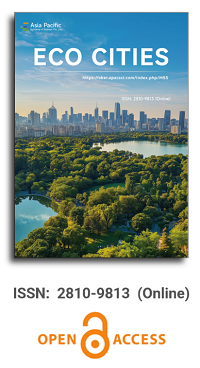
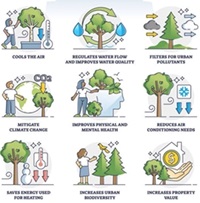
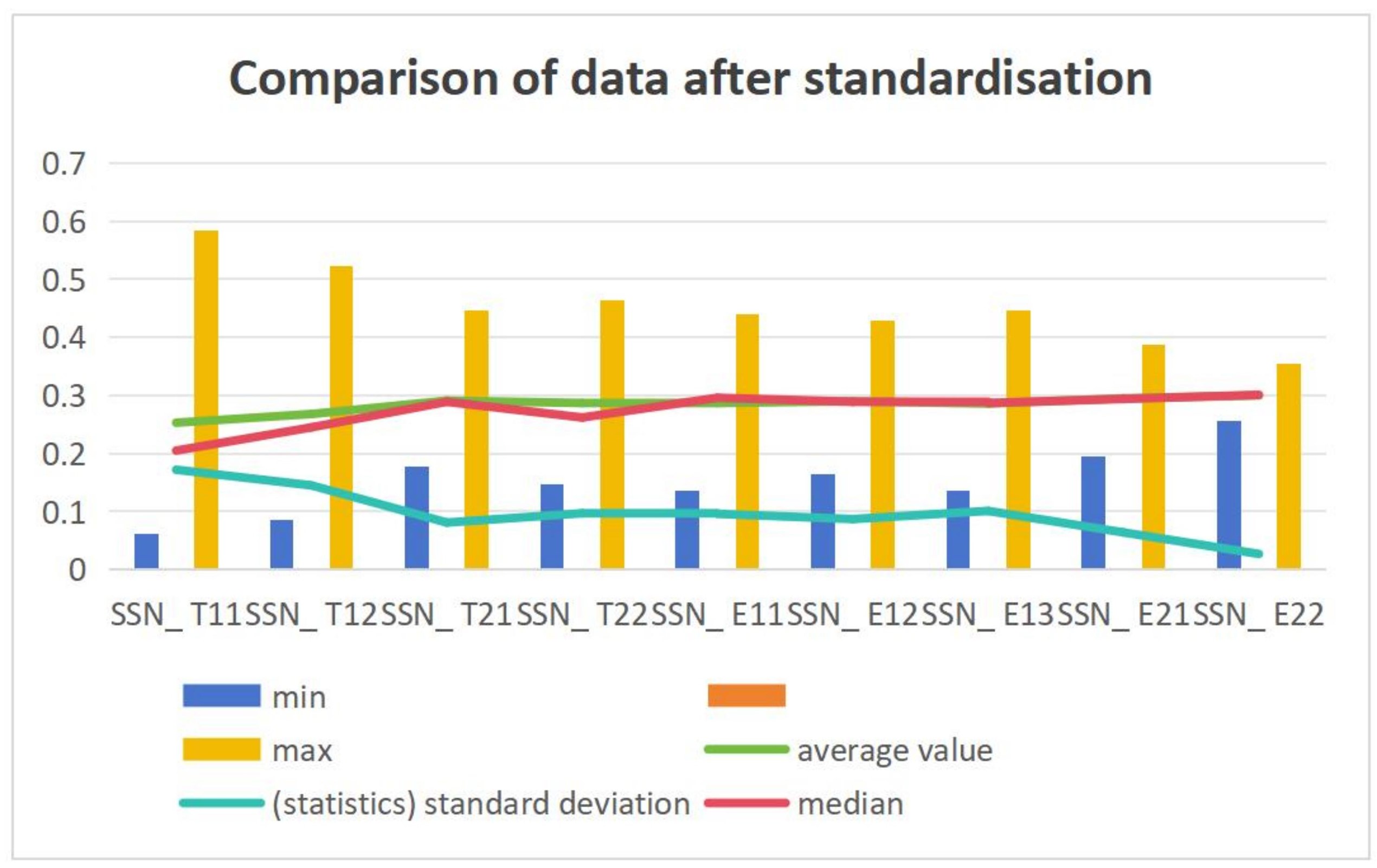
Exploring traffic accidents patterns: Spatial distribution and socio-economic determinants
Vol 4, Issue 1, 2023
Download PDF
Abstract
This study employs a machine learning methodology, specifically the decision tree algorithm, in conjunction with the Quantum Geographic Information System (QGIS), to conduct a rigorous analysis of traffic accident data. The research aims to investigate various factors associated with traffic accidents, with a particular emphasis on their spatial distribution and the socio-economic determinants contributing to recurring accidents caused by drivers. The study focuses on the city of Duhok, located in the Kurdistan Region of Iraq, and utilizes a questionnaire to collect data from drivers regarding accident locations and the frequency of accidents within the past decade (from 2010 to 2020). The findings of the study reveal that the city center experiences the highest concentration of accidents, while severe collisions tend to occur in specific “black spots” scattered across the city’s road network. The decision tree model, employed to classify drivers with multiple accidents, identifies the primary causes of accidents as traffic conditions, traffic law violations, and overspeeding. Furthermore, the accident locations are found to be influenced by various factors, including different types of road hierarchy. The age and gender of drivers also contribute to accident patterns. These research findings have practical implications for enhancing road safety measures and reducing the frequency of traffic accidents. The utilization of machine learning techniques, combined with the analysis of spatial data through QGIS, provides a comprehensive understanding of the underlying factors contributing to accidents. Moreover, this research contributes novel insights to the field of road traffic accidents and safety, particularly in the context of the city of Duhok in Kurdistan Region, Iraq, and provides a valuable reference for future studies in the domain of road safety and urban planning.
Keywords
References
- Kaparias I, Liu P, Tsakarestos A, et al. Predictive road safety impact assessment of traffic management policies and measures. Case Studies on Transport Policy 2020; 8(2): 508–516. doi: 10.1016/j.cstp.2019.11.004
- Bucsuházy K, Matuchová E, Zůvala R, et al. Human factors contributing to the road traffic accident occurrence. Transportation Research Procedia 2020; 45: 555–561. doi: 10.1016/j.trpro.2020.03.057
- Zefreh MM, Torok A. Theoretical comparison of the effects of different traffic conditions on urban road environmental external costs. Sustainability 2021; 13(6): 3541. doi: 10.3390/su13063541
- Borsos A. Application of bivariate extreme value models to describe the joint behavior of temporal and speed related surrogate measures of safety. Accident Analysis & Prevention 2021; 159: 106274. doi: 10.1016/j.aap.2021.106274
- Chand A, Jayesh S, Bhasi AB. Road traffic accidents: An overview of data sources, analysis techniques and contributing factors. Materials Today: Proceedings 2021; 47: 5135–5141. doi: 10.1016/j.matpr.2021.05.415
- McKenzie G. Spatiotemporal comparative analysis of scooter-share and bike-share usage patterns in Washington, DC. Journal of Transport Geography 2019; 78: 19–28. doi: 10.1016/j.jtrangeo.2019.05.007
- Ouni F, Belloumi M. Spatio-temporal pattern of vulnerable road user’s collisions hot spots and related risk factors for injury severity in Tunisia. Transportation Research Part F: Traffic Psychology and Behaviour 2018; 56: 477–495. doi: 10.1016/j.trf.2018.05.003
- Ouni F, Hzami M, Harizi R. Spatio-temporal hotspots analysis of pedestrian-vehicle collisions in Tunisian coastal regions. In: Proceedings of the 2020 IEEE 13th International Colloquium of Logistics and Supply Chain Management (LOGISTIQUA); 2–4 December 2020; Fez, Morocco. pp. 1–6.
- Turki Z, Ghédira A, Ouni F, Kahloul A. Spatio-temporal analysis of road traffic accidents in Tunisia. In: Proceedings of 2022 14th International Colloquium of Logistics and Supply Chain Management (LOGISTIQUA); 25–27 May 2022; El Jadida, Morocco. pp. 1–7.
- Belloumi M, Ouni F. Factors affecting the severity of motor vehicle traffic crashes in Tunisia. SAE International Journal of Transportation Safety 2019; 7(2): 99–128. doi: 10.4271/09-07-02-0006
- Harizi R, Ouni F, M’rihi R. Detection and classification of road accident black zones using exploratory spatial data techniques. International Journal of Trend in Research and Development 2006; 3(1): 173–180.
- Dereli MA, Erdogan S. A new model for determining the traffic accident black spots using GIS-aided spatial statistical methods. Transportation Research Part A: Policy and Practice 2017; 103: 106–117. doi: 10.1016/j.tra.2017.05.031
- Sun TJ, Liu SJ, Xie FK, et al. Influence of road types on road traffic accidents in northern Guizhou Province, China. Chinese Journal of Traumatology 2021; 24(1): 34–38. doi: 10.1016/j.cjtee.2020.11.002
- Kurakina E, Kravchenko P, Brylev I, Rajczyk J. Systemic approach to auditing road traffic accident black spots. Transportation Research Procedia 2020; 50: 330–336. doi: 10.1016/j.trpro.2020.10.039
- Taamneh M. Investigating the role of socio-economic factors in comprehension of traffic signs using decision tree algorithm. Journal of Safety Research 2018; 66: 121–129. doi: 10.1016/j.jsr.2018.06.002
- Clarke DD, Forsyth R, Wright R. Machine learning in road accident research: decision trees describing road accidents during cross-flow turns. Ergonomics 1998; 41(7): 1060–1079. doi: 10.1080/001401398186603
- Bordarie J. Predicting intentions to comply with speed limits using a ‘decision tree’ applied to an extended version of the theory of planned behaviour. Transportation Research Part F: Traffic Psychology and Behaviour 2019; 63: 174–185. doi: 10.1016/j.trf.2019.04.005
- da Cruz Figueira A, Pitombo CS, Meira E Silva de Oliveira PT, Larocca APC. Identification of rules induced through decision tree algorithm for detection of traffic accidents with victims: A study case from Brazil. Case Studies on Transport Policy 2017; 5(2): 200–207. doi: 10.1016/j.cstp.2017.02.004
- Abellán J, López G, de Oña J. Analysis of traffic accident severity using Decision Rules via Decision Trees. Expert Systems with Applications 2013; 40(15): 6047–6054. doi: 10.1016/j.eswa.2013.05.027
- Abdullah P, Sipos T. Drivers’ behavior and traffic accident analysis using decision tree method. Sustainability 2022; 14(18): 11339. doi: 10.3390/su141811339
- Abdullah P, Sipos T. Scenarios for mobility improvement based on individual socioeconomic factors. The Journal of Duhok University 2023; 26(1): 22–28. doi: 10.26682/sjuod.2023.26.1.3
- Sobral T, Galvão T, Borges J. Visualization of urban mobility data from intelligent transportation systems. Sensors 2019; 19(2): 332. doi: 10.3390/s19020332
- Fan Z, Liu C, Cai D, Yue S. Research on black spot identification of safety in urban traffic accidents based on machine learning method. Safety Science 2019; 118: 607–616. doi: 10.1016/j.ssci.2019.05.039
- Abdullah PH, Perschon J, Ameen AM. The relationship between car dependency and use of public transport in Duhok city-barriers analysis and recommendations. Journal of University of Duhok 2020; 23(2): 59–68. doi: 10.26682/sjuod.2020.23.2.7
Refbacks
- There are currently no refbacks.
Copyright (c) 2023 Pires Abdullah, Tibor Sipos

This work is licensed under a Creative Commons Attribution 4.0 International License.
This site is licensed under a Creative Commons Attribution 4.0 International License (CC BY 4.0).
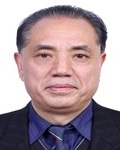
Chinese Academy of Sciences, China
Indexing & Archiving
-
-
-
-
-
-
-
- J-Gate
-
-
-
Asia Pacific Academy of Science Pte. Ltd. (APACSCI) specializes in international journal publishing. APACSCI adopts the open access publishing model and provides an important communication bridge for academic groups whose interest fields include engineering, technology, medicine, computer, mathematics, agriculture and forestry, and environment.