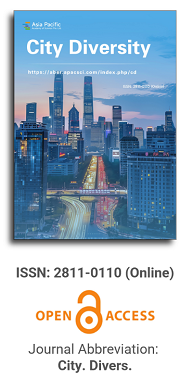
Asia Pacific Academy of Science Pte. Ltd. (APACSCI) specializes in international journal publishing. APACSCI adopts the open access publishing model and provides an important communication bridge for academic groups whose interest fields include engineering, technology, medicine, computer, mathematics, agriculture and forestry, and environment.
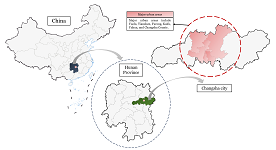
As China's pillar industry, the property market has suffered a considerable impact in recent years, with a decline in turnover and many developers at risk of bankruptcy. As one of the most concerned factors for stakeholders, housing prices need to be predicted more objectively and accurately to minimize decision-making errors by developers and consumers. Many prediction models in recent years have been unfriendly to consumers due to technical difficulties, high data demand, and varying factors affecting house prices in different regions. A uniform model across the country cannot capture local differences accurately, so this study compares and analyses the fitting effects of multiple machine learning models using February 2024 new building data in Changsha as an example, aiming to provide consumers with a simple and practical reference for prediction methods. The modeling exploration applies several regression techniques based on machine learning algorithms, such as Stepwise regression, Robust regression, Lasso regression, Ridge regression, Ordinary Least Squares (OLS) regression, Extreme Gradient Boosted regression (XGBoost), and Random Forest (RF) regression. These algorithms are used to construct forecasting models, and the best-performing model is selected by conducting a comparative analysis of the forecasting errors obtained between these models. The research found that machine learning is a practical approach to property price prediction, with least squares regression and Lasso regression providing relatively more convincing results.
Analysis of territorial patterns of age population structure at the subnational level in Chile
Vol 4, Issue 1, 2023
Download PDF
Abstract
From the evidence obtained in the 2017 Chilean population census pyramid for the country scale, the question arises whether the population structure by age is replicated homogeneously at the subnational level or, on the contrary, it presents heterogeneous local characteristics based on age groups. For each of the third-level subnational units (n = 346), the standard deviation of the different age classes was compared in relation to the national population pyramid. With these differences, the k-means method was used to identify eight groups of communes, and four demographic indicators were calculated. The results allow to affirm that the population structure by age and sex at the communal level presents heterogeneous characteristics. The analysis of territorial patterns identified groups of the aging population, households with active aging, transition to aging, adult population, men in terminal productive age, men in initial age, households with children under 15 years and households with children over 15 years.
Keywords
References
1. Amaglobeli D. Dabla-Norris E. Gaspar V. Aging without impoverishment. Finance and Development. 2020; 57: 31–34.
2. Aroca P. Atienza M. Economic implications of long distance commuting in the Chilean mining industry. Resources Policy. 2011; 36(3): 196–203. doi: 10.1016/j.resourpol.2011.03.004
3. Pino AO. Concha MG. Comparative analysis of interregional commutation in the new Biobío region (Spanish). Entramado. 2019; 16(1): 60–74. doi: 10.18041/1900-3803/entramado.1.6066
4. Blanco M. The life course approach: origins and development. Revistam de Latinoamer. 2011; 5(8): 5–31.
5. ECLAC. Demographic Observatory. Economic Commission for Latin America and the Caribbean. Santiago de Chile; 2019.
6. Coy M. Risk and vulnerability studies from human geography. Its relevance for Latin America. Población y Sociedad. 2010; 17: 9–28.
7. Di Virgilio MM. Gil y de Anso ML. Housing strategies of families from popular and middle sectors residing in the metropolitan area of Buenos Aires (Argentina). Revista de Estudios Sociales. 2012; (44): 158–170. doi: 10.7440/res44.2012.15
8. Fuenzalida M. Carvajal E. Inequalities in hospital care for diabetes mellitus in the Servicio de Salud Metropolitano Central. Chile. Revista Universidad Geográfica. 2019; 28: 91–112.
9. INE Síntesis Resultados Censo. National Institute of Statistics. Chile; 2017.
10. Jaramillo S. The role of the land market in the configuration of some socio-spatial features of Latin American cities. Territorios. 1999; 2: 107–129.
11. Sánchez GS. López AA. Review and analysis on the determinants of access to primary care services in rural and urban older adult population. ResearchGate. 2020; 19(2): 175–184.
12. León Salas B. The demographic contribution of immigration: the case of Spain. Politics and Culture. 2005; 23: 121–143.
13. Mac-Clure Ó. Calvo R. Social inequalities and types of territories in Chile (Spanish). Polis (Santiago). 2013; 12(34): 467–490. doi: 10.4067/s0718-65682013000100023
14. Manzano FA. The contribution of the geographical dimension in the analysis of Argentine labor market imbalances. Geografia em Atos. 2016; 1(3): 1–19.
15. Newbold KB. Population Geography: Tools and Issues. Rowman & Littlefield Publishers; 2017.
16. Otero Gómez MC. Consumption of children’s products at the base of the population pyramid: analysis of inf luencing mechanisms (Spanish). Económicas CUC. 2017; 38(1): 165–184. doi: 10.17981/econcuc.38.1.08
17. Peláez E. Minoldo S. Impacto del envejecimiento sobre demandas de servicios en el Cono Sur. Revista Latinoamericana de Población. 2018; (23): 62–84. doi: 10.31406/n23a4
18. Polanía MJ. Pacheco B. Rosselli D. The use of population pyramids as a graphic representation of the Colombian health system. Archivos de Medicina (Manizales). 2018; 18(1): 127–133. doi: 10.30554/archmed.18.1.2038.2018
19. Rodríguez QT. Fabelo RJR. Iglesias MS. Perceived barriers in community and health services by older adults. Revista Cubana de Salud Pública. 2017; 43: 16–26.
20. Saavedra CF. De Cea M. Teitelboim B. Chilean presidential 2017. For whom and how much indigenous people vote. Estudios Políticos. 2019; 55: 14–36.
21. Sabatini F. Rasse A. Cáceres G. et al. Real estate developers. gentrification and residential segregation in Santiago de Chile. Rev Mex Sociol. 2017; 79: 229–260.
Refbacks
- There are currently no refbacks.
Copyright (c) 2023 Manuel Fuenzalida, María Paz Trebilcock, Paulette Landon, Francisco Maturana

This work is licensed under a Creative Commons Attribution 4.0 International License.
This site is licensed under a Creative Commons Attribution 4.0 International License (CC BY 4.0).
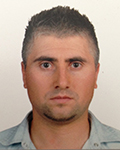
Prof. Mehmet Cetin
Kastamonu University,
Turkey