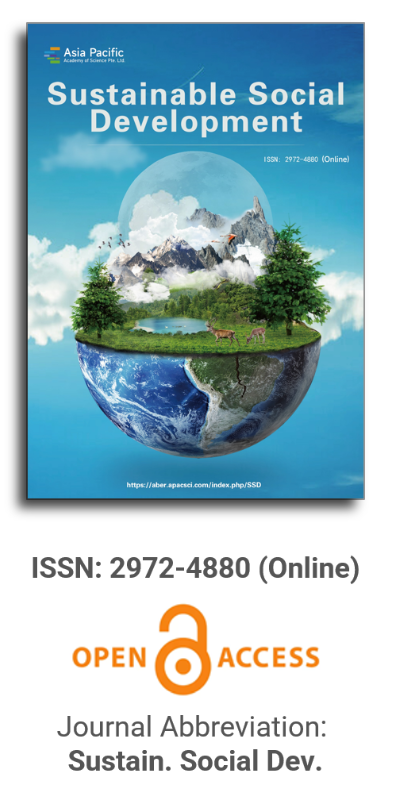
Asia Pacific Academy of Science Pte. Ltd. (APACSCI) specializes in international journal publishing. APACSCI adopts the open access publishing model and provides an important communication bridge for academic groups whose interest fields include engineering, technology, medicine, computer, mathematics, agriculture and forestry, and environment.
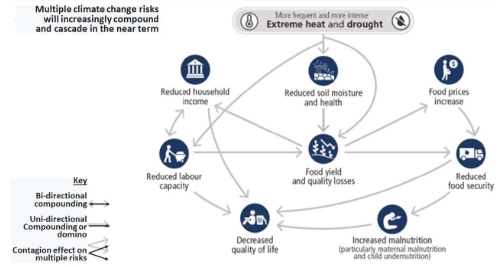
Forecasting the number of road accidents in Poland depending on weather conditions and COVID‐19
Vol 1, Issue 2, 2023
Download PDF
Abstract
COVID‐19 fundamentally changed the way that people travel by road in Poland and throughout the world. The lack of mobility during the period, especially during the beginning of the epidemic, had a significant impact on the number of traffic accidents. The goals of this study are to forecast the number of accidents on the basis of weather in Poland and to assess how the COVID‐19 epidemic has affected that number. For this objective, annual statistics on weather-related traffic accidents were acquired and evaluated. Based on previous information from police records, the number of traffic accidents was also forecast for pandemic and non-pandemic variants in order to assess the impact of the pandemic. The number of traffic accidents in Poland was predicted using specific time series models and exponential models in relation to the weather. There has been a decrease in the number of traffic accidents during the pandemic. Traffic accidents were on average 22% fewer in 2020 than they were in 2019, and by 2021, the difference was over 24%. When it snows or hails, this is extremely clear. This time period mostly saw the outbreak of the pandemic. The majority of traffic accidents occur when the weather is good. When the weather is bad, drivers are more cautious on the road. Prediction of traffic accidents is important for future planning and measures. The problem of estimating the number of traffic accidents, however, is not one that academics are particularly fond of.
Keywords
References
1. WHO. Global status on road safety 2018. Available online: https://www.who.int/publications/i/item/9789241565684 (accessed on 16 May 2022).
2. Tambouratzis T, Souliou D, Chalikias M, Gregoriades A. Maximising accuracy and efficiency of traffic accident prediction combining information mining with computational intelligence approaches and decision trees. Journal of Artificial Intelligence and Soft Computing Research 2014; 4(1): 31–42. doi: 10.2478/jaiscr-2014-0023
3. Zhu L, Lu L, Zhang W, et al. Analysis of accident severity for curved roadways based on Bayesian networks. Sustainability 2019; 11(8): 2223. doi: 10.3390/su11082223
4. Arteaga C, Paz A, Park J. Injury severity on traffic crashes: A text mining with an interpretable machine-learning approach. Safety Science 2020; 132: 104988. doi: 10.1016/j.ssci.2020.104988
5. Yang Z, Zhang W, Feng J. Predicting multiple types of traffic accident severity with explanations: A multi-task deep learning framework. Safety Science 2022; 146: 105522. doi: 10.1016/j.ssci.2021.105522
6. Chen C. Analysis and forecast of traffic accident big data. ITM Web of Conferences 2017; 12: 04029. doi: 10.1051/itmconf/20171204029
7. Khaliq KA, Chughtai O, Shahwani A, et al. Road accidents detection, data collection and data analysis using V2X communication and edge/cloud computing. Electronics 2019; 8(8): 896. doi: 10.3390/electronics8080896
8. Rajput H, Som T, Kar S. An automated vehicle license plate recognition system. Computer 2015; 48(8): 56–61. doi: 10.1109/MC.2015.244
9. Zheng Z, Wang C, Wang P, et al. Framework for fusing traffic information from social and physical transportation data. PLoS One 2018; 13(8): e0201531. doi: 10.1371/journal.pone.0201531
10. Abdullah E, Emam A. Traffic accidents analyzer using big data. In: Proceedings of the 2015 International Conference on Computational Science and Computational Intelligence (CSCI 2015); 07–09 December 2015; Las Vegas, NV, USA. pp. 392–397.
11. Vilaça M, Silva N, Coelho MC. Statistical analysis of the occurrence and severity of crashes involving vulnerable road users. Transportation Research Procedia 2017; 27: 1113–1120. doi: 10.1016/j.trpro.2017.12.113
12. Bąk I, Cheba K, Szczecińska B. The statistical analysis of road traffic in cities of Poland. Transportation Research Procedia 2019; 39: 14–23. doi: 10.1016/j.trpro.2019.06.003
13. Chand A, Jayesh S, Bhasi AB. Road traffic accidents: An overview of data sources, analysis techniques and contributing factors. Materialstoday Proceedings 2021; 47(15): 5135–5141. doi: 10.1016/j.matpr.2021.05.415
14. Helgason A. Fractional integration methods and short Time series: Evidence from a simulation study. Cambridge University Press 2016; 24(1): 59–68. doi: 10.1093/pan/mpv026
15. Lavrenz SM, Vlahogianni EI, Gkritza K, Ke Y. Time series modeling in traffic safety research. Accident Analysis & Prevention 2018; 117: 368–380. doi: 10.1016/j.aap.2017.11.030
16. Forecasting based on time series (Polish). Available online: http://pis.rezolwenta.eu.org/Materialy/PiS-W-5.pdf (accessed on 16 May 2022).
17. Procházka J, Flimmel S, Čamaj M, Bašta M. Modelling the number of road accidents. In: Proceedings of the 20th International Scientific Conference, Applications of Mathematics and Statistics in Economics (AMSE 2017). 30–3 September 2017; Szklarska Por˛eba, Poland.
18. Sunny CM, Nithya S, Sinshi KS, et al. Forecasting of road accident in Kerala: A case study. In: Proceedings of the 2018 International Conference on Data Science and Engineering (ICDSE 2018); 07–09 August 2018; Kochi, India.
19. Dudek G. Forecasting time series with multiple seasonal cycles using neural networks with local learning. In: Rutkowski L, Korytkowski M, Scherer R, et al. (editors). Artificial Intelligence and Soft Computing, Proceedings of the 12th International Conference, ICAISC 2013; 9–13 June 2013; Zakopane, Poland. Springer-Verlag Berlin Heidelber; 2013. Volume 7894, pp. 52–63.
20. Szmuksta-Zawadzka M, Zawadzki J. Forecasting on the basis of Holt-Winters models for complete and incomplete data (Polish). Research Papers of the Wrocław University of Economics 2009; 38: S85–S99.
21. Wójcik A. Autoregressive vector models as a response to the critique of multi-equation structural econometric models (Polish). Studia Ekonomiczne 2014; 193: 112–128.
22. Monederoa BD, Gil-Alanaa LA, Martínezaa MCV. Road accidents in Spain: Are they persistent? IATSS Research 2021: 45(3): 317–325. doi: 10.1016/j.iatssr.2021.01.002
23. Al-Madani HMN. Global road fatality trends’ estimations based on country-wise microlevel data. Accident Analysis & Prevention 2018; 111: 297–310. doi: 10.1016/j.aap.2017.11.035
24. Mamczur M. Machine learning how does linear regression work? And is it worth using (Polish)? Available online: https://miroslawmamczur.pl/jak-dziala-regresja-liniowa-i-czy-warto-ja-stosowac/ (accessed on 16 May 2022).
25. Biswas AA, Mia J, Majumder A. Forecasting the number of road accidents and casualties using random forest regression in the context of Bangladesh. In: Proceedings of the 2019 10th International Conference on Computing, Communication and Networking Technologies (ICCCNT 2019); 30 December 2019; Kanpur, India.
26. Fijorek K, Mróz K, Niedziela K, Fijorek D. Forecasting electricity prices on the day-ahead market using data mining methods (Polish). Rynek Energii 2010; 6: 46–50.
27. Chudy-Laskowska K, Pisula T. Forecast of the number of road accidents in Poland (Polish). Logistyka 2014; 6: 2710–2712.
28. Kashpruk N. Comparative Research of Statistical Models and Soft Computing for Identification of Time Series and Forecasting (Polish) [PhD thesis]. Politechnika Opolska; 2010.
29. Prochazka J. Camaj M. Modelling the number of road accidents of uninsured drivers and their severity. In: Kaderabkova B, Holmanova J, Rotschedl J (editors). Proceedings of International Academic Conferences. Proceedings of the 5th Business & Management Conference, Rome; 05–08 April 2017; Prague, Czech Republic.
30. Dutta B, Barman MP, Patowary AN. Application of Arima model for forecasting road accident deaths in India. International Journal of Agricultural and Statistical 2020; 16(2): 607–615.
31. Karlaftis MG, Vlahogianni EI. Memory properties and fractional integration in transportation time-series. Transportation Research Part C: Emerging 2009; 17(4): 444–453. doi: 10.1016/j.trc.2009.03.001
32. Łobejko S, Masłowska K, Wojdan R. Time Series Analysis and Forecasting with SAS (Polish). Warszawa; 2015.
33. Dudek G. Exponential smoothing models for short-term power system load forecasting (Polish). Rynek Energii 2013; 3: 14–19.
34. Chudy-Laskowska K, Pisula T. Forecasting the number of traffic accidents in Subcarpathia. Logistyka 2015; 4(2): 2782–2796.
35. Wrobel MS. Application of Neural Fuzzy Systems in Chemistry (Polish) [PhD thesis]. University of Silesia; 2017.
36. Data mining techniques (Polish). Available online: https://www.statsoft.pl/textbook/stathome_stat.html?https%3A%2F%2Fwww.statsoft.pl%2Ftextbook%2Fstdatmin.html (accessed on 16 May 2022).
37. Kumar PS, Viswanadham V, Bharathi B. Analysis of road accident. IOP Conference Series: Materials Science and Engineering 2019; 590: 012029. doi: 10.1088/1757-899X/590/1/012029
38. Top advantages and disadvantages of Hadoop 3. Available online: https://data-flair.training/blogs/advantages-and-disadvantages-of-hadoop/ (accessed on 16 May 2022).
39. Perczak G, Fiszeder P. GARCH model-using additional information on minimum and maximum prices (Polish). Bank i Kredyt 2014; 2: 105–131.
40. Fiszeder P. GARCH Class Models in Empirical Financial Research (Polish) [Master’s thesis]. Wydawnictwo Naukowe Uniwersytetu Mikołaja Kopernika; 2009
41. McIlroy RC, Plant KA, Hoque MS, et al. Who is responsible for global road safety? A cross-cultural comparison of actor maps. Accident Analysis & Prevention 2019; 122: 8–18. doi: 10.1016/j.aap.2018.09.011
42. Muck J. Econometrics. Time series modeling. Stationarity. Unit root tests. Unit root. ARDL models. Cointegration (Polish). Available online: http://web.sgh.waw.pl/~jmuck/Ekonometria/EkonometriaPrezentacja5.pdf (accessed on 16 May 2022).
43. Shetty P, Sachin PC, Kashyap VK, Madi V. Analysis of road accidents using data mining techniques. Inernational Research Journal of Engineering and Technology 2017; 4(4): 1494–1496.
44. Li L, Shrestha S, Hu G. Analysis of road traffic fatal accidents using data mining techniques. In: Proceedings of the 2017 IEEE 15th International Conference on Software Engineering Research, Management and Applications (SERA 2017); 07–09 June 2017; London, UK. pp. 363–370.
45. Marcinkowska K. Statistical methods and data mining in assessing the occurrence of syncope in the group of narrow QRS complex (AVNRT and AVRT) (Polish) [PhD thesis]. Medical University in Poznan; 2015.
46. Sebego M, Naumann RB, Rudd RA, et al. The impact of alcohol and road traffic policies on crash rates in Botswana, 2004–2011: A time-series analysis. Accident Analysis & Prevention 2014; 70: 33–39. doi: 10.1016/j.aap.2014.02.017
47. Bloomfield P. An exponential model in the spectrum of a scalar time series. Biometrika 1973; 60(2): 217–226.
48. Gorzelańczyk P, Jurkovič M, Kalina T, Mohanty M. Forecasting the road accident rate and the impact of the COVID 19 on its frequency in the polish provinces. Komunikácie 2022; 24(4): A216–A231. doi: 10.26552/com.C.2022.4.A216-A231
49. Jurkovic M, Gorzelanczyk P, Kalina T, et al. Impact of the COVID‐19 pandemic on road traffic accident forecasting in Poland and Slovakia. Open Engineering 2022; 12(1): 578–589. doi: 10.1515/eng-2022-0370
50. Statistic road accident (Polish). Available online: https://statystyka.policja.pl/ (accessed on 16 May 2022).
Refbacks
- There are currently no refbacks.
Copyright (c) 2023 Piotr Gorzelanczyk
License URL: https://creativecommons.org/licenses/by/4.0/
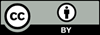
This site is licensed under a Creative Commons Attribution 4.0 International License (CC BY 4.0).
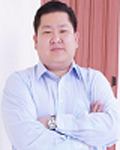
Prof. Kittisak Jermsittiparsert
University of City Island, Cyprus
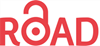
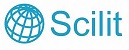
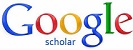
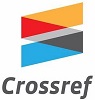
Title: Economic Sustainability and Innovation
Deadline: June 30, 2025.