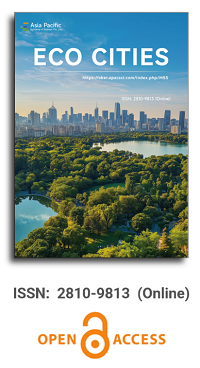
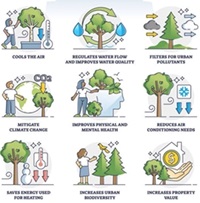
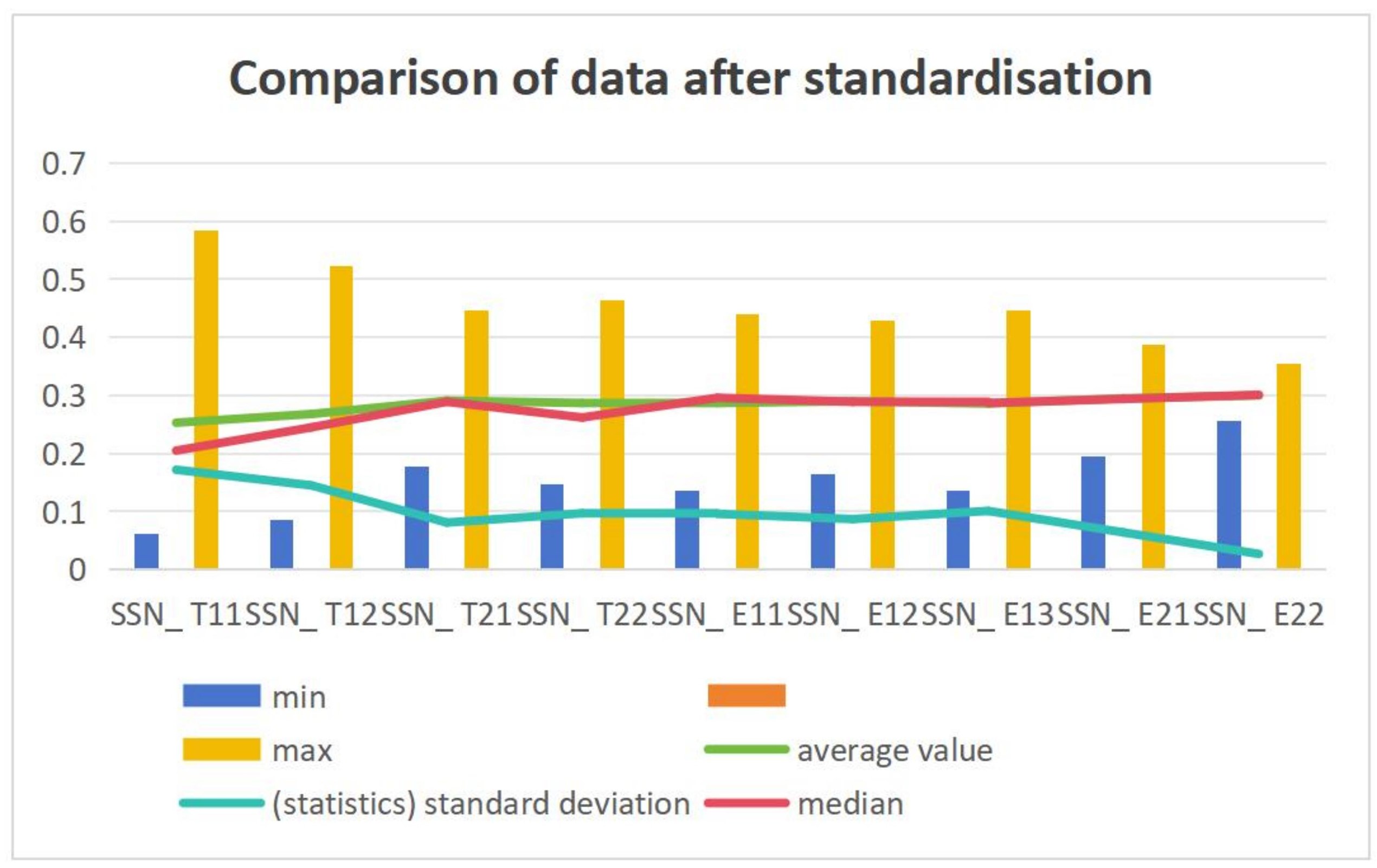
Influence of different air pollutants on concentration of PM2.5 in national capital region (NCR), India
Vol 6, Issue 1, 2025
Download PDF
Abstract
Air pollution has become a hot topic, especially among megacities throughout the globe. In this context, the national capital region (NCR) of India, including Delhi and its adjoining areas, deserves special mention. Air pollutants such as sulfur dioxide (SO2), nitrogen oxides (NOx), ground-level ozone (O3), carbon monoxide (CO), and particulate matter (PM2.5, PM10) are some of the important elements often making adverse impacts on the city air in recent times. Especially PM2.5 and PM10 in the NCR have come up several times in the news reports due to extremely high concentrations. The present article focuses on finding out the correlation between the concentration of PM2.5 and other pollutants in two regions named Indirapuram and Noida, prominent locations in the NCR. The results (R2) indicate that correlation fits better when the concentration of two pollutants is compared against PM2.5 rather than compared with a concentration of a single pollutant. In most of the cases, a positive correlation is observed, while in a few cases, a negative correlation is attained. Finally, the model is tested against some known values of independent and dependent variables.
Keywords
References
- Vaishali, Verma G, Das RM. Influence of Temperature and Relative Humidity on PM2.5 Concentration over Delhi. MAPAN. 2023; 38(3): 759-769. doi: 10.1007/s12647-023-00656-8
- Hama SML, Kumar P, Harrison RM, et al. Four-year assessment of ambient particulate matter and trace gases in the Delhi-NCR region of India. Sustainable Cities and Society. 2020; 54: 102003. doi: 10.1016/j.scs.2019.102003
- Chen Z, Li S, Liu C. Challenges and Potentials: Environmental Assessment of Particulate Matter in Spaces Under Highway Viaducts. Atmosphere. 2024; 15(11): 1325. doi: 10.3390/atmos15111325
- Selokar A, Ramachandran B, Elangovan KN, et al. PM 2.5 particulate matter and its effects in Delhi/NCR. Materials Today: Proceedings. 2020; 33: 4566-4572. doi: 10.1016/j.matpr.2020.08.187
- Kaushik N, Das RM. Investigation of NOx and related secondary pollutants at Anand Vihar, one of the most polluted area of Delhi. Urban Climate. 2023; 52: 101747. doi: 10.1016/j.uclim.2023.101747
- Goenka R, Thakur J, Taori A, et al. The prevailing smog conditions over the Delhi-NCR during the 2022 post monsoon. Advances in Space Research. 2024; 73(5): 2609-2617. doi: 10.1016/j.asr.2023.12.018
- Singh AK, Pathak AK, Saini G. Premature mortality risk and associated economic loss assessment due to PM2.5 exposure in Delhi, India during 2015–2019. Air Quality, Atmosphere & Health. 2024; 17(9): 1867-1883. doi: 10.1007/s11869-024-01550-1
- Singh V, Singh S, Biswal A. Exceedances and trends of particulate matter (PM2.5) in five Indian megacities. Science of The Total Environment. 2021; 750: 141461. doi: 10.1016/j.scitotenv.2020.141461
- Sharma SK, Mandal TK. Elemental Composition and Sources of Fine Particulate Matter (PM2.5) in Delhi, India. Bulletin of Environmental Contamination and Toxicology. 2023; 110(3). doi: 10.1007/s00128-023-03707-7
- Gupta L, Joshi S, Habib G, et al. Characteristics and atmospheric processes of water-soluble ions in PM2.5 and PM10 over an industrial city in the National Capital Region (NCR) of India. Atmospheric Environment. 2023; 312: 120020. doi: 10.1016/j.atmosenv.2023.120020
- Bera B, Bhattacharjee S, Sengupta N, et al. PM2.5 concentration prediction during COVID-19 lockdown over Kolkata metropolitan city, India using MLR and ANN models. Environmental Challenges. 2021; 4: 100155. doi: 10.1016/j.envc.2021.100155
- Gokul PR, Mathew A, Bhosale A, et al. Spatio-temporal air quality analysis and PM2.5 prediction over Hyderabad City, India using artificial intelligence techniques. Ecological Informatics. 2023; 76: 102067. doi: 10.1016/j.ecoinf.2023.102067
- Prasad NVK, Sarma MSSRKN, Sasikala P, et al. Regression Model to Analyse Air Pollutants Over a Coastal Industrial Station Visakhapatnam (India). International Journal of Data Science. 2020; 1(2): 107-113. doi: 10.18517/ijods.1.2.107-113.2020
- Central Pollution Control Board. Central Pollution Control Board. Available online: http://cpcb.nic.in/ (accessed on 10 November 2024).
- Gupta S, Sharma SK, Tiwari P, et al. Insight Study of Trace Elements in PM2.5 During Nine Years in Delhi, India: Seasonal Variation, Source Apportionment, and Health Risks Assessment. Archives of Environmental Contamination and Toxicology. 2024; 86(4): 393-409. doi: 10.1007/s00244-024-01070-0
- Chetna, Dhaka SK, Longiany G, et al. Trends and Variability of PM2.5 at Different Time Scales over Delhi: Long-term Analysis 2007–2021. Aerosol and Air Quality Research. 2023; 23(5): 220191. doi: 10.4209/aaqr.220191
- Bawase M, Sathe Y, Khandaskar H, et al. Chemical composition and source attribution of PM2.5 and PM10 in Delhi-National Capital Region (NCR) of India: results from an extensive seasonal campaign. Journal of Atmospheric Chemistry. 2021; 78(1): 35-58. doi: 10.1007/s10874-020-09412-7
- Kumar A, Singh D, Singh BP, et al. Spatial and temporal variability of surface ozone and nitrogen oxides in urban and rural ambient air of Delhi-NCR, India. Air Quality, Atmosphere & Health. 2014; 8(4): 391-399. doi: 10.1007/s11869-014-0309-0
- Mandal P, Sarkar R, Kamal N, et al. Diurnal and Seasonal Variation of Atmospheric Particulate Matter and Trace Gases in Industrial Area of Delhi: A Study. Bulletin of Environmental Contamination and Toxicology. 2022; 109(3): 488-494. doi: 10.1007/s00128-022-03572-w
- Tyagi S, Tiwari S, Mishra A, et al. Spatial variability of concentrations of gaseous pollutants across the National Capital Region of Delhi, India. Atmospheric Pollution Research. 2016; 7(5): 808-816. doi: 10.1016/j.apr.2016.04.008
- Ni XY, Huang H, Du WP. Relevance analysis and short-term prediction of PM 2.5 concentrations in Beijing based on multi-source data. Atmospheric Environment. 2017; 150: 146-161. doi: 10.1016/j.atmosenv.2016.11.054
- Cobourn WG. An enhanced PM2.5 air quality forecast model based on nonlinear regression and back-trajectory concentrations. Atmospheric Environment. 2010; 44(25): 3015-3023. doi: 10.1016/j.atmosenv.2010.05.009
Supporting Agencies
Copyright (c) 2025 Author(s)
License URL: https://creativecommons.org/licenses/by/4.0/
This site is licensed under a Creative Commons Attribution 4.0 International License (CC BY 4.0).
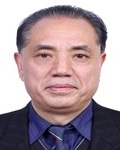
Chinese Academy of Sciences, China
Indexing & Archiving
Asia Pacific Academy of Science Pte. Ltd. (APACSCI) specializes in international journal publishing. APACSCI adopts the open access publishing model and provides an important communication bridge for academic groups whose interest fields include engineering, technology, medicine, computer, mathematics, agriculture and forestry, and environment.