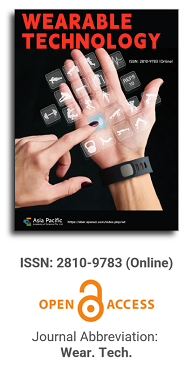
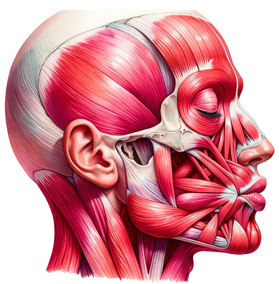
This paper delves deeply into the innovative realm of integrating human emotions with wearable technology. The primary focus is on the conceptualization and development of a kiss transfer device that harnesses the power of wearable technology to bridge the physical gap in human-human interactions. By investigating the intricate nuances of the human-human kissing process, the research seeks to replicate this intimate gesture through a technological medium. The paper not only elaborates on the anatomy, evolution, and hormonal dynamics of kissing but also underscores the transformative potential of wearable technology in capturing and transmitting these intimate moments. This exploration opens up new horizons for long-distance relationships, offering a tangible touchpoint that goes beyond traditional communication methods. Through this pioneering work, the research positions wearable technology as not just a tool for communication but as an extension of our human emotions and expressions.
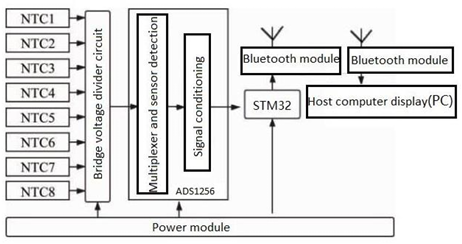
Research progress on wearable devices for daily human health management
Vol 2, Issue 1, 2021
Download PDF
Abstract
As the public’s demand for portable access to personal health information continues to expand, wearable devices are not only widely used in clinical practice, but also gradually applied to the daily health management of ordinary families due to their intelligence, miniaturization, and portability. This paper searches the literature of wearable devices through PubMed and CNKI databases, classifies them according to the different functions realized by wearable devices, and briefly describes the algorithms and specific analysis methods of their applications and made a prospect of its development trend in the field of human health.
Keywords
References
- Jalloul N, Poree F, Viardot G, et al. Activity recognition using complex network analysis. IEEE Journal of Biomedical and Health Informatics 2018; 22(4): 989–1000.
- Ahola TM. Pedometer for running activity using accelerometer sensors on the wrist. Medical Equipment Insights 2010; (3): 1–8.
- Gaglani S, Moore J, Haynes MR, et al. Using commercial activity monitors to measure gait in patients with suspected iNPH: Implications for ambulatory monitoring. Cureus 2015; 7(11): e382.
- Winfree KN, Dominick G. Modeling clinically validated physical activity assessments using commodity hardware. 2017 IEEE EMBS International Conference on Biomedical and Health Informatics; 2012 Jan 5-7 Jan; Orlando: IEEE Press; p. 157–160.
- Tao R, Zhang C. Research on the validity of the step counting function of fitness APP in the era of artificial intelligence. Abstracts of the 11th National Sports Science Conference; 2019 Nov 1–3; Nanjing: Chinese Society of Sports Science. p. 5468–5470.
- Toth LP, Park S, Springer CM, et al. Video-recorded validation of wearable step counters under free-living conditions. Medicine and Science in Sports and Exercise 2018; 50(6): 1315–1322.
- Bassett DR, Toth LP, LaMunion SR, et al. Step counting: A review of measurement considerations and health-related applications. Sports Medicine 2017; 47(7): 1303–1315.
- EI Arawy F, Nounou MI. Are currently available wearable devices for activity tracking and heart rate monitoring accurate, precise, and medically beneficial? Healthcare Informatics Research 2015; 21(4): 315–320.
- Pande A, Zhu J, Das AK, et al. Using smart-phone sensors for improving energy expenditure estimation. IEEE Journal of Translational Engineering in Health and Medicine 2015; 3: 270021.
- Shcherbina A, Mattsson CM, Waggott D, et al. Accuracy in wristworn, sensor-based measurements of heart rate and energy Expenditure in a diverse cohort. Journal of Personalized Medicine 2017; 7(2): 3.
- Roos L, Taube W, Beeler N, et al. Validity of sports watches when estimating energy expenditure during running. BMC Sports Science, Medicine and Rehabilitation 2017; 9(1): 22.
- Ryu JS, Park JJ. Validation of wearable devices to measure energy consumption. The Asian Journal of Kinesiology 2020; 22(1): 33–37.
- Guo YN, Tao DP, Liu WF, et al. Multiview cauchy estimator feature embedding for depth and inertial sensor-based human action recognition. IEEE Transactions on Systems, Man, and Cybernetics: Systems 2017; 47(4): 617–627.
- Mooney R, Qunilan LR, Corley G, et al. Evaluation of the Finis Swimsense (R) and the Garmin Swim (TM) activity monitors for swimming performance and stroke kinematics analysis. PloSOne 2017; 12(2): e0170902.
- Kanoga S, Kanemura A, Asoh H. Are armband sEMG devices dense enough for long-term use?—Sensor placement shifts cause significant reduction in recognition accuracy. Biomedical Signal Processing and Control 2020; 60: 101981.
- Gualtieri L, Rosenbluth S, Phillips J. Can a free wearable activity tracker change behavior? The impact of trackers on adults in a physician-led wellness group. JMIR Research Protocols 2016; 5(4): e237.
- De Brouwer M, Ongenae F, Bonte P, et al. Towards a cascading reasoning framework to support responsive ambient-intelligent healthcare interventions. Sensors 2018; 18(10): 3514.
- Jovanov E. Wearables meet IoT: Synergistic personal area networks (SPANs). Sensors 2019; 19(19): 4295.
- Voss C, Schwartz J, Daniels J, et al. Effect of wearable digital intervention for improving socialization in children with autism spectrum disorder a randomized clinical trial. JAMA Pediatrics 2019; 173(5): 446–454.
- De Zambotti M, Cellini N, Goldstone A, et al. Wearable sleep technology in clinical and research settings. Medicine and Science in Sports and Exercise 2019; 51(7): 1538–1557.
- Gautam A, Naik VS, Gupta A, et al. An smartphone-based algorithm to measure and model quantity of sleep. The 7th International Conference on Communication Systems and Networks; 2017 Nov 11–13; Bangalore: IEEE Press; p. 1–6.
- Meltzer LJ, Hiruma LS, Avis K, et al. Comparison of a commercial accelerometer with polysomnography and actigraphy in children and adolescents. Sleep 2015; 38(8): 1323–1330.
- Xie J, Wen D, Liang L, et al. Evaluating the validity of current mainstream wearable devices in fitness tracking under various physical activities: Comparative study. JMIR mHealth and uHealth 2018; 6(4): e94.
- Wang Y, Zhang S, Xu W. Portable and wearable ECG measurement devices in atrial fibrillation application in screening. Chinese Medical Journal 2020; 55(06): 592–593.
- Aboy M, McNames J, Thong T, et al. An automatic beat detection algorithm for pressure signals. IEEE Transactions on Biomedical Engineering 2005; 52(10): 1662–1670.
- Xu X, Wei S, Ma C, et al. Atrial fibrillation beat identification using the combination of modified frequency slice wavelet transform and convolutional neural networks. Journal of Healthcare Engineering 2018; 2018: 2102918.
- Fan P, Chen C, Peng Y, et al. Clinical application of E-U08 snap ECG recorder in remote monitoring of arrhythmia and myocardial ischemia. Journal of Practical Electrocardiology 2017; 26(1): 10–15.
- William AD, Kanbour M, Callahan T, et al. Assessing the accuracy of an automated atrial fibrillation detection algorithm using smartphone technology: The iREAD study. Heart Rhythm 2018; 15(10): 1561–1565.
- Bumgarner JM, Lambert CT, Hussein AA, et al. Smartwatch algorithm for automated detection of atrial fibrillation. Journal of the American College of Cardiology 2018; 71(21): 2381–2388.
- January CT, Wann LS, Calkins H, et al. 2019 AHA/ACC/HRS focused update of the 2014 AHA/ACC/HRS guideline for the management of patients with atrial fibrillation: A report of the American College of Cardiology/American Heart Association task force on clinical practice guidelines and the heart rhythm society. Circulation 2019; 140(2): e125–e151.
- Zhao X, Hu A, He W. Fall detection based on convolutional neural network and XGBoost. Las Optoelect Prog 2020; 57(16): 16102.
- Aicha AN, Englebienne G, Van Schooten KS, et al. Deep learning to predict falls in older adults based on daily-life trunk accelerometry. Sensors 2018; 18(5): 1654.
- Özdemir AT. An analysis on sensor locations of the human body for wearable fall detection devices: Principles and practice. Sensors 2016; 16(8): 1161.
- Zhen X, Tian J, Shi Z, et al. Analysis on the application value of wearable devices in blood glucose management of diabetic patients. China Modern Doctor 2020; 58(10): 100–103.
- Hamdi T, Ali JB, Costanzo VD, et al. Accurate prediction of continuous blood glucose based on support vector regression and differential evolution algorithm. Biocybernetics and Biomedical Engineering 2018; 38(2): 362–372.
- Mhaskar HN, Pereverzyev SV, Van Der Walt MD. A deep learning approach to diabetic blood glucose prediction. Frontiers in Applied Mathematics and Statistics 2017; 3: 14.
- Bonn SE, Alexandrou C, Steiner KH, et al. App-technology to increase physical activity among patients with diabetes type 2-The DiaCert-study, a randomized controlled trial. BMC Public Health 2018; 18: 119.
- Jia N, Li Y. Construction of personalized health monitoring platform based on intelligent wearable devices. Computer Science 2019; 46(z1): 566–570.
- Schutz Y, Weinsier RL, Hunter GR. Assessment of free-living physical activity in humans: An overview of currently available and proposed new measures. Obesity Research 2001; 9(6): 368–379.
- Perez MV, Mahaffey KW, Hedlin H, et al. Large-scale assessment of a smartwatch to identify atrial fibrillation. The New England Journal of Medicine 2019; 381(20): 1909–1917.
- Gilani M, Eklund JM, Makrehchi M. Automated detection of atrial fibrillation episode using novel heart rate variability feature. The 38th Annual International Conference of the IEEE Engineering in Medicine and Biology Society (EMBC); 2016 Aug 16–20; Orlando: IEEE Press; p. 3461–3464.
- Hu K, Chen X, Zhang S, et al. Research and application of wearable devices in rehabilitation medicine in developed countries. China Digital Medicine 2018; 13(8): 56–59, 15.
- Kou J. Deepening the application of smart wearable devices in the medical and health field. People’s Posts and Telecommunications, 2020 Jun 12.
- Wu X, Wu Y, Huang X, et al. Study on the optimization of health management model for the elderly in urban communities under the background of smart medical treatment. China Medical Herald 2020; 17(33): 194–196.
Refbacks
- There are currently no refbacks.
Copyright (c) 2021 Anan Li, Ping Shi

This work is licensed under a Creative Commons Attribution 4.0 International License.
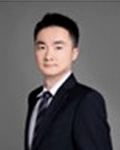
Prof. Zhen Cao
College of Information Science & Electronic Engineering, Zhejiang University
China, China
Processing Speed
-
-
-
- <5 days from submission to initial review decision;
- 62% acceptance rate
-
-
Asia Pacific Academy of Science Pte. Ltd. (APACSCI) specializes in international journal publishing. APACSCI adopts the open access publishing model and provides an important communication bridge for academic groups whose interest fields include engineering, technology, medicine, computer, mathematics, agriculture and forestry, and environment.