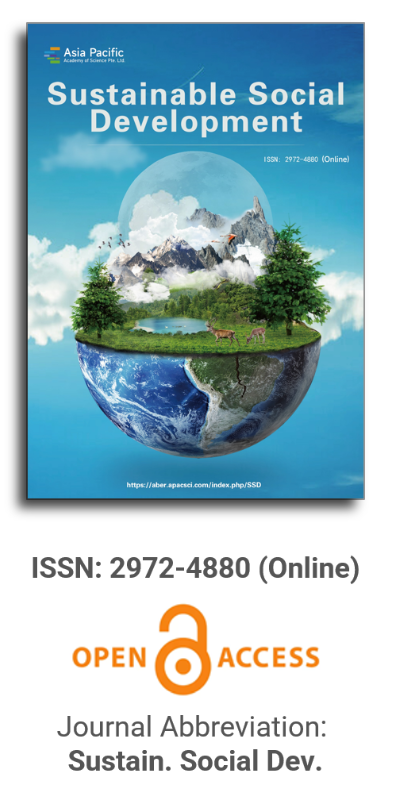
Asia Pacific Academy of Science Pte. Ltd. (APACSCI) specializes in international journal publishing. APACSCI adopts the open access publishing model and provides an important communication bridge for academic groups whose interest fields include engineering, technology, medicine, computer, mathematics, agriculture and forestry, and environment.
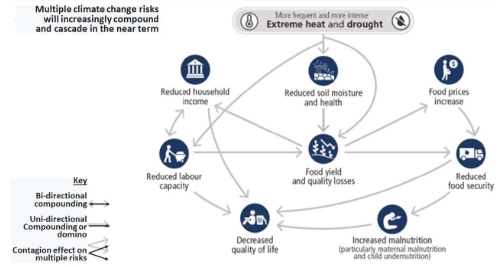
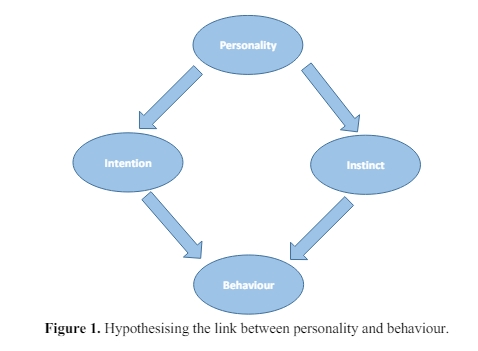
A survey of sustainable development of intelligent transportation system based on urban travel demand
Vol 2, Issue 1, 2024
Issue release: 28 February 2024
VIEWS - 4666 (Abstract)
Download PDF
Abstract
This paper provides a comprehensive exploration of urban travel demand forecasting and its implications for intelligent transportation systems, emphasizing the crucial role of intelligent transportation systems in promoting sustainable urban development. With the increasing challenges posed by traffic congestion, environmental pollution, and diverse travel needs, accurate prediction of urban travel demand becomes essential for optimizing transportation systems, fostering sustainable travel methods, and creating opportunities for business development. However, achieving this goal involves overcoming challenges such as data collection and processing, privacy protection, and information security. To address these challenges, the paper proposes a set of strategic measures, including advancing intelligent transportation technology, integrating intelligent transportation systems with urban planning, enforcing policy guidance and market supervision, promoting sustainable travel methods, and adopting intelligent transportation technology and green energy solutions. Additionally, the study highlights the role of intelligent transportation systems in mitigating traffic congestion and environmental impact through intelligent road condition monitoring, prediction, and traffic optimization. Looking ahead, the paper foresees an increasingly pivotal role for intelligent transportation systems in the future, leveraging advancements in deep learning and information technology to more accurately collect and analyze urban travel-related data for better predictive modeling. By combining data analysis, public transportation promotion, shared travel modes, intelligent transportation technology, and green energy adoption, cities can build more efficient, environmentally friendly transportation systems, enhancing residents’ travel experiences while reducing congestion and pollution to promote sustainable urban development. Furthermore, the study anticipates that intelligent transportation systems will be intricately integrated with urban public services and management, facilitating efficient and coordinated urban functions. Ultimately, the paper envisions intelligent transportation systems playing a vital role in supporting urban traffic management and enhancing the overall well-being of urban construction and residents’ lives. In conclusion, this research not only enhances our understanding of urban travel demand forecasting and the evolving landscape of intelligent transportation systems but also provides valuable insights for future research and practical applications in related fields. The study encourages greater attention and investment from scholars and practitioners in the research and practice of intelligent transportation systems to collectively advance the progress of urban transportation and sustainable development.
Keywords
References
1. Xu Z, Lv Z, Chu B, et al. Fast autoregressive tensor decomposition for online real-time traffic flow prediction. Knowledge-Based Systems. 2023, 282: 111125. doi: 10.1016/j.knosys.2023.111125
2. Sun H, Lv Z, Li J, et al. Prediction of Cancellation Probability of Online Car-Hailing Orders Based on Multi-source Heterogeneous Data Fusion. In: Wang L, Segal M, Chen J, Qiu T (editors). Wireless Algorithms, Systems, and Applications, Proceedings of the 17th International Conference, WASA 2022; 24–26 November 2022; Dalian, China. Springer; 2022. Volume 13472. pp. 168–180. doi: 10.1007/978-3-031-19214-2_14
3. Lv Z, Li J, Dong C, et al. Deep learning in the COVID-19 epidemic: A deep model for urban traffic revitalization index. Data & Knowledge Engineering. 2021, 135: 101912. doi: 10.1016/j.datak.2021.101912
4. Chen J, Chen Y. The study on the influence of highway transportation on regional economic development. In: Striełkowski W, Black JM, Butterfield SA, et al. (editors). Advances in Social Science, Education and Humanities Research, Proceedings of the 5th International Education, Economics, Social Science, Arts, Sports and Management Engineering Conference (IEESASM 2017); 28–29 December 2017; Qingdao, China. Volume 179. pp. 360–364. doi: 10.2991/ieesasm-17.2018.74
5. Xu Z, Lv Z, Li J, et al. A Novel Perspective on Travel Demand Prediction Considering Natural Environmental and Socioeconomic Factors. IEEE Intelligent Transportation Systems Magazine. 2023, 15(1): 136-159. doi: 10.1109/mits.2022.3162901
6. Sheng Z, Lv Z, Li J, et al. Deep spatial-temporal travel time prediction model based on trajectory feature. Computers and Electrical Engineering. 2023, 110: 108868. doi: 10.1016/j.compeleceng.2023.108868
7. Li H, Li J, Lv Z, Xu Z. MFAGCN: Multi-feature based attention graph convolutional network for traffic prediction. In: Liu Z, Wu F, Das SK (editors). Wireless Algorithms, Systems, and Applications, Proceedings of the 16th International Conference, WASA 2021; 25–27 June 2021; Nanjing, China. Springer; 2021. Volume 12937. pp. 227–239. doi: 10.1007/978-3-030-85928-2_18
8. Li H, Lv Z, Li J, et al. Traffic Flow Forecasting in the COVID-19: A Deep Spatial-temporal Model Based on Discrete Wavelet Transformation. ACM Transactions on Knowledge Discovery from Data. 2023, 17(5): 1-28. doi: 10.1145/3564753
9. Xu L, Pan S, Xia L, et al. Molecular Property Prediction by Combining LSTM and GAT. Biomolecules. 2023, 13(3): 503. doi: 10.3390/biom13030503
10. Lv Z, Li J, Li H, et al. Blind Travel Prediction Based on Obstacle Avoidance in Indoor Scene. Wireless Communications and Mobile Computing. 2021, 2021: 1-14. doi: 10.1155/2021/5536386
11. Sheng Z, Lv Z, Li J, et al. Taxi travel time prediction based on fusion of traffic condition features. Computers and Electrical Engineering. 2023, 105: 108530. doi: 10.1016/j.compeleceng.2022.108530
12. Xu Z, Li J, Lv Z, et al. A graph spatial-temporal model for predicting population density of key areas. Computers & Electrical Engineering. 2021, 93: 107235. doi: 10.1016/j.compeleceng.2021.107235
13. Lv Z, Cheng Z, Li J, et al. TreeCN: Time Series Prediction with the Tree Convolutional Network for Traffic Prediction. IEEE Transactions on Intelligent Transportation Systems. 2023, 1(1): 1-16. doi: 10.1109/TITS.2023.3325817
14. Xu Z, Li J, Lv Z, et al. A classification method for urban functional regions based on the transfer rate of empty cars. IET Intelligent Transport Systems. 2021, 16(2): 133-147. doi: 10.1049/itr2.12134
15. Lv Z, Li J, Dong C, et al. DeepPTP: A Deep Pedestrian Trajectory Prediction Model for Traffic Intersection. KSII Transactions on Internet & Information Systems. 2021, 15(7). doi: 10.3837/tiis.2021.07.002
16. Yuan H, Li G. A Survey of Traffic Prediction: from Spatio-Temporal Data to Intelligent Transportation. Data Science and Engineering. 2021, 6(1): 63-85. doi: 10.1007/s41019-020-00151-z
17. Kim E, Helal S, Cook D. Human Activity Recognition and Pattern Discovery. IEEE Pervasive Computing. 2010, 9(1): 48-53. doi: 10.1109/mprv.2010.7
18. Lv Z, Wang X, Cheng Z, et al. A new approach to COVID-19 data mining: A deep spatial–temporal prediction model based on tree structure for traffic revitalization index. Data & Knowledge Engineering. 2023, 146: 102193. doi: 10.1016/j.datak.2023.102193
19. Li Y, Li J, Lv Z, et al. GASTO: A Fast Adaptive Graph Learning Framework for Edge Computing Empowered Task Offloading. IEEE Transactions on Network and Service Management. 2023, 20(2): 932-944. doi: 10.1109/tnsm.2023.3250395
20. Lv Z, Li J, Xu Z, et al. Parallel Computing of Spatio-Temporal Model Based on Deep Reinforcement Learning. In: Liu Z, Wu F, Das SK (editors). Wireless Algorithms, Systems, and Applications, Proceedings of the 16th International Conference, WASA 2021; 25–27 June 2021; Nanjing, China. Springer; 2021. Volume 12937. pp. 391–403. doi: 10.1007/978-3-030-85928-2_31
21. Lv Z, Li J, Dong C, et al. DeepSTF: A Deep Spatial–Temporal Forecast Model of Taxi Flow. The Computer Journal. 2021, 66(3): 565-580. doi: 10.1093/comjnl/bxab178
22. Xia L, Xu L, Pan S, et al. Drug-target binding affinity prediction using message passing neural network and self supervised learning. BMC Genomics. 2023, 24(1). doi: 10.1186/s12864-023-09664-z
23. Montanino M, Monteil J, Punzo V. From homogeneous to heterogeneous traffic flows: LpString stability under uncertain model parameters. Transportation Research Part B: Methodological. 2021, 146: 136-154. doi: 10.1016/j.trb.2021.01.009
24. Xu Z, Lv Z, Li J, et al. A Novel Approach for Predicting Water Demand with Complex Patterns Based on Ensemble Learning. Water Resources Management. 2022, 36(11): 4293-4312. doi: 10.1007/s11269-022-03255-5
25. Pan S, Xia L, Xu L, et al. SubMDTA: drug target affinity prediction based on substructure extraction and multi-scale features. BMC Bioinformatics. 2023, 24(1). doi: 10.1186/s12859-023-05460-4
26. Tian C, Fei L, Zheng W, et al. Deep learning on image denoising: An overview. Neural Networks. 2020, 131: 251-275. doi: 10.1016/j.neunet.2020.07.025
27. Ye R, Xu Z, Pang J. DDFM: A novel perspective on urban travel demand forecasting based on the ensemble empirical mode decomposition and deep learning. In: Proceedings of the ICBDT 2022: 2022 5th International Conference on Big Data Technologies; 23–25 September 2022; Qingdao, China. pp. 373–379. doi: 10.1145/3565291.3565351
Refbacks
- There are currently no refbacks.
Copyright (c) 2024 Hongyu Yan, Zhiqiang Lv

This work is licensed under a Creative Commons Attribution 4.0 International License.
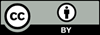
This site is licensed under a Creative Commons Attribution 4.0 International License (CC BY 4.0).
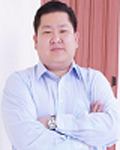
Prof. Kittisak Jermsittiparsert
University of City Island, Cyprus