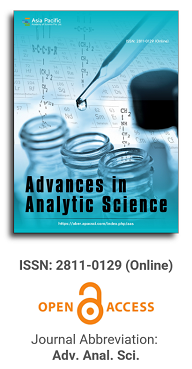
Asia Pacific Academy of Science Pte. Ltd. (APACSCI) specializes in international journal publishing. APACSCI adopts the open access publishing model and provides an important communication bridge for academic groups whose interest fields include engineering, technology, medicine, computer, mathematics, agriculture and forestry, and environment.
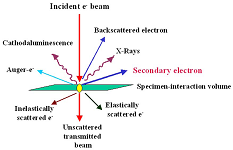
Multi-layer perceptron artificial neural network for environmental risks prediction of SW-induced pollution in Dar es Salaam, Tanzania
Vol 5, Issue 2, 2024
Download PDF
Abstract
Many metropolitan areas face significant environmental challenges posed by improper disposal and management of solid waste. As a result, environmental risks have emerged as a pressing concern, prompting dedicated research efforts. This study on environmental risk prediction of Dar es Salaam SW coincides with a mounting governmental effort over rising pollution levels from inadequate SW management. Using the multi-layer perceptron artificial neural network (MLP-ANN) model, it effectively examines the prevailing conditions and forecasts waste generation rates (WGRs) and environmental risk index (ERI) associated with SW pollution. As confirmed with 94.5% prediction accuracy and 86.5% success rate of the MLP-ANN model, WGRs in Dar es Salaam have doubled in less than two decades. Besides, over 40% of the overall generated SW is left unattended. Consequently, the ERI exhibits a consistent upward trajectory throughout the assessment period, with intermittent fluctuations between Level II and III but a persistent overall increase. Projections indicate an escalation of ERI to Level IV by 2025/26 and to a critical threshold (Level V) by 2038. The key indices such as pressure, state, and impact are anticipated to reach critical thresholds ahead of the comprehensive ERI. This underscores the imperative for timely interventions and the urgency of addressing SW management issues to curb the escalating environmental risks in Dar es Salaam and other metropolises with similar challenges.
Keywords
References
- Nabegu AB. An analysis of municipal solid waste in Kano metropolis, Nigeria. Journal of Human Ecology. 2010; 31(2): 111–119. doi:10.1080/09709274.2010.11906301
- Kazuva E, Zhang J, Tong Z, et al. The DPSIR model for environmental risk assessment of municipal solid waste in Dar es Salaam city, Tanzania. International journal of environmental research and public health. 2018; 15(8): 1692. doi: 10.3390/ijerph15081692
- Kazuva E, Zhang J, Tong Z, et al. GIS-and MCD-based suitability assessment for optimized location of solid waste landfills in Dar es Salaam, Tanzania. 2021; 28: 11259–11278. doi: 10.1007/s11356-020-11213-0
- Kazuva E. Determinants of Individuals’ willingness to use Economic Instruments foe Solid waste Management in Dar es Salaam. International Journal of Environmental Science and Natural Resources. 2017; 4(4). doi: 10.19080/IJESNR.2017.04.555644
- Kazuva E, Zhang J. Analyzing municipal solid waste treatment scenarios in rapidly urbanizing cities in developing countries: The case of Dar es Salaam, Tanzania. International Journal of Environmental Research and Public Health. 2019; 16(11): 2035. doi:10.3390/ijerph16112035
- Siddiqua A, Hahladakis JN, Al-Attiya WAK. An overview of the environmental pollution and health effects associated with waste landfilling and open dumping. Environmental Science Pollution Research. 2022; 29(39): 58514–58536. doi: 10.1007/s11356-022-21578-z
- Ajibade FO, Adelodun B, Lasisi KH, et al. Environmental pollution and their socioeconomic impacts. Woodhead Publishing Series in Food Science, Technology and Nutrition. 2021; E 321–354. doi: 10.1016/B978-0-12-821199-1.00025-0
- Boadi KO, Kuitunen M. Environmental and health impacts of household solid waste handling and disposal practices in third world cities: The case of the Accra Metropolitan Area, Ghana. Journal of Environmental Health. 2005; 68(4): 32–37.
- Andeobu L, Wibowo S, Grandhi S. Artificial intelligence applications for sustainable solid waste management practices in Australia: A systematic review. Science of The Total Environment. 2022; 834: 155389. doi: 10.1016/j.scitotenv.2022.155389
- Shams SR, Kalantary S, Jahani A, et al. Assessing the effectiveness of artificial neural networks (ANN) and multiple linear regressions (MLR) in forecasting AQI and PM10 and evaluating health impacts through AirQ+ (case study: Tehran). Environmental Pollution. 2023; 338: 122623. doi: 10.1016/j.envpol.2023.122623
- Sahour S, Khanbeyki M, Gholami V, et al. Particle swarm and grey wolf optimization: Enhancing groundwater quality models through artificial neural networks. Stochastic Environmental Research and Risk Assessment. 2024; 38(3): 993–1007.
- Azid A, Juahir H, Toriman ME, et al. Prediction of the level of air pollution using principal component analysis and artificial neural network techniques: A case study in Malaysia. Water, Air, Soil Pollution. 2014; 225: 1–14.
- Moradi MH, Sohani A, Zabihigivi M, et al. Machine learning and artificial intelligence application in land pollution research. Current Trends Advances in Computer-Aided Intelligent Environmental Data Engineering. 2022; 273–296. doi: 10.1016/B978-0-323-85597-6.00008-2
- Olawoyin R. Application of backpropagation artificial neural network prediction model for the PAH bioremediation of polluted soil. Chemosphere. 2016; 161: 145–150. doi: 10.1016/j.chemosphere.2016.07.003
- Kristian S, Hansen SF. Environmental risk assessment of chemicals and nanomaterials—the best foundation for regulatory decision-making? Science of the Total Environment. 2016; 541: 784–794. doi: 10.1016/j.scitotenv.2015.09.112
- Quinlan RJ. Human parental effort and environmental risk. Proceedings of the Royal Society B: Biological Sciences. 2007; 274(1606): 121–125. doi: 10.1098/rspb.2006.3690
- Lindell MK, Perry RW. Communicating environmental risk in multiethnic communities. Sage Publications; 2023. Volume 7.
- Rand GM. Fundamentals of aquatic toxicology: Effects, environmental fate and risk assessment. CRC press; 1995.
- Van der Oost R, Beyer J, Vermeulen NP. Fish bioaccumulation and biomarkers in environmental risk assessment: A review. Environmental Toxicology and Pharmacology. 2003; 13(2): 57–149. doi: 10.1016/S1382-6689(02)00126-6
- McRoberts DB, Quiring SM, Guikema SD. Improving hurricane power outage prediction models through the inclusion of local environmental factors. Risk Analysis. 2018; 38(12): 2722–2737. doi:10.1111/risa.12728
- Abbasi M, El Hanandeh A. Forecasting municipal solid waste generation using artificial intelligence modelling approaches. Waste Management. 2016; 56: 13–22. doi: 10.1016/j.wasman.2016.05.018
- Al-Khatib IA, Fkhidah IA, Khatib JI, et al. Implementation of a multi-variable regression analysis in the assessment of the generation rate and composition of hospital solid waste for the design of a sustainable management system in developing countries. Waste Management & Research. 2016; 34(3): 225–234. doi: 10.1177/0734242X15622813
- Thanh NP, Matsui Y, Fujiwara T. Household solid waste generation and characteristics in a Mekong Delta city, Vietnam. Journal of Environmental Management. 2010; 91(11): 2307–2321. doi: 10.1016/j.jenvman.2010.06.016
- Abbasi M, Rastgoo MN, Nakisa B. Monthly and seasonal modeling of municipal waste generation using radial basis function neural network. Environmental Progress & Sustainable Energy. 2019; 38(3): e13033. doi: 10.1002/ep.13033
- UN. World population review: Urbanization prospect, United Nations-Population Division. Available online: http://worldpopulationreview.com/world-cities/dar-es-salaam-population/#sources (accessed on 27 February 2024).
- Anande DM, Luhunga PM. Assessment of socio-economic impacts of the December 2011 flood event in Dar es Salaam, Tanzania. Atmospheric Climate Sciences. 2019; 9(03): 421. doi: 10.4236/acs.2019.93029
- Linster M, Fletcher L. Using the Pressure-State-Response Model to Develop Indicators of Sustainability. Available online: https://destinet.eu/resources/...-various-target-groups/individual-puplications/OECD_P-S-R_indicator_model.pdf/download/de/1/OECD_P-S-R_indicator_model.pdf (accessed on 2 February 2024).
- USEPA. Solid Waste and Emergency Response—Full Cost Accounting for Municipal Solid Waste Management: A Handbook. USEPA; 1997.
- Xie H, Zhang X. The measure and Countermeasure of ecological security in the suburban area in Beijing city of Haidian district as an example. China Population Resources and Environment. 2004; 14(3): 23–26.
- Zebardast L, Salehi E, Afrasiabi H. Application of DPSIR Framework for Integrated Environmental Assessment of Urban Areas: A Case Study of Tehran. International Journal of Environmental Research. 2015; 9(2): 445–456.
- Liu Y, Sun Y, Hao M, et al. Establishment of Assessment Indicator System for Environment-friendly Society Based on DPSIR Model. Environmental Protection Science. 2014.
- Bottero M, Ferretti V. Integrating the analytic network process (ANP) and the driving force-pressure-state-impact-responses (DPSIR) model for the sustainability assessment of territorial transformations. Management of Environmental Quality. 2010; 21(5): 618–644. doi: 10.1108/14777831011067926
- Elliott M. The role of the DPSIR approach and conceptual models in marine environmental management: An example for offshore wind power. Marine Pollution Bulletin. 2002; 44(6): iii–vii. doi: 10.1016/S0025-326X(02)00146-7
- Shao C, Ju M, Zhang Y, Li Z. Eco-environment security assessment study for the Binhai New Area, Tianjin, based on DPSIR model. Journal of Safety & Environment. 2008.
- Chen M, Xu L, Liu T, Huang H. The Ecosystem Health Assessment of Ganjiang River upstream frame model based on PSR. Journal of Jiangxi Agricultural University. 2012. 34(4): 839–845.
- Popescu M-C, Balas VE, Perescu-Popescu L, et al. Multilayer perceptron and neural networks. WSEAS Transactions on Circuits and Systems. 2009; 8(7): 579–588.
- Chen YC. Effects of urbanization on municipal solid waste composition. Waste Management. 2018; 79: 828–836. doi: 10.1016/j.wasman.2018.04.017
- Patel AK, Bundela VS. Quantification and prediction of solid waste generation based on socio-economical parameters. Journal of Material Cycles and Waste Management. 2024; 1–19.
- Teshome YM, Habtu NG, Molla MB, et al. Municipal solid wastes quantification and model forecasting. Global Journal of Environmental Science and Management. 2023; 9(2): 227. doi:10.22034/GJESM.2023.02.04
- Beigl P, Lebersorger S, Salhofer S. Modelling municipal solid waste generation: A review. Waste management. 2008; 28(1): 200–214. doi: 10.1016/j.wasman.2006.12.011
- Wei J, Chen H. Determining the number of factors in approximate factor models by twice K-fold cross-validation. Economics Letters. 2020; 191: 109149. doi: 10.1016/j.econlet.2020.109149
- Chicco D, Warrens MJ, Jurman G. The coefficient of determination R-squared is more informative than SMAPE, MAE, MAPE, MSE and RMSE in regression analysis evaluation. Peerj computer science. 2021; 7: e623. doi: 10.7717/peerj-cs.623
- Karunasingha DSK. Root mean square error or Mean absolute error? Use their ratio as well. Information Sciences. 2022; 585: 609–629. doi: 10.1016/j.ins.2021.11.036
- Dursun B, Fatih A, Metin Z, et al. Modeling and estimating of load demand of electricity generated from hydroelectric power plants in Turkey using machine learning methods. Advances in Electrical and Computer Engineering. 2014; 14(1): 121–132.
- Wolpert DH, Macready WG. Coevolutionary free lunches. IEEE Transactions on evolutionary computation. 2005; 9(6): 721–735. doi: 10.1109/TEVC.2005.856205
- Sentosa I, Rashid AZA, Hizam SM, et al. An empirical study on the internet usage among young creative entrepreneurs in Malaysia: A structural equation modeling approach. Business, Computer Science. 2017; 7: 447–456.
- URT. 2013–2015 National Population Projections in National Bureau of Statistics (NBS)—Ministry of Finance and Planning. Available online: https://www.nbs.go.tz/nbs/takwimu/census2012/Projection-Report-20132035.pdf (accessed on 25 June 2023).
- Flott LW. Using the scatter diagram tool to compare data, show relationship. Metal Finishing. 2012; 8(110): 33–35. doi: 10.1016/S0026-0576(13)70148-X
- Malav LC, Yadav KK, Gupta N, et al. A review on municipal solid waste as a renewable source for waste-to-energy project in India: Current practices, challenges, and future opportunities. Journal of Cleaner Production. 2020; 277: 123227. doi: 10.1016/j.jclepro.2020.123227
- Hossain MS, Santhanam A, Norulaini NAN, et al. Clinical solid waste management practices and its impact on human health and environment–A review. Waste Management. 2011; 31(4): 754–766. doi: 10.1016/j.wasman.2010.11.008
Refbacks
- There are currently no refbacks.
Copyright (c) 2024 Emmanuel Kazuva
License URL: https://creativecommons.org/licenses/by/4.0/
This site is licensed under a Creative Commons Attribution 4.0 International License (CC BY 4.0).
1.jpg)
Prof. Sivanesan Subramanian
Anna University, India