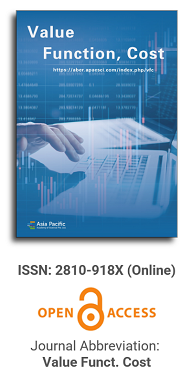
Asia Pacific Academy of Science Pte. Ltd. (APACSCI) specializes in international journal publishing. APACSCI adopts the open access publishing model and provides an important communication bridge for academic groups whose interest fields include engineering, technology, medicine, computer, mathematics, agriculture and forestry, and environment.
Dynamic Load Reactive Power Optimization Based on Convolutional Neural Network VGG16 Model
Vol 3, Issue 1, 2023
Download PDF
Abstract
In reactive power optimization of distribution network, in most cases, the processing of load data is often only for the specific data under a certain operation condition, so it is difficult to classify and process the data of multiple working conditions in a certain operation stage. To solve the above problems, an optimization method basedon convolutional neural network VGG16 (visual geometry group network) is proposed. Firstly, it is classified according to the capacity proportion of transformer in the station area, and three kinds of scenes of light, medium and high are obtained. Then, particle swarm optimization algorithm is used to optimize each kind of scene, and the corresponding optimization strategy is obtained. The effectiveness of this method to solve the problem of dynamic reactive power and voltage in the substation area is verified by simulation.
Keywords
References
- Zhang Guoyan, Jiang Lei, Zhan Wenhua, et al. Reactive Power Optimization Strategy for Distribution Networks Based on Load Curve Segmentation [J]. Science and Technology and Engineering, 2021, 21 (25): 10710-10717.
- Zhou Buxiang, Yang Jingjie, Liu Zhifan, et al. Two stage dynamic reactive power optimization of power systems based on improved state transfer algorithm [J]. Power Capacitors and Reactive Power Compensation, 2021, 42 (06): 22-30.
- Liu Aiguo, Shen Yiyi, Hou Xianguo, et al. Dynamic reactive power optimization of active distribution networks based on scenario method [J]. Guangdong Electric Power, 2021, 34 (09): 18-26.
- Wang Lianguo, Han Xiaohui, Song Lei. Reactive voltage control partitioning based on improved hybrid frog jump K-means clustering algorithm [J]. Sensors and Microsystems, 2013, 32 (06): 18-21.
- Wu Xiaomeng, Liu Xinyu, Su Zhili, et al. Dynamic reactive power optimization of distribution networks in typical scenarios based on K-means clustering [J]. Modern Electronic Technology, 2021, 44 (11): 151-154.
- Zhang Kaizhen, Li Yanwu, Liu Bo, et al. Research on hyperparameter adjustment strategies based on VGG16 networks [J]. Science and Innovation, 2021 (22): 10-13.
- Hu Junru, Dou Xiaobo, Li Chen, et al. Distributed Collaborative Reactive Power Optimization Strategy for Medium and Low Voltage Distribution Networks [J]. Power System Automation, 2021, 45 (22): 47-54.
- Wu Hao. Overview of Establishing Mathematical Models for Reactive Power Optimization in Power Systems [J]. Science and Technology Wind, 2019 (11): 187-188.
- Liao Wenlong, Yu Yan, Wang Yusen, et al. Reactive Power Optimization of Distribution Networks Based on Graph Convolutional Networks [J]. Power Grid Technology, 2021, 45 (06): 2150-2160.
- Gao Yuan, Ding Lu, Ji Jie. A novel hybrid algorithm for reactive power optimization based on genetic algorithm and artificial neural network algorithm [J]. Electronic Testing, 2020 (13): 47-49, 76.
Refbacks
- There are currently no refbacks.
Copyright (c) 2024 DUAN Jun peng, YANG Chen chen, HE Peng, XU Ke wei, LI Guo you, WANG Jia fu

This work is licensed under a Creative Commons Attribution-NonCommercial 4.0 International License.
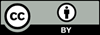
This site is licensed under a Creative Commons Attribution 4.0 International License (CC BY 4.0).
Zhibiao Gao
Nankai University, China