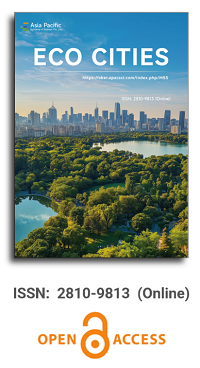
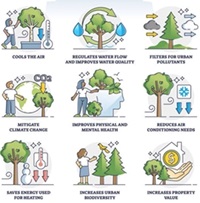
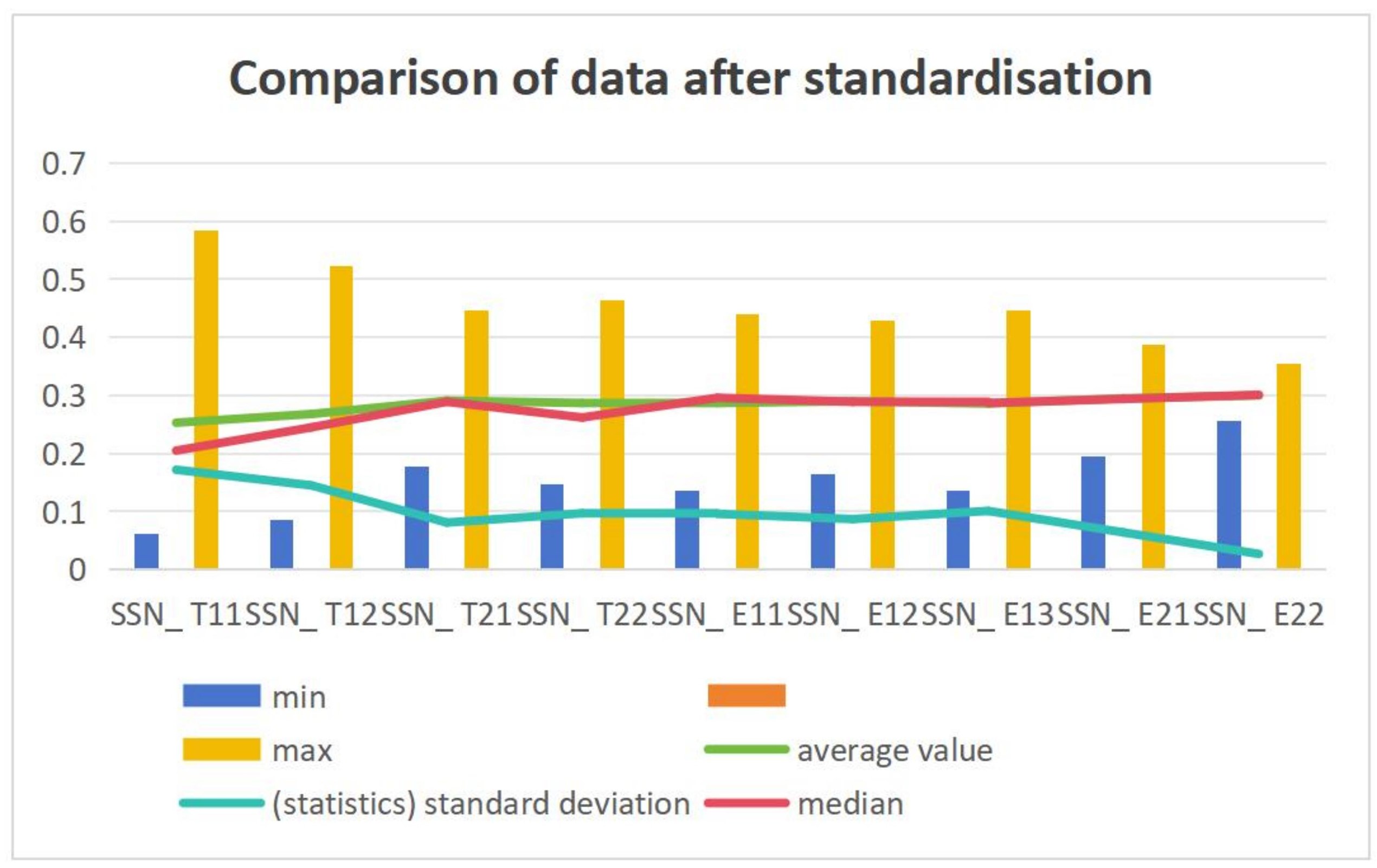
Impact of LULC spatial dynamics on incompatible mixed land use in Kaduna: A remote sensing and GIS risk analysis
Vol 5, Issue 2, 2024
Download PDF
Abstract
This study assesses the spatio-temporal changes and the impact of urbanization leading to unchecked development within Kaduna city. By utilizing satellite remote sensing data and land use maps of Kaduna, the investigation focused on how the city has expanded over the years and the resultant spurring of incompatible land uses, where residential and commercial uses are increasingly mixed with industrial zones. Using LULC analysis, the results revealed that Kaduna has experienced a 145% increase in urban area between 2001–2014, with the expansion primarily occurring in the southern part of Kaduna metropolis. A change map showing different degrees of increase and decrease in land cover classes was obtained from the post-classification comparison. Using buffer analysis, the study identified and mapped risk zones that represent areas highly susceptible to adverse effects of industrial pollution in the study area. Notably, the Kakuri industrial area has seen significant new incompatible residential and commercial developments, and areas surrounding the Kaduna Refinery and Petrochemical Company (KRPC refinery) have witnessed the proliferation of high-density residential neighborhoods such as Sabon Tasha, Maraba, and Romi. Additionally, other areas such as Mando and Western Bypass are experiencing a mixture of industrial, residential, and commercial activities. These findings underscore the need for effective urban planning and land use management to address the challenges posed by rapid urban expansion and mixed land use in Kaduna.
Keywords
References
1. Billah M, Rahman GA. Land cover mapping of Khulna city applying remote sensing technique. Bangladesh University of Engineering and Technology. 2004; 2: 46–59.
2. Cui Y, Cheng D, Choi CE, et al. The cost of rapid and haphazard urbanization: lessons learned from the Freetown landslide disaster. Landslides. 2019; 16: 1167–76.
3. Unger E-M, Zevenbergen J, Bennett R, Lemmen C. Application of LADM for disaster prone areas and communities. Land Use Policy. 2019; 80: 118–26. doi: 10.1016/j.landusepol.2018.10.012
4. Kunwar S, Ferdush J. Mapping of Land Use and Land Cover (LULC) using EuroSAT and Transfer Learning. Revue Internationale de Geomatique. 2023; 33: 1-13.
5. Agbola T. Kaduna. Cities. 1986; 3: 282–289.
6. Abolade AO, Dugeri T, Adama JU. Challenges of digitalizing land administration system in Nigeria: The Kaduna State experience. In: Proceedings of the 18th African real estate society (AFRES) annual conference; 2018. pp. 67–82.
7. Sati S, Danwalis S. Analysis of Land Use Changes in Kaduna North Local Government Area, Nigeria. Ethiopian Journal of Environmental Studies & Management. 2020; 13: 126–37.
8. Liu J, Jiao L, Zhang B, Xu G, Yang L, Dong T, et al. New indices to capture the evolution characteristics of urban expansion structure and form. Ecological Indicators. 2021; 122: 107302.
9. Kasei RA, Kalanda-Joshua MD, Benefor DT. Rapid urbanisation and implications for indigenous knowledge in early warning on flood risk in African cities. Journal of the British Academy. 2019; 7: 183–214.
10. Kafi KM, Barau AS, Aliyu A. The effects of windstorm in African medium-sized cities: An analysis of the degree of damage using KDE hotspots and EF-scale matrix. International Journal of Disaster Risk Reduction. 2021; 55: 102070.
11. Ul Din S, Mak HWL. Retrieval of land-use/land cover change (LUCC) maps and urban expansion dynamics of Hyderabad, Pakistan via Landsat datasets and support vector machine framework. Remote Sensing. 2021; 13: 3337.
12. Shi H, Zhao M, Chi B. Behind the land use mix: Measuring the functional compatibility in urban and sub-urban areas of China. Land. 2021; 11: 2.
13. Beillouin D, Cardinael R, Berre D, et al. A global overview of studies about land management, land‐use change, and climate change effects on soil organic carbon. Global Change Biology. 2022; 28: 1690–702. doi: 10.1111/gcb.15998.
14. Wu H, Shah SMA, Nawaz A, et al. Disaster management policy development and engineering economics: an analysis of game-changing impact of COVID 19 on oil-power industry, environment, and economy. Revista Argentina de Clínica Psicológica. 2020; 29: 550.
15. Jiang H, Sun Z, Guo H, Weng Q, Du W, Xing Q, et al. An assessment of urbanization sustainability in China between 1990 and 2015 using land use efficiency indicators. Npj Urban Sustainability. 2021; 1: 34.
16. Barau AS, Kafi KM, Mu’allim MA, Dallimer M, Hassan A. Comparative mapping of smellscape clusters and associated air quality in Kano City, Nigeria: An analysis of public perception, hotspots, and inclusive decision support tool. Sustainable Cities and Society. 2023; 96.
17. Sandström UG, Angelstam P, Khakee A. Urban comprehensive planning—identifying barriers for the maintenance of functional habitat networks. Landscape and Urban Planning. 2006; 75: 43–57. doi: 10.1016/j.landurbplan.2004.11.016
18. Steiner F. Frontiers in urban ecological design and planning research. Landscape and Urban Planning. 2014; 125: 304–11.
19. Zhao L, Li H, Sun Y, et al. Planning emergency shelters for urban disaster resilience: An integrated location-allocation modeling approach. Sustainability. 2017; 9: 2098.
20. Kafi KM, Shafri HZM, Shariff ABM. An analysis of LULC change detection using remotely sensed data; A Case study of Bauchi City. IOP Conf Ser: Earth Environ Sci. 2014; 20: 012056. doi: 10.1088/1755-1315/20/1/012056
21. Maddah S, Karimi S, Rezai H, Khaledi J. Detecting Land use Changes Affected by Human Activities using Remote Sensing (Case Study: Karkheh River Basin). Curr World Environ. 2015; 10: 473–81. doi: 10.12944/CWE.10.2.11
22. Panuju DR, Paull DJ, Griffin AL. Change detection techniques based on multispectral images for investigating land cover dynamics. Remote Sensing. 2020; 12: 1781.
23. Nkiruka EM, Chinedu AD, Smart UN. Landuse, landcover change dynamics and flooding in the lower Niger basin Onitsha, South Eastern Nigeria. Land Use Policy. 2023; 131: 106748. doi: 10.1016/j.landusepol.2023.106748
24. Aburas MM, Ho YM, Ramli MF, Ash’aari ZH. The simulation and prediction of spatio-temporal urban growth trends using cellular automata models: A review. International Journal of Applied Earth Observation and Geoinformation. 2016; 52: 380–9. doi: 10.1016/j.jag.2016.07.007
25. Wang J, Bretz M, Dewan MAA, Delavar MA. Machine learning in modelling land-use and land cover-change (LULCC): Current status, challenges and prospects. Science of The Total Environment. 2022; 822: 153559.
26. Hyandye CB, Worqul A, Martz LW, Muzuka ANN. The impact of future climate and land use/cover change on water resources in the Ndembera watershed and their mitigation and adaptation strategies. Environ Syst Res. 2018; 7: 7. doi: 10.1186/s40068-018-0110-4
27. Singh RK, Singh P, Drews M, Kumar P, Singh H, Gupta AK, et al. A machine learning-based classification of LANDSAT images to map land use and land cover of India. Remote Sensing Applications: Society and Environment. 2021; 24: 100624.
28. Giri C, Zhu Z, Reed B. A comparative analysis of the Global Land Cover 2000 and MODIS land cover data sets. Remote Sensing of Environment. 2005; 94: 123–32.
29. Agapiou A, Lysandrou V, Hadjimitsis DG. Earth observation contribution to cultural heritage disaster risk management: case study of Eastern Mediterranean open air archaeological monuments and sites. Remote Sensing. 2020; 12: 1330.
30. Mahamoud A, Maher G, Mohamed NA, et al. Monitoring shoreline change using remote sensing, GIS, and field surveys: a case study of the Ngazidja Island Coast, Comoros. Arab J Geosci. 2023; 16: 114. doi: 10.1007/s12517-023-11200-y
31. Roy DP, Lewis PE, Justice CO. Burned area mapping using multi-temporal moderate spatial resolution data—A bi-directional reflectance model-based expectation approach. Remote Sensing of Environment. 2002; 83: 263–86.
32. Mundia CN, Aniya M. Analysis of land use/cover changes and urban expansion of Nairobi city using remote sensing and GIS. International Journal of Remote Sensing. 2005; 26: 2831–2849. doi: 10.1080/01431160500117865
33. Piramanayagam S, Saber E, Schwartzkopf W, Koehler FW. Supervised classification of multisensor remotely sensed images using a deep learning framework. Remote Sensing. 2018; 10: 1429.
34. Ilunga JTW, Kalombo DK, Inabanza ON, et al. Contributions of Remote Sensing and GIS to the Inventory and Mapping of Colonial Geodetic Markers in the Katangese Copper Belt. RIG. 2024; 33.
35. Isa Z, Sawa BA, Abdussalam AF, et al. Impact of climate change on climate extreme indices in Kaduna River basin, Nigeria. Environ Sci Pollut Res. 2023; 30: 77689–712. doi: 10.1007/s11356-023-27821-5
36. Bununu YA, Ludin ANM, Hosni N. City profile: Kaduna. Cities. 2015; 49: 53–65.
37. Ishaya S, Abaje IB. Indigenous people’s perception on climate change and adaptation strategies in Jema’a local government area of Kaduna State, Nigeria. Journal of Geography and Regional Planning. 2008; 1: 138.
38. Al-Amin MA, Dadan-Garba A. Urban vegetation study of Kaduna Metropolis using GIS and remotely sensed data. Journal of Natural Sciences Research. 2014; 4: 160–71.
39. Bruce CM, Hilbert DW. Pre-processing methodology for application to Landsat TM/ETM+ imagery of the wet tropics. Cairns, Qld.: Rainforest CRC; 2006.
40. Yu Z, Di L, Yang R, Tang J, et al. Selection of landsat 8 OLI band combinations for land use and land cover classification. In: Proceedings of the 2019 8th International Conference on Agro-Geoinformatics (Agro-Geoinformatics); 2019. pp. 1–5.
41. Lock M. Kaduna, 1917, 1967, 2017: a survey and plan of the capital territory for the government of Northern Nigeria. (No Title). London: Faber and Faber; 1967.
42. Zhu Z. Change detection using landsat time series: A review of frequencies, preprocessing, algorithms, and applications. ISPRS Journal of Photogrammetry and Remote Sensing. 2017; 130: 370–84.
43. Yang X, Lo CP. Using a time series of satellite imagery to detect land use and land cover changes in the Atlanta, Georgia metropolitan area. International Journal of Remote Sensing. 2002; 23: 1775–98. doi: 10.1080/01431160110075802
44. Chen Z, Yang B, Wang B. A preprocessing method for hyperspectral target detection based on tensor principal component analysis. Remote Sensing. 2018; 10: 1033.
45. Barsi JA, Markham BL, Czapla-Myers JS, et al. Landsat-7 ETM+ radiometric calibration status. In: Proceedings of Spiethe International Society for Optical Engineering. NIH Public Access; 2016.
46. Chander G, Markham BL, Helder DL. Summary of current radiometric calibration coefficients for Landsat MSS, TM, ETM+, and EO-1 ALI sensors. Remote Sensing of Environment. 2009; 113: 893–903.
47. Alexandri G, Georgoulias AK, Meleti C, et al. A high resolution satellite view of surface solar radiation over the climatically sensitive region of Eastern Mediterranean. Atmospheric Research. 2017; 188: 107–21. doi: 10.1016/j.atmosres.2016.12.015
48. Jiang J, Zheng H, Ji X, et al. Analysis and evaluation of the image preprocessing process of a six-band multispectral camera mounted on an unmanned aerial vehicle for winter wheat monitoring. Sensors. 2019; 19: 747.
49. Horning N. Selecting the appropriate band combination for an RGB image using Landsat imagery. New York, USA; 2004.
50. Lillesand T, Kiefer RW, Chipman J. Remote sensing and image interpretation. John Wiley & Sons; 2015.
51. Foody GM, Mathur A, Sanchez-Hernandez C, Boyd DS. Training set size requirements for the classification of a specific class. Remote Sensing of Environment. 2006; 104: 1–14.
52. Benza M, Weeks JR, Stow DA, et al. A pattern-based definition of urban context using remote sensing and GIS. Remote Sensing of Environment. 2016; 183: 250–64. doi: 10.1016/j.rse.2016.06.011
53. Wu W, Chen WY, Yun Y, et al. Urban greenness, mixed land-use, and life satisfaction: Evidence from residential locations and workplace settings in Beijing. Landscape and Urban Planning. 2022; 224: 104428. doi: 10.1016/j.landurbplan.2022.104428
54. Momeni R, Aplin P, Boyd DS. Mapping complex urban land cover from spaceborne imagery: The influence of spatial resolution, spectral band set and classification approach. Remote Sensing. 2016; 8: 88.
55. Hilpert M. Setbacks for gas stations in a world with regionally varying emissions factors and acceptable health risks. Frontiers in Environmental Health. 2023; 2: 1214376.
56. Lewis C, Greiner LH, Brown DR. Setback distances for unconventional oil and gas development: Delphi study results. PLoS One. 2018; 13: e0202462.
57. Lü YL, Geng J, He GZ. Industrial transformation and green production to reduce environmental emissions: Taking cement industry as a case. Advances in Climate Change Research. 2015; 6: 202–9.
58. Mak HWL, Laughner JL, Fung JCH, et al. Improved satellite retrieval of tropospheric NO2 column density via updating of air mass factor (AMF): Case study of Southern China. Remote Sensing. 2018; 10: 1789.
59. Vural E. Assessment of Particle Matter Pollution during Post-Earthquake Debris Removal in Adiyaman City. Ridg. 2024; 33: 37–50. doi: 10.32604/rig.2024.047908
60. Usman MB, Sanusi YA, Musa D. Physical and commuting characteristics of selected peri-urban settlements in Kaduna, Kaduna State, Nigeria. Journal of Geography and Regional Planning. 2017; 10: 317–29.
61. Kodag S, Mani SK, Balamurugan G, Bera S. Earthquake and flood resilience through spatial Planning in the complex urban system. Progress in Disaster Science. 2022; 14: 100219. doi: 10.1016/j.pdisas.2022.100219
62. Kafi KM, Ponrahono Z, Salisu Barau A. Addressing knowledge gaps on emerging issues in weather and climate extreme events: a systematic review. Climatic Change. 2024; 177: 56. doi: 10.1007/s10584-024-03714-5
63. Tini NH, Light BJ. Impacts of Urban Sprawl on Livability in Kaduna Metropolis, Nigeria. International Journal of Scientific Research in Science and Technology. 2020; 7: 334–43.
64. Siegan BH. Land use without zoning. Rowman & Littlefield Publishers; 2020.
65. Shi Q, Cao G. Urban spillover or rural industrialisation: Which drives the growth of Beijing Metropolitan Area. Cities. 2020; 105: 102354.
66. Barau AS. Transitioning to inclusive and nature-based decarbonisaton through recreating tree-based artisanal industries in Kano City, Nigeria. Norsk Geografisk Tidsskrift-Norwegian Journal of Geography. 2023: 1–12.
67. Adeyemi AA, Ojekunle ZO. Concentrations and health risk assessment of industrial heavy metals pollution in groundwater in Ogun state, Nigeria. Scientific African. 2021; 11: e00666.
68. Emigilati MA, Ishiaku I, Usman BY, et al. Assessment of effluents discharged from textiles industries in selected villages in Kaduna State, Nigeria. African Journal of Environmental Science and Technology. 2015; 9: 385–389.
69. Chi YL, Mak HWL. From comparative and statistical assessments of liveability and health conditions of districts in Hong Kong towards future city development. Sustainability. 2021; 13: 8781.
70. Jong P, Van Beek L. Spatial planning to improve the liveability of rural areas: a case from the Netherlands. In: AESOP Annual Congress proceedings. AESOP; 2024.
Refbacks
Copyright (c) 2024 Kamil Muhammad Kafi, Suraj Ibrahim, Fatima Umar Aliyu, Mashkurah Ahmed Usman, Kemi Hamdat Olugbodi
License URL: https://creativecommons.org/licenses/by/4.0/
This site is licensed under a Creative Commons Attribution 4.0 International License (CC BY 4.0).
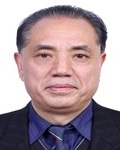
Chinese Academy of Sciences, China
Indexing & Archiving
Asia Pacific Academy of Science Pte. Ltd. (APACSCI) specializes in international journal publishing. APACSCI adopts the open access publishing model and provides an important communication bridge for academic groups whose interest fields include engineering, technology, medicine, computer, mathematics, agriculture and forestry, and environment.