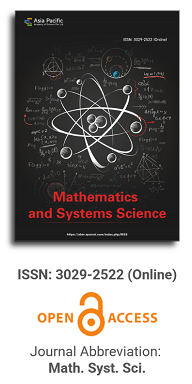
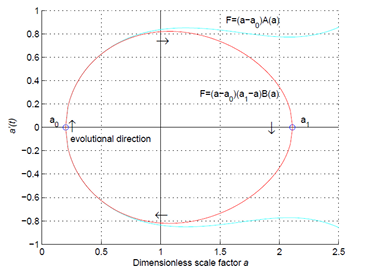
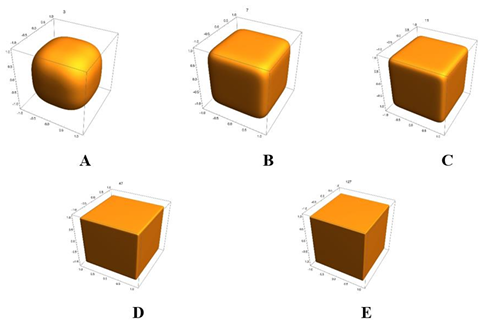
Receptivity of flow in a square lid driven cavity to implicit numerical excitations for high-fidelity HPC
Vol 3, Issue 1, 2025
Download PDF
Abstract
This study aims to investigate the effects of implicit numerical excitation on the receptivity of flow inside a square lid-driven cavity (LDC) leading to bifurcation and instability for a fixed (257 × 257) grid with different temporal resolutions via the solution of the Navier-Stokes equation. Computational results have been provided showing the flow dynamics of the LDC problem as explained with a time series at a representative point near the top corner of the cavity at (0.95, 0.95) for supercritical Reynolds numbers with respect to the bifurcation phenomenon by lowering the time step. As the accuracy of numerical methods plays a vital role in capturing the dynamics at different Reynolds numbers, this vortex-dominated flow is explained for bifurcation and instability. We propose this as a benchmark problem for the direct numerical simulation (DNS) and for machine learning (ML) of fluid flow that will lead to efficient ML algorithms and an understanding of flow receptivity, instability, and transition by DNS.
Keywords
References
- Ghia U, Ghia KN, Shin CT. High-Re solutions for incompressible flow using the Navier-Stokes equations and a multigrid method. Journal of Computational Physics. 1982; 48(3): 387–411. doi: 10.1016/0021-9991(82)90058-4
- Sengupta S, Sengupta TK, Puttam JK, et al. Global spectral analysis for convection-diffusion-reaction equation in one and two-dimensions: Effects of numerical anti-diffusion and dispersion. Journal of Computational Physics. 2020; 408. doi: 10.1016/j.jcp.2020.109310
- Sagaut P, Suman VK, Sundaram P, et al. Global spectral analysis: Review of numerical methods. Computers and Fluids. 2023; 261. doi: 10.1016/j.compfluid.2023.105915
- Sengupta TK. High Accuracy Computing Methods: Fluid Flows and Wave Phenomena. Cambridge University Press; 2013.
- Sengupta S, Sreejith NA, Mohanamuraly P, et al. Global spectral analysis of the Lax-Wendroff central difference scheme applied to convection-diffusion equation. Computers and Fluids. 2022; 242. doi: 10.1016/j.compfluid.2022.105508
- Sengupta TK, Lakshmanan V, Vijay VVSN. A new combined stable and dispersion relation preserving compact scheme for non-periodic problems. Journal of Computational Physics. 2009; 228(8). doi: 10.1016/j.jcp.2009.01.003
- Ozbenli E, Vedula P. Construction of invariant compact finite difference schemes. Physical Review E. 2020; 101. doi: 10.1103/PhysRevE.101.023303
- Sengupta TK, Ganerwal G, Dipankar A. High accuracy compact schemes and Gibbs’ phenomenon. Journal of Scientific Computing. 2004; 21(3). doi: 10.1007/s10915-004-1317-2
- Auteri F, Quartapelle L, Vigevano L. Accurate ω-ψ spectral solution of the singular driven cavity problem. Journal of Computational Physics. 2002; 180(2): 597–615. doi: 10.1006/jcph.2002.7108
- Botella O, Peyret R. Benchmark spectral results on the lid-driven cavity flow. Computers and Fluids. 1998; 27(4): 421–433. doi: 10.1016/S0045-7930(98)00002-4
- Suman VK, Viknesh SS, Tekriwal MK, et al. Grid sensitivity and role of error in computing a lid-driven cavity problem. Physical Review E. 2019; 99(1). doi: 10.1103/PhysRevE.99.013305
- Burggraf OR. Analytical and numerical studies of the structure of steady separated flows. Journal of Fluid Mechanics. 1966; 24(1): 113–151. doi: 10.1017/S0022112066000545
- Schreiber R, Keller HB. Driven cavity flows by efficient numerical techniques. Journal of Computational Physics. 1983; 49(2): 310–333. doi: 10.1016/0021-9991(83)90129-8
- Lestandi L, Bhaumik S, Avatar GRKC, et al. Multiple Hopf bifurcations and flow dynamics inside a 2D singular lid driven cavity. Computers and Fluids. 2018; 166: 86–103. doi: 10.1016/j.compfluid.2018.01.038
- Sengupta TK, Sundaram P, Suman VK, et al. A high accuracy preserving parallel algorithm for compact schemes for DNS. ACM Transactions on Parallel Computing. 2020; 7(4): 1–32. doi: 10.1145/3418073
- Sundaram P, Sengupta A, Sengupta TK. A non-overlapping high accuracy parallel subdomain closure for compact scheme: Onset of Rayleigh-Taylor instability by ultrasonic waves. Journal of Computational Physics. 2022; 470. doi: 10.1016/j.jcp.2022.111593
- Ranade R, Hill C, Pathak J. Discretization Net: A machine-learning based solver for Navier-Stokes equations using finite volume discretization. Computer Methods in Applied Mechanics and Engineering. 2021; 378: 113722. doi: 10.1016/j.cma.2021.113722
- Sahin M, Owens RG. A novel fully implicit finite volume method applied to the lid-driven cavity problem—Part I: High Reynolds number flow calculations. International Journal for Numerical Methods in Fluids. 2003; 42(1): 57–77. doi: 10.1002/fld.442
- Bruneau CH, Saad M. The 2D lid-driven cavity problem revisited. Computers and Fluids. 2006; 35(3): 326–348. doi: 10.1016/j.compfluid.2004.12.004
- Beckers M, van Heijst GJF. The observation of a triangular vortex in a rotating fluid. Fluid Dynamics Research. 1998; 22(5). doi: 10.1016/S0169-5983(97)00039-7
- Carnevale GF, Kloosterziel RC. Emergence and evolution of triangular vortices. Journal of Fluid Mechanics. 1994; 259: 305–331. doi: 10.1017/S0022112094000157
- Jansson TRN, Haspang MP, Jensen KH, et al. Polygons on a rotating fluid surface. Physical Review Letters. 2006; 96(17). doi: 10.1103/PhysRevLett.96.174502
- van der Vorst HA. Bi-CGSTAB: A fast and smoothly converging variant of Bi-CG for the solution of nonsymmetric linear systems. SIAM Journal on Scientific and Statistical Computing. 1992; 13(2). doi: 10.1137/0913035
- Landau LD, Lifshitz EM. Fluid Mechanics, 2nd ed. Elsevier; 1987.
- Brunton SL, Noack BR, Komoutsakos P. Machine learning for fluid mechanics. Annual Review of Fluid Mechanics. 2020; 52: 477–508. doi: 10.1146/annurev-fluid-010719-060214
- Jiang Q, Zhu L, Shu C, et al. Multilayer perceptron neural network activated by adaptive Gaussian radial basis function and its application to predict lid-driven cavity flow. Acta Mechanica Sinica. 2021; 37(12): 1757–1772. doi: 10.1007/s10409-021-01144-5
- Amalinadhi C, Palar PS, Stevenson R, et al. On physics-informed deep learning for solving Navier-Stokes equations. In: Proceedings of the AIAA SCITECH 2022 Forum; 3–7 January 2022; San Diego, CA, USA.
- McDevitt C, Fowler E, Roy S. Physics-constrained deep learning of incompressible cavity flow. In: Proceedings of the AIAA SCITECH 2024 Forum; 8–12 January 2024; Orlando, FL, USA.
- Sengupta TK. Transition to Turbulence: A Dynamical System Approach to Receptivity. Cambridge University Press; UK, 2021
- Sengupta TK, Vijay VVSN, Singh N. Universal instability modes in internal and external flows. Computers and Fluids. 2011; 40(1): 221–235. doi: 10.1016/j.compfluid.2010.09.006
- Eckhaus W. Studies in Non-Linear Stability Theory. In: Springer Tracts in Natural Philosophy. Springer; 1965.
- Drazin PG, Reid WH. Hydrodynamic Stability. Cambridge University Press; 2004.
- Sengupta TK. Instability of Flows and Transition to Turbulence. CRC Press; 2012
- Chu PC, Fan C. A three-point combined compact difference scheme. Journal of Computational Physics. 1998; 140(2): 370–399. doi: 10.1006/jcph.1998.5899
- Sengupta TK, Dipankar A, Sagaut P. Error dynamics: Beyond von Neumann analysis. Journal of Computational Physics. 2007; 226(2): 1211–1218. doi: 10.1016/j.jcp.2007.06.001
- Tan R, Ooi A, Sandberg RD. Two-dimensional analysis of hybrid spectral/finite difference schemes for linearized compressible Navier-Stokes equations. Journal of Scientific Computing. 2021; 87(42). doi: 10.1007/s10915-021-01442-x
- Cheviakov AF, Dorodnitsyn V, Kapstov EI. Invariant conservation law-preserving discretizations of linear and nonlinear wave equations. Journal of Mathematical Physics, vol. 61, 081504, 2020, doi: 10.1063/5.0004372
- Coppola G, Capuano F, de Luca L. Discrete energy-conservation properties in the numerical simulation of the Navier-Stokes equations. Applied Mechanics Review. 2019; 71(1). doi: 10.1115/1.4042820
- Coppola G, Capuano F, Pirozzoli S, et al. Numerically stable formulations of convective terms for turbulent compressible flows. Journal of Computational Physics. 2019; 382: 86–104. doi: 10.1016/j.jcp.2019.01.007
- Sjögreen B, Yee HC, Kotov D. Skew-symmetric splitting and stability of high order central schemes. In: Proceedings of the 11th International Conference on Numerical Modeling of Space Plasma Flows: ASTRONUM-2016; 6–10 June 2016; Monterey, CA, USA.
- Sengupta TK, Singh N, Suman VK. Dynamical system approach to instability of flow past a circular cylinder. Journal of Fluid Mechanics. 2010; 656: 82–115. doi: 10.1017/S0022112010001035
- Chomaz JM. Global instabilities in spatially developing flows: Non-normality and nonlinearity. Annual Review of Fluid Mechanics. 2005; 37: 357–392. doi: 10.1146/annurev.fluid.37.061903.175810
- Sengupta TK, Lestandi L, Haider SI, et al. Reduced order model of flows by time-scaling interpolation of DNS data. Advanced Modeling and Simulation in Engineering Sciences. 2018; 5(26). doi: 10.1186/s40323-018-0119-2
- Drakoulas GI, Goritsas TV, Bourantas GC, et al. Fast SVD-ML-ROM: A reduced order modelling framework based on machine learning for real time application. Computer Methods in Applied Mechanics and Engineering. 2023; 414: 116155. doi: 10.1016/j.cma.2023.116155
- Lam R, Sanchez-Gonzalez A, Willson M, et al., Learning skillful medium-range global weather forecasting. Science. 2023; 382(6677): 1416–1421. doi: 10.1126/science.adi23
- Kochkov D, Smith JA, Alieva A, et al., Machine learning-accelerated computational fluid dynamics. PNAS. 2021; 118(21). doi: 10.1073/pnas.2101784118
- Salim DM, Burkhart B, Sondak D. Extending a physics-informed machine-learning network for superresolution studies of Rayleigh-Benard convection. The Astrophysical Journal. 2024; 964(1). doi: 10.3847/1538-4357/ad1c55
- Takhirov A, Trenchia C, Waters J. Second order efficient nonlinear filter stabilization for high Reynolds number flows. Numerical Methods for Partial Differential Equations. 2023; 39(1): 90–107. doi: 10.1002/num.22859
- Bastian P, Altenbernd M, Dreier NA, et al. Exa-Dune-Flexible PDE solvers, numerical methods and applications. Software for Exascale Computing-SPPEXA 2016–2019. Springer; 2020. Volume 136.
- Rogallo RS. Numerical experiments in homogeneous turbulence. Available online: https://ntrs.nasa.gov/api/citations/19810022965/downloads/19810022965.pdf (accessed on 20 November 2024).
- Yeung PK, Donzis DA, Sreenivasan KR. Dissipation, enstrophy and pressure statistics in turbulence simulations at high Reynolds numbers. Journal of Fluid Mechanics. 2012; 700: 5–15. doi: 10.1017/jfm.2012.5
- Buaria D, Sreenivasan KR. Dissipation range of the energy spectrum in high Reynolds number turbulence. Physical Review of Fluids. 2020; 5(9). doi: 10.1103/PhysRevFluids.5.092601
- Buaria D, Bodenschatz E, Pumir A. Vortex stretching and enstrophy production in high Reynolds number turbulence. Physical Review Fluids. 2020; 5(10). doi: 10.1103/PhysRevFluids.5.104602
- Buaria D, Pumir A, Bodenschatz E. Self attenuation of extreme events in Navier-Stokes turbulence. Nature Communications. 2020; 11: 5852. doi: 10.1038/s41467-020-19530-1
- Orszag SA. Numerical methods for the simulation of turbulence. Physics of Fluids. 1969; 12(12). doi: 10.1063/1.1692445
- Gottlieb D, Orszag SA. Numerical Analysis of Spectral Methods: Theory and Applications. SIAM; 1977.
- Ishihara T, Gotoh T, Kaneda Y. Study of high-Reynolds number isotropic turbulence by DNS. Annual Review of Fluid Mechanics. 2009; 41: 165–180. doi: 10.1146/annurev.fluid.010908.165203
- Ishihara T, Morishita K, Yokokawa M, et al. Energy spectrum in high resolution direct numerical simulation of turbulence. Physical Review Fluids. 2016; 1(8). doi: 10.1103/PhysRevFluids.1.082403
- Doering CR, Gibbon JD. Applied Analysis of the Navier-Stokes Equations. Cambridge University Press; 1995.
- Kraichnan RH. Inertial range in two-dimensional turbulence. Physics of Fluids. 1967; 10(7): 1417–1423. doi: 10.1063/1.1762301
- Batchelor GK. Computation of the energy spectrum in homogeneous two-dimensional turbulence. Physics of Fluids. 1969; 12(12): 233–239. doi: 10.1063/1.1692443
- Brachet ME, Meneguzzi R, Politano H, et al. The dynamics of freely decaying two dimensional turbulence. Journal of Fluid Mechanics. 1988; 194: 333–349. doi: 10.1017/S0022112088003015
- Sengupta TK, Sarkar A, Joshi B, et al. Direct simulation of vortex dynamics of multicellular Taylor-Green vortex by Pseudospectral method. European Journal of Mechanics/B Fluids. 2024; 108: 226–236. doi: 10.1016/j.euromechflu.2024.08.004
- Pawar S, San O. Equation free surrogate modelling and geophysical flows at the intersection of machine learning and data assimilation. Journal of Advanced Modelling Earth System. 2022; 14(11). doi: 10.1029/2022MS003170
- Quaini A, San O, Veneziani A, et al. Bridging large eddy simulation and reduced order modelling of convection dominated flows through spatial filtering: Reviews and perspectives. Fluids. 2024; 9(8): 178. doi: 10.3390/fluids9080178
- Takhirov A, Trenchia C. Efficient nonlinear filter stabilization of the Leray-α model. Journal of Computational Physics. 2022; 471: 111668. doi: 10.1016/j.jcp.2022.111668
- Chung WT, Jung KS, Chen JH, et al. BLASTNet: A call for community-involved big data in combustion machine learning. Applications in Energy and Combustion Science. 2022; 12: 100087. doi: 10.1016/j.jaecs.2022.100087
- Lucor D, Agrawal A, Sergeant A. Simple computational strategies for more effective physics informed neural networks modeling of turbulent natural convection. Journal of Computational Physics. 2022; 456: 111022. doi: 10.1016/j.jcp.2022.111022
- Sofos F, Drikakis D, Kokkinakis IW, et al. A deep learning super-resolution model for turbulent image upscaling and its application to shock wave-boundary layer interaction. Physics of Fluids. 2024; 36(2). doi: 10.1063/5.0190272
- Zhou Y. Turbulence theories and statistical closure approaches. Physics Report. 2021; 935: 1–117. doi: 10.1016/j.physrep.2021.07.001
- Zhou Y, Clark TT, Clark DS, et al. Turbulent mixing and transition criteria of flows induced by hydrodynamic instabilities. Physics of Plasmas. 2019; 26(8). doi: 10.1063/1.5088745
- Zhou Y, Williams RJR, Ramaprabhu P, et al. Rayleigh-Taylor and Richtmeyer-Meshkov instabilities: A journey through peaks. Physica D: Nonlinear Phenomena. 2021; 423. doi: 10.1016/j.physd.2020.132832
- Joshi B, Sengupta TK, Sundaram P, et al. Highly resolved Peta-scale DNS: Onset of Kelvin-Helmholtz Rayleigh-Taylor instability via pressure pulses. Computers and Fluids. 2024; 284: 106442. doi: 10.1016/j.compfluid.2024.106442
- Zhou Y. Hydrodynamic Instabilities and Turbulence: Rayleigh-Taylor, Richtmeyer-Meshkov and Kelvin-Helmholtz Mixing. Cambridge University Press; 2024.
- Zhou Y, Saddler JD, Hurricane OA. Instabilities and mixing in inertial confinement fusion. Annual Review of Fluid Mechanics. 2025; 57: 197–225. doi: 10.1146/annurev-fluid-022824-110008
- Pereira FS, Grinstein FF, Israel D. Effect of numerical discretization schemes in shock driven turbulent mixing simulations. Computers and Fluids. 2020; 201: 104487. doi: 10.1016/j.compfluid.2020.104487
- Sengupta TK, Sharma PK, Sengupta A, et al. Tracking disturbances in transitional and turbulent flows: Coherent structures. Physics of Fluids. 2029; 31(12): 124106. doi: 10.1063/1.5130918
Refbacks
- There are currently no refbacks.
Copyright (c) 2025 Author(s)
License URL: https://creativecommons.org/licenses/by/4.0/
Editor-in-Chief
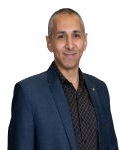
Prof. Youssri Hassan Youssri
Cairo University, Egypt
Asia Pacific Academy of Science Pte. Ltd. (APACSCI) specializes in international journal publishing. APACSCI adopts the open access publishing model and provides an important communication bridge for academic groups whose interest fields include engineering, technology, medicine, computer, mathematics, agriculture and forestry, and environment.