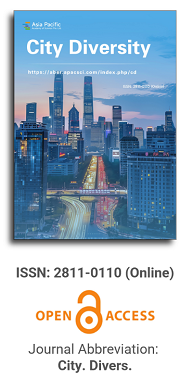
Asia Pacific Academy of Science Pte. Ltd. (APACSCI) specializes in international journal publishing. APACSCI adopts the open access publishing model and provides an important communication bridge for academic groups whose interest fields include engineering, technology, medicine, computer, mathematics, agriculture and forestry, and environment.
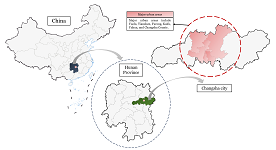
As China's pillar industry, the property market has suffered a considerable impact in recent years, with a decline in turnover and many developers at risk of bankruptcy. As one of the most concerned factors for stakeholders, housing prices need to be predicted more objectively and accurately to minimize decision-making errors by developers and consumers. Many prediction models in recent years have been unfriendly to consumers due to technical difficulties, high data demand, and varying factors affecting house prices in different regions. A uniform model across the country cannot capture local differences accurately, so this study compares and analyses the fitting effects of multiple machine learning models using February 2024 new building data in Changsha as an example, aiming to provide consumers with a simple and practical reference for prediction methods. The modeling exploration applies several regression techniques based on machine learning algorithms, such as Stepwise regression, Robust regression, Lasso regression, Ridge regression, Ordinary Least Squares (OLS) regression, Extreme Gradient Boosted regression (XGBoost), and Random Forest (RF) regression. These algorithms are used to construct forecasting models, and the best-performing model is selected by conducting a comparative analysis of the forecasting errors obtained between these models. The research found that machine learning is a practical approach to property price prediction, with least squares regression and Lasso regression providing relatively more convincing results.
Prediction of new housing prices in Changsha urban area based on multiple machine learning algorithms: A comparative analysis
Vol 5, Issue 1, 2024
Download PDF
Abstract
As China’s pillar industry, the property market has suffered a considerable impact in recent years, with a decline in turnover and many developers at risk of bankruptcy. As one of the most concerned factors for stakeholders, housing prices need to be predicted more objectively and accurately to minimize decision-making errors by developers and consumers. Many prediction models in recent years have been unfriendly to consumers due to technical difficulties, high data demand, and varying factors affecting house prices in different regions. A uniform model across the country cannot capture local differences accurately, so this study compares and analyses the fitting effects of multiple machine learning models using February 2024 new building data in Changsha as an example, aiming to provide consumers with a simple and practical reference for prediction methods. The modeling exploration applies several regression techniques based on machine learning algorithms, such as Stepwise regression, Robust regression, Lasso regression, Ridge regression, Ordinary Least Squares (OLS) regression, Extreme Gradient Boosted regression (XGBoost), and Random Forest (RF) regression. These algorithms are used to construct forecasting models, and the best-performing model is selected by conducting a comparative analysis of the forecasting errors obtained between these models. The research found that machine learning is a practical approach to property price prediction, with least squares regression and Lasso regression providing relatively more convincing results.
Keywords
References
1. Feng Y, Wahab MA, Azmi NAB, et al. Chinese Residents’ Willingness to Buy Housing: An Evaluation in Nanyang City, Henan Province, China Based on the Extension Cloud Model. Buildings. 2022; 12(10): 1695. doi: 10.3390/buildings12101695
2. National Bureau of Statistics. Statistical Bulletin of the People’s Republic of China on National Economic and Social Development, 2023. Available online: https://www.stats.gov.cn/sj/zxfb/202402/t20240228_1947915.html (accessed on 22 June 2024).
3. Li B, Li RYM, Wareewanich T. Factors Influencing Large Real Estate Companies’ Competitiveness: A Sustainable Development Perspective. Land. 2021; 10(11): 1239. doi: 10.3390/land10111239
4. Li Y, Xiang Z, Xiong T. The Behavioral Mechanism and Forecasting of Beijing Housing Prices from a Multiscale Perspective. Discrete Dynamics in Nature and Society. 2020; 2020: 1-13. doi: 10.1155/2020/5375206
5. Rico-Juan JR, Taltavull de La Paz P. Machine learning with explainability or spatial hedonics tools? An analysis of the asking prices in the housing market in Alicante, Spain. Expert Systems with Applications. 2021; 171: 114590. doi: 10.1016/j.eswa.2021.114590
6. Xu X, Zhang Y. House price forecasting with neural networks. Intelligent Systems with Applications. 2021; 12: 200052. doi: 10.1016/j.iswa.2021.200052
7. Li R, Li H. Have Housing Prices Gone with the Smelly Wind? Big Data Analysis on Landfill in Hong Kong. Sustainability. 2018; 10(2): 341. doi: 10.3390/su10020341
8. Miles D, Monro V. UK house prices and three decades of decline in the risk-free real interest rate. Economic Policy. 2021; 36(108): 627-684. doi: 10.1093/epolic/eiab006
9. Duca JV, Muellbauer J, Murphy A. What Drives House Price Cycles? International Experience and Policy Issues. Journal of Economic Literature. 2021; 59(3): 773-864. doi: 10.1257/jel.20201325
10. Barron K, Kung E, Proserpio D. The Effect of Home-Sharing on House Prices and Rents: Evidence from Airbnb. Marketing Science. 2021; 40(1): 23-47. doi: 10.1287/mksc.2020.1227
11. Bangura M, Lee CL. House price diffusion of housing submarkets in Greater Sydney. Housing Studies. 2019; 35(6): 1110-1141. doi: 10.1080/02673037.2019.1648772
12. Liu G. Research on Prediction and Analysis of Real Estate Market Based on the Multiple Linear Regression Model. Scientific Programming. 2022; 2022: 1-8. doi: 10.1155/2022/5750354
13. Madhuri CHR, Anuradha G, Pujitha MV. House Price Prediction Using Regression Techniques: A Comparative Study. In: Proceedings of the 2019 International Conference on Smart Structures and Systems (ICSSS). doi: 10.1109/icsss.2019.8882834
14. Kim J, Lee Y, Lee MH, et al. A Comparative Study of Machine Learning and Spatial Interpolation Methods for Predicting House Prices. Sustainability. 2022; 14(15): 9056. doi: 10.3390/su14159056
15. Thamarai M, Malarvizhi SP. House Price Prediction Modeling Using Machine Learning. International Journal of Information Engineering and Electronic Business. 2020; 12(2): 15-20. doi: 10.5815/ijieeb.2020.02.03
16. Qin L, Zong W, Peng K, et al. Assessing Spatial Heterogeneity in Urban Park Vitality for a Sustainable Built Environment: A Case Study of Changsha. Land. 2024; 13(4): 480. doi: 10.3390/land13040480
17. Zhou Z, Yang F, Li J, et al. Identification of Critical Areas of Openness-Vitality Intensity Imbalance in Waterfront Spaces and Prioritization of Interventions: A Case Study of Xiangjiang River in Changsha, China. Land. 2024; 13(5): 686. doi: 10.3390/land13050686
18. Anjuke. Changsha New Homes Information. Available online: https://m.anjuke.com/cs/ (accessed on 13 March 2024).
19. Li N, Li RYM, Nuttapong J. Factors affect the housing prices in China: a systematic review of papers indexed in Chinese Science Citation Database. Property Management. 2022; 40(5): 780-796. doi: 10.1108/pm-11-2020-0078
20. Liu M, Ma QP. Determinants of house prices in China: a panel-corrected regression approach. The Annals of Regional Science. 2021; 67(1): 47-72. doi: 10.1007/s00168-020-01040-z
21. Wang Z, Feng Y, Li Y, et al. Inheritance dynamics and housing price fluctuations: Evidence from the China household finance survey. Finance Research Letters. 2024; 67: 105743. doi: 10.1016/j.frl.2024.105743
22. Sun Q, Javeed SA, Tang Y, et al. The impact of housing prices and land financing on economic growth: Evidence from Chinese 277 cities at the prefecture level and above. PLOS ONE. 2024; 19(4): e0302631. doi: 10.1371/journal.pone.0302631
23. Papazafeiropoulos G. Stepwise Regression for Increasing the Predictive Accuracy of Artificial Neural Networks: Applications in Benchmark and Advanced Problems. Modelling. 2024; 5(1): 153-179. doi: 10.3390/modelling5010009
24. Ma L, Yang H, Yang J. A Multimodal Teaching Quality Evaluation for Hybrid Education Based on Stepwise Regression Analysis. Journal on special topics in mobile networks and applications/Mobile networks and applications. 2023; 1-11.
25. Arashi M, Roozbeh M, Hamzah NA, et al. Ridge regression and its applications in genetic studies. PLOS ONE. 2021; 16(4): e0245376. doi: 10.1371/journal.pone.0245376
26. Hoerl RW. Ridge Regression: A Historical Context. Technometrics. 2020; 62(4): 420-425. doi: 10.1080/00401706.2020.1742207
27. Samaniego A. CAPM-alpha estimation with robust regression vs. linear regression. Análisis Económico. 2023; 38(97): 27-37. doi: 10.24275/uam/azc/dcsh/ae/2022v38n97/samaniego
28. Gao C. Robust regression via mutivariate regression depth. Bernoulli. 2020; 26(2). doi: 10.3150/19-bej1144
29. Verardi V, Croux C. Robust Regression in Stata. SSRN Electronic Journal. 2008. doi: 10.2139/ssrn.1369144
30. Xin SJ, Khalid K. Modelling House Price Using Ridge Regression and Lasso Regression. International Journal of Engineering & Technology. 2018; 7(4.30): 498. doi: 10.14419/ijet.v7i4.30.22378
31. Roth V. The Generalized LASSO. IEEE Transactions on Neural Networks. 2004; 15(1): 16-28. doi: 10.1109/tnn.2003.809398
32. Sanchez JM. Estimating Detection Limits in Chromatography from Calibration Data: Ordinary Least Squares Regression vs. Weighted Least Squares. Separations. 2018; 5(4): 49. doi: 10.3390/separations5040049
33. Nascimento RS, Froes RES, e Silva NOC, et al. Comparison between ordinary least squares regression and weighted least squares regression in the calibration of metals present in human milk determined by ICP-OES. Talanta. 2010; 80(3): 1102-1109. doi: 10.1016/j.talanta.2009.08.043
34. Zhang X, Yan C, Gao C, et al. Predicting Missing Values in Medical Data Via XGBoost Regression. Journal of Healthcare Informatics Research. 2020; 4(4): 383-394. doi: 10.1007/s41666-020-00077-1
35. Shehadeh A, Alshboul O, Al Mamlook RE, et al. Machine learning models for predicting the residual value of heavy construction equipment: An evaluation of modified decision tree, LightGBM, and XGBoost regression. Automation in Construction. 2021; 129: 103827. doi: 10.1016/j.autcon.2021.103827
36. Iannace G, Ciaburro G, Trematerra A. Wind Turbine Noise Prediction Using Random Forest Regression. Machines. 2019; 7(4): 69. doi: 10.3390/machines7040069
37. Mendez G, Lohr S. Estimating residual variance in random forest regression. Computational Statistics & Data Analysis. 2011; 55(11): 2937-2950. doi: 10.1016/j.csda.2011.04.022
38. Yao Q, Li RYM, Song L, et al. Construction safety knowledge sharing on Twitter: A social network analysis. Safety Science. 2021; 143: 105411. doi: 10.1016/j.ssci.2021.105411
39. Daoud JI. Multicollinearity and Regression Analysis. Journal of Physics: Conference Series. 2017; 949: 012009. doi: 10.1088/1742-6596/949/1/012009
40. Tiku ML. Tables of the Power of the F-Test. Journal of the American Statistical Association. 1967; 62(318): 525. doi: 10.2307/2283980
41. Colin Cameron A, Windmeijer FAG. An R-squared measure of goodness of fit for some common nonlinear regression models. Journal of Econometrics. 1997; 77(2): 329-342. doi: 10.1016/S0304-4076(96)01818-0
42. Mao Q, Wang L, Guo Q, et al. Evaluating Cultural Ecosystem Services of Urban Residential Green Spaces from the Perspective of Residents’ Satisfaction with Green Space. Frontiers in Public Health. 2020; 8. doi: 10.3389/fpubh.2020.00226
43. Feng Q, Wang Y, Chen C, et al. Effect of Homebuyer Comment on Green Housing Purchase Intention—Mediation Role of Psychological Distance. Frontiers in Psychology. 2021; 12. doi: 10.3389/fpsyg.2021.568451
44. Guo M, Xiao S. An empirical analysis of the factors driving customers’ purchase intention of green smart home products. Frontiers in Psychology. 2023; 14. doi: 10.3389/fpsyg.2023.1272889
45. Bai S, Li F, Xie W. Green but Unpopular? Analysis on Purchase Intention of Heat Pump Water Heaters in China. Energies. 2022; 15(7): 2464. doi: 10.3390/en15072464
46. Zhao S, Chen L. Exploring Residents’ Purchase Intention of Green Housings in China: An Extended Perspective of Perceived Value. International Journal of Environmental Research and Public Health. 2021; 18(8): 4074. doi: 10.3390/ijerph18084074
47. Ma D, Lv B, Li X, et al. Heterogeneous Impacts of Policy Sentiment with Different Themes on Real Estate Market: Evidence from China. Sustainability. 2023; 15(2): 1690. doi: 10.3390/su15021690
48. Song Y, Zhang C. City size and housing purchase intention: Evidence from rural-urban migrants in China. Urban Studies. 2019; 57(9): 1866-1886. doi: 10.1177/0042098019856822
49. Zou J, Chen J, Chen Y. Hometown landholdings and rural migrants’ integration intention: The case of urban China. Land Use Policy. 2022; 121: 106307. doi: 10.1016/j.landusepol.2022.106307
50. Xiaolan Z. 160,000 old neighborhoods look forward to a ‘new look’. People’s Daily Online. 2019. Available online: https://house.people.com.cn/n1/2019/0726/c164220-31257403.html (accessed on 23 June 2024).
51. Urban Construction Division (UCD). Nationwide, 53,700 new urban old districts to be renovated by 2023. Available online: https://www.mohurd.gov.cn/xinwen/gzdt/202402/20240201_776526.html (accessed on 23 June 2024).
52. Zeng L, Li RYM, Li R. Chromaticity Analysis on Ethnic Minority Color Landscape Culture in Tibetan Area: A Semantic Differential Approach. Applied Sciences. 2024; 14(11): 4672. doi: 10.3390/app14114672
Refbacks
- There are currently no refbacks.
Copyright (c) 2024 Yin Junjia, Aidi Hizami Alias, Nuzul Azam Haron, Nabilah Abu Bakar
License URL: https://creativecommons.org/licenses/by/4.0/
This site is licensed under a Creative Commons Attribution 4.0 International License (CC BY 4.0).
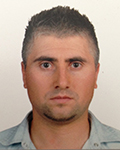
Prof. Mehmet Cetin
Kastamonu University,
Turkey