Research on the identification of myocardial infarction location based on multi-Resolution residual network
Vol 1, Issue 1, 2020
VIEWS - 26 (Abstract)
Abstract
In order to realize the classification and recognition of anterior myocardial infarction, inferior myocardial infarction, anterior septal myocardial infarction and normal ECG signals, this study takes the clinical database as the experimental data source, extracts the training set and test set data for training and testing the network model, optimizes the traditional neural network, and designs a new network algorithm: multi-resolution residual network. The multi-resolution residual network is visually compared with the traditional network to evaluate the recognition effect of the model. The test set accuracy of multi-resolution residual network is 91.8%, which is higher than that of classical neural network. The algorithm in this study can assist doctors in the diagnosis of myocardial infarction diseases, and has certain clinical significance.
Keywords
Full Text:
PDFReferences
1. Guojihong New concept electrocardiogram [m] Beijing: Peking University Medical Press, 2014
2. Liu Wenxia, Wang Sheng, Ma Yi Progress in the study of electrocardiographic localization of blood vessels causing acute inferior myocardial infarction. Armed police medicine 2018; 29 (6): 635–638
3. Lvcongmin, Tang Jianmin Clinical practical electrocardiography. Beijing: Science Press, 2016
4. Lecun Y, Bengio Y, Hinton G. Deep learning. Nature 2015; 521(7553): 436–444.
5. Lecun Y, Bottou L, Bengio Y, et al. Gradient based learning applied to document recognition. P IEEE, 1998; 86(11): 2278–2324.
6. Robinson A J. An application of recurrent nets to phone probability estimation. IEEE Transactions on Neural Networks 1994; 5 (2): 298–305.
7. Simonyan K, Zisserman A. Very deep convolutional networks for large- scale image recognition. Computer Science, 2014.
8. He K, Zhang X, Ren S, et al. Deep residual learning for image recognition. Computer Vision and patternrecognition. IEEE 2015; 770–778.
9. Wu Z, Shen C, Hengel AVD. Wider or deeper: revisiting the resnet model for visual recognition. Patternrecognition 2019; 90: 119–133.
10. Chen ZX, Xie ZF, Zhang WB, et al. resnet and model fusion for automatic spoofing detection. Proc. Interspeech 2017; 102–106.
11. Wang L, Guo S, Huang W, et al. Knowledge guided disambiguation for large scale scene classification with multi resolution cnns. IEEE Transactions on Image Processing, 2017, 26 (4): 2055–2068.
12. Xue WC, Zhao HH, Zhang LQ. Encoding multi resolution two- stream cnns for action recognition. International Conference on Neural Information Processing, 2016: 564–571.
13. Zhang Q, Zhou D, Zeng X. Heartid: a multiresolution convolutional neural network for ECG based biometric human identification in smart health applications. IEEE Access 2017: 1–1.
14. Kiranyaz S, Ince T, Gabbouj M. Real time patient specific ecgclassification by 1D convolutional neural networks. IEEE Transactions on Bio medical Engineering 2016; 63(3): 664.
15. Isin A, Ozdalili S. Cardiac arrhythmia detection using deep learning. Procedia Computer Science 2017; 120: 268–275.
16. Rajpurkar P, Hannun AY, Haghpanahi M, et al.Cardiologist level arrhythmia detection with convolutional neural networks. [2017–07–06]. http//cn. Arxiv. Org/ abs/1707.01836.
17. Ioffe S, Szegedy C. Batch normalization: accelerating deep network training by reducing internal covariate shift. Arxiv preprint arxiv: 1502.03167, 2015.
18. Nair V, Hinton GE. Rectified linear units improve restricted boltzmann machines. Proceedings of the 27th International Conference on Machine Learning (ICML 10). Haifa 2010; 807–814.
19. Simonyan K, Zisserman A. Very deep convolutional networks for large scale image recognition. Arxiv preprint arxiv 1409.1556, 2014.
Refbacks
- There are currently no refbacks.
Copyright (c) 2020 Ji Qi, Hua Jiang, Ruiqing Zhang, Yang Shen, Yanni Tong, Xianzheng Sha, Shijie Chang

This work is licensed under a Creative Commons Attribution 4.0 International License.
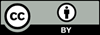
This site is licensed under a Creative Commons Attribution 4.0 International License (CC BY 4.0).
eISSN
2811-0099
Publication Frequency
Semi-annual