Lagrange’s Interpolation: A Case of Lagos State University of Science and Technology. Journal of Computer Engineering and Intelligent Systems. 2023; 14(4).
62. Miller MA. Implementing IPV6, 1st ed. M&T Books, New York, USA; 1998.
63. Shirvani MH, Masdari M. A Survey on Trust- based Security in Internet of Things Challenges and Issues. Journal of Internet of Things. 2023; 21:100640. doi: 10.1016/j.iot.2022.100640
64. Herabad MG. Communication-efficient semi-synchronous hierarchical federated learning with balanced training in heterogeneous IoT edge environments. Internet of Things. 2023; 21: 100642. doi: 10.1016/j.iot.2022.100642
65. Nakhlestani A, Kaveri SV, Radfar M, et al. Low-Power Area-Efficient LDO With Loop-Gain and Bandwidth Enhancement Using Non-Dominant Pole Movement Technique for IoT Applications. IEEE Transactions on Circuits and Systems II: Express Briefs. 2021; 68(2): 692-696. doi: 10.1109/tcsii.2020.3013646
66. Tekin N, Acar A, Aris A, et al. Energy consumption of on-device machine learning models for IoT intrusion detection. Internet of Things. 2023; 21: 100670. doi: 10.1016/j.iot.2022.100670
67. Oktian YE, Le TTH, Jo U, et al. Blockchain-Powered Bandwidth Trading on SDN-Enabled Edge Network. IEEE Access. 2022; 10: 114024-114039. doi: 10.1109/access.2022.3217534
68. Buckley O, Hodges D, Windle J, et al. CLICKA: Collecting and leveraging identity cues with keystroke dynamics. Computers & Security. 2022; 120: 102780. doi: 10.1016/j.cose.2022.102780
69. Said O. A bandwidth control scheme for reducing the negative impact of bottlenecks in IoT environments: Simulation and performance evaluation. Internet of Things. 2023; 21: 100682. doi: 10.1016/j.iot.2023.100682
70. Onossovski V, Terekhov A. Modern Interactive Internet Services. In: Proceedings of the 7th Conference of Open Innovations Framework Programme FRUCT 2010; 2010.
71. Orsini G, Posdorfer W, Lamersdorf W. Saving bandwidth and energy of mobile and IoT devices with link predictions. Journal of Ambient Intelligence and Humanized Computing. 2020; 12(8): 8229-8240. doi: 10.1007/s12652-020-02557-z
72. Nayak P, Swapna G. Security issues in IoT applications using certificateless aggregate signcryption schemes: An overview. Internet of Things. 2023; 21: 100641. doi: 10.1016/j.iot.2022.100641
73. Kumar P, Suresh S. DeepTransHAR: a novel clustering-based transfer learning approach for recognizing the cross-domain human activities using GRUs (Gated Recurrent Units) Networks. Internet of Things. 2023; 21: 100681. doi: 10.1016/j.iot.2023.100681
74. Pratap A, Gupta R, Nadendla VSS, et al. Bandwidth-constrained task throughput maximization in IoT-enabled 5G networks. Pervasive and Mobile Computing. 2020; 69: 101281. doi: 10.1016/j.pmcj.2020.101281
75. Sotenga PZ, Djouani K, Kurien AM. A virtual network model for gateway media access control virtualisation in Large Scale Internet of Things. Internet of Things. 2023; 21: 100668. doi: 10.1016/j.iot.2022.100668
76. Rahman MS, Islam MA, Uddin MA, et al. A survey of blockchain-based IoT eHealthcare: Applications, research issues, and challenges. Internet of Things. 2022; 19: 100551. doi: 10.1016/j.iot.2022.100551
77. Kumar R, Sharma S, Vachhani C, et al. What changed in the cyber-security after COVID-19? Computers & Security. 2022; 120: 102821. doi: 10.1016/j.cose.2022.102821
78. Ghadhbane RM, Fernandez-Mayoralas J. Performance of a Parallel Hamming Coding in Short-frame OFDM Sensor’s Network. ITU Journal on Future and Evolving Technologies. 2021; 2(1): 77-88.
79. Sharma RP, Dharavath R, Edler DR. IoFT-FIS: Internet of Farm Things Based Prediction for Crop Pest Infestation Using Optimized Fuzzy Inference System. Journal of Internet of Things. 2023; 21. doi: 10.1016/j.iot.2022.100658
80. Villalón-Fonseca R. The nature of security: A conceptual framework for integral-comprehensive modeling of IT security and cybersecurity. Computers & Security. 2022; 120: 102805. doi: 10.1016/j.cose.2022.102805
81. Herrero R. Mechanism for IPv6 adaptation in LoRa topologies. Internet of Things. 2023; 21: 100647. doi: 10.1016/j.iot.2022.100647
82. Sara Cavallero, Nicol Decarli, Giampaolo Cuozzo, et al. Terahertz networks for future Industrial Internet of Things. ITU Journal on Future and Evolving Technologies. 2023; 4(1): 196-208. doi: 10.52953/athi4610
83. Schelhaas W. Predicting Network Performance in IoT Environments Using Long Short-term Memory (LSTM). Uppsala university; 2022.
84. Wang S, Sun L, Qin S, et al. KRTunnel: DNS channel detector for mobile devices. Computers & Security. 2022; 120: 102818. doi: 10.1016/j.cose.2022.102818
85. Bozorgi SM, Golsorkhtabaramiri M, Yazdani S, et al. A smart optimizer approach for clustering protocol in UAV-assisted IoT wireless networks. Internet of Things. 2023; 21: 100683. doi: 10.1016/j.iot.2023.100683
86. Rani S, Gupta D, Herencsar N, et al. Blockchain-enabled cooperative computing strategy for resource sharing in fog networks. Internet of Things. 2023; 21: 100672. doi: 10.1016/j.iot.2022.100672
87. Yu S, Xie L, Huang Q. Inception convolutional vision transformers for plant disease identification. Internet of Things. 2023; 21: 100650. doi: 10.1016/j.iot.2022.100650
88. Shenker S, Zhang L, Clark DD. Some observations on the dynamics of a congestion control algorithm. ACM SIGCOMM Computer Communication Review. 1990; 20(5): 30-39. doi: 10.1145/381906.381931
89. Sharma S, Aparicio E. Organizational and team culture as antecedents of protection motivation among IT employees. Computers & Security. 2022; 120: 102774. doi: 10.1016/j.cose.2022.102774
90. Sinche S, Raposo D, Armando N, et al. A Survey of IoT Management Protocols and Frameworks. IEEE Communications Surveys & Tutorials. 2020; 22(2): 1168-1190. doi: 10.1109/comst.2019.2943087
91. Sobin CC. A Survey on Architecture, Protocols and Challenges in IoT. Wireless Personal Communications. 2020; 112(3): 1383-1429. doi: 10.1007/s11277-020-07108-5
92. Stoyanova M, Nikoloudakis Y, Panagiotakis S, et al. A Survey on the Internet of Things (IoT) Forensics: Challenges, Approaches, and Open Issues. IEEE Communications Surveys & Tutorials. 2020; 22(2): 1191-1221. doi: 10.1109/comst.2019.2962586
93. Subramani N, Perumal SK, Kallimani JS, et al. Controlling energy aware clustering and multihop routing protocol for IoT assisted wireless sensor networks. Concurrency and Computation: Practice and Experience. 2022; 34(21). doi: 10.1002/cpe.7106
94. Iftikhar S, Ahmad MMM, Tuli S, et al. HunterPlus: AI based energy-efficient task scheduling for cloud–fog computing environments. Internet of Things. 2023; 21: 100667. doi: 10.1016/j.iot.2022.100667
95. Iftikhar S, Gill SS, Song C, et al. AI-based fog and edge computing: A systematic review, taxonomy and future directions. Internet of Things. 2023; 21: 100674. doi: 10.1016/j.iot.2022.100674
96. Mansouri T, Sadeghi Moghadam MR, Monshizadeh F, et al. IoT Data Quality Issues and Potential Solutions: A Literature Review. The Computer Journal. 2021; 66(3): 615-625. doi: 10.1093/comjnl/bxab183
97. Mansouri T, Moghadam MRS, Monshizadeh F, Zareravasan A. IoT Data Quality Issues and Potentials Solutions: A Literature Review. Journal of Internet of Things. 2023; 66(3): 615-625.
98. Alalibo TJ, Orike S, Elechi P. Bandwidth Optimization of Wireless Networks Using Artificial Intelligence Technique. Journal of Engineering Research. 2020; 3(9): 125-130.
99. Ogletree TW, Soper ME. Upgrading and Repairing Networks, 5th ed. Que Publishing, USA; 2006.
100. Riera TS, Higuera JRB, Higuera JB, et al. A new multi-label dataset for Web attacks CAPEC classification using machine learning techniques. Computers & Security. 2022; 120: 102788. doi: 10.1016/j.cose.2022.102788
101. Vadim Nozdrin. Economic efficiency of spectrum allocation. ITU Journal on Future and Evolving Technologies. 2021; 2(1): 67-76. doi: 10.52953/mwlm5949
102. Lukaj V, Martella F, Fazio M, et al. Establishment of a trusted environment for IoT service provisioning based on X3DH-Based brokering and Federated Blockchain. Internet of Things. 2023; 21: 100686. doi: 10.1016/j.iot.2023.100686
103. Paxson V. End-to-end Internet packet dynamics. IEEE/ACM Transactions on Networking. 1999; 7(3): 277-292. doi: 10.1109/90.779192
104. Wang F, Tian Q, Zhang Q, et al. A Dynamic Bandwidth Allocation Scheme for Internet of Thing in Network-Slicing Passive Optical Networks. 2020 IEEE Computing, Communications and IoT Applications (ComComAp); 2020.
105. Wang R, Zhang B, Wu M, et al. Performance bottleneck analysis and resource optimized distribution method for IoT cloud rendering computing system in cyber-enabled applications. EURASIP Journal on Wireless Communications and Networking. 2019; 2019(1). doi: 10.1186/s13638-019-1401-9
106. Waqar Ali Aziz, Vasos Vassiliou, Taqwa Saeed, et al. On the use of intelligent metasurfaces in data centers. ITU Journal on Future and Evolving Technologies. 2023; 4(1): 102-115. doi: 10.52953/waxe8722
107. Feng W, Kandlur DD, Saha D, et al. Understanding and improving TCP performance over networks with minimum rate guarantees. IEEE/ACM Transactions on Networking. 1999; 7(2): 173-187. doi: 10.1109/90.769766
108. Wright GR, Stevens WR. TCP/IP Illustrated: The Implementation. Boston MAC, Addison Wesley; 1995.
109. Ding X, Zhang Y, Li J, et al. A feasibility study of multi-mode intelligent fusion medical data transmission technology of industrial Internet of Things combined with medical Internet of Things. Internet of Things. 2023; 21: 100689. doi: 10.1016/j.iot.2023.100689
110. Tian Y, Gao W, Zhang Q, et al. Improving long-tailed classification by disentangled variance transfer. Internet of Things. 2023; 21: 100687. doi: 10.1016/j.iot.2023.100687
111. Zhao X, Lucani DE, Shen X, et al. Reliable IoT Storage: Minimizing Bandwidth Use in Storage Without Newcomer Nodes. IEEE Communications Letters. 2018; 22(7): 1462-1465. doi: 10.1109/lcomm.2018.2831669
112. Chen Z, Cheng Z, Luo W, et al. FSMFA Efficient Task Scheduling for Cloud-Fog Multi-factor Authentication Protocol for IoT Devices. Journal of Internet of Things. 2023; 2(8).
Refbacks
- There are currently no refbacks.
Copyright (c) 2024 Michael F. Adaramola, Oluwagbemiga O. Shoewu, Mary A. Adedoyin, Emmanuel B. Balogun
License URL: https://creativecommons.org/licenses/by/4.0/
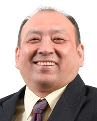
Prof. Maode Ma
Qatar University, Qatar
The field of computer and telecommunications engineering is rapidly advancing, with the following being some of the latest developments.
more
We are pleased to congratulate the first anniversiry of the journal of Computer and Telecommunication Engineering (CTE).
more
Owing to the tireless dedication of the editor-in-chief, editorial board members, and the in-house editorial team, we are proud to announce the successful online launch of the first issue of Computer and Telecommunication Engineering.
Asia Pacific Academy of Science Pte. Ltd. (APACSCI) specializes in international journal publishing. APACSCI adopts the open access publishing model and provides an important communication bridge for academic groups whose interest fields include engineering, technology, medicine, computer, mathematics, agriculture and forestry, and environment.