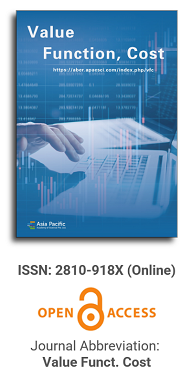
Asia Pacific Academy of Science Pte. Ltd. (APACSCI) specializes in international journal publishing. APACSCI adopts the open access publishing model and provides an important communication bridge for academic groups whose interest fields include engineering, technology, medicine, computer, mathematics, agriculture and forestry, and environment.
An Aerial Image Dehazing Algorithm Using a Prior-Based Dense Attentive Network
Vol 3, Issue 1, 2023
Download PDF
Abstract
To address the problem that the acquired images tend to degrade in clarity and fidelity in aerial hazy conditions to the extent that the target is difficult to detect, this paper proposes an aerial image dehazing algorithm using a prior-based dense attentive network. The network is based on dense blocks and attention blocks with an encoder-decoder structure, which can directly learn the mapping between the input image and the corresponding haze-free image without relying on the traditional atmospheric scattering model. In addition, to better handle inhomogeneous hazy images, the initial fuzzy density map is first extracted from the original hazy images and then used as a common input to the network together with the original hazy images. Finally, this paper synthesizes a large -scale aerial image dehazing dataset containing two subsets of uniform and non -uniform images. The experimental results and data analysis show that the proposed method exhibits better performance of dehazing with other algorithms on both synthetic and real images.
Keywords
References
1. Wang Jingdong, Zhang Wentao, Wang Zirui, Xu Lihong. A fast aerial image dehazing algorithm [J]. Journal of Aeronautics, 2013, 34 (03): 636-643.
2. Wu Di, Zhu Qingsong. Latest research progress on image dehazing [J]. Journal of Automation, 2015, 41 (2): 221-239.
3. Fattal R. Single image dehazing [J]. ACM Transactions on Graphics, 2008, 27(3): 72.
4. He K, Sun J, Tang X. Single image haze removal using dark channel prior[C]//IEEE Conference on Computer Vision and Pattern Recognition. CVPR 2009. IEEE, 2009: 1956-1963.
5. Cai B, Xu X, Jia K, et al. DehazeNet: An End-to–End System for Single Image Haze Removal [J]. IEEE Transactions on Image Processing, 2016, 25 (11): 5187-5198.
6. Ren, X., Bo, L. and Fox, D. (2012) Rgb-(d) Scene Labeling: Features and Algorithms. IEEE Conference on Computer Vision and Pattern Recognition, Providence, 2759-2766.
7. Li Yong-Fu, Cui Heng-Qi, Zhu Hao, Zhang Kai-Bi. A defogging algorithm for aerial image with improved AOD-Net. Acta Automatica Sinica, 2022, 48(6): 1543−1559.
8. Zheng, S., Jayasumana, S., Romera-Paredes, B., et al. (2015) Condi-tional Random Fields as Recurrent Neural Networks. Proceedings of the IEEE International Conference on Computer Vision, Santiago, 1529-1537.
9. Shel-hamer, E., Long, J. and Darrell, T. (2017) Fully Convolutional Networks for Semantic Segmentation. IEEE Transactions on Pattern Analysis & Machine Intelligence, 39, 640.
10. Csurka, G. and Perronnin, F. (2011) An Efficient Approach to Semantic Segmentation. International Journal of Com-puter Vision, 95, 198-212.
11. Xiao, J.X. and Quan, L. (2009) Multi-ple View Semantic Segmentation for Street View Images. International Conference on Computer Vision, Kyoto, 29 Sep-tember-2 October 2009, 686-693.
12. Shotton, J., Winn, J. and Bother, C. (2006) Textonboost: Joint Appearance, Shape and Context Modeling for Multiclass Object Recognition and Segmentation. ECCV, 1, 1-15.
13. Noh, H., Hong, S. and Han, B. (2015) Learning Deconvolution Network for Semantic Segmentation. Proceedings of the IEEE International Conference on Computer Vision, Santiago, 18 February 2015, 1520-1528.
14. Zhao Quanhua, Zhao Xuemei, Li Yu Fuzzy ISODATA high-resolution remote sensing image segmentation combined with HMRF model [J] Signal Processing, 2016, 32 (2): 157-166.
15. Li Quanwu Research on Road Background Detection Algorithm Based on K-means [J] Information and Computer (Theoretical Edition), 2020, 32 (15): 48-50.
16. Xu Xinzheng, Ding Shifei, Shi Zhongzhi, etc New theories and methods for image segmentation [J] Journal of Electronics, 2010, 38 (2): 76-82.
Refbacks
- There are currently no refbacks.
Copyright (c) 2024 ZHAO Hang

This work is licensed under a Creative Commons Attribution-NonCommercial 4.0 International License.
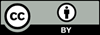
This site is licensed under a Creative Commons Attribution 4.0 International License (CC BY 4.0).
Zhibiao Gao
Nankai University, China