Discussion on the biodiversity protection planning methods based on urban–regional scale
Vol 2, Issue 2, 2021
VIEWS - 45 (Abstract)
Abstract
This paper explores the relationship between the spatial scale and biodiversity protection level and thinks that under the specifi c urban-region scale, landscape diversity is the key level to the macroscopic protection of biodiversity. Based on the systematicness and the otherness of urban–regional environment and the biologic fl ow and process, the protection pattern of biodiversity within urban–regional scale should be established. The author holds that seeking the “optimal landscape pattern”is one of the core missions of biodiversity protection planning. Based on that, the paper summarizes the two types of city space and their biodiversity planning and design in two ways: first, the”Urban-rural landscape pattern optimization approach”specific to the space of city matrix, which includes “agglomeration segregation”,”landscape”, “green infrastructure”, three models and five pattern optimization strategies; second, the “Nature reserve approach” specific to the space of natural matrix, which includes”reserve circle”and”protected area network”two modes and six reserve design principles.
Keywords
Full Text:
PDFReferences
1. Yu K, Li D. Landscape planning approach for biodiversity conservation. Biodiversity 1998; 6(3): 205–212.
2. Fu B, Chen L. Types of landscape diversity and their ecological significance. Journal of geography 1996; 51(5): 454–462.
3. Wang Q, Wang X, Luo J, et al Ecotone and biodiversity. Biodiversity 1997;5(2): 126–131.
4. Yu K, Huang G, Li D, et al Construction and organization of Landscape Network -- Discussion on landscape ecological planning of Shihua Cave Scenic Spot. Journal of urban planning 2005; (3): 76 – 81.
5. Fu Bojie, chen Liding, markming, et al Principles and applications of Landscape Ecology (Second Edition). Beijing: Science Press; 2011; 253–254.
6. Yu K, Wang S, Li D. Regional ecological security pattern: Beijing case. China Construction Industry Press; 2011.
7. Mark A. Benedict, edward T. Mcmahon Green infrastructure. Translated by huangliling, zhuqiang, duxiuwen, et al Beijing: China Construction Industry Press; 2010; 12–14.
8. Fu B, Chen L et al Principles and applications of Landscape Ecology (Second Edition). Beijing: Science Press; 2011; 288–289.
Refbacks
- There are currently no refbacks.
Copyright (c) 2022 Bangrui Yue, Shilei Kang, Chang Jiang

This work is licensed under a Creative Commons Attribution 4.0 International License.
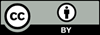
This site is licensed under a Creative Commons Attribution 4.0 International License (CC BY 4.0).