Arrhythmia classification based on convolution neural network feature extraction and fusion
Vol 2, Issue 1, 2021
VIEWS - 48 (Abstract)
Abstract
This study proposes a new automatic classification method of arrhythmias to assist doctors in diagnosing and treating arrhythmias. The convolution neural network is constructed to extract the features of ECG signals and wavelet components of QRS complex. The ECG signal features and wavelet features extracted by the network and the artificially extracted RR interval features are input to the full connection layer for fusion, and the softmax function is used to classify the beats in the output layer. The network is trained and tested using the mil lead data in MIT BIH arrhythmia database. The overall classification accuracy of this method is 98.12%, the average sensitivity is 87.32%, and the average positive predictive value is 90.37%. This method can quickly identify different types of arrhythmias, and has certain reference value for the application of computer-aided diagnosis of arrhythmias.
Keywords
Full Text:
PDFReferences
1. He K, Nie Z, Zhong G, et al. Localization of origins of premature ventricular contraction in the whole ventricle based on machine learning and automatic beat recognition from 12 lead ECG. Physiologicalmeasurement 2020; 41(5): 55–60.
2. Mondéjar Guerra V, Novo J, Rouco J, et al. Heartbeat classification fusing temporal and morphological information of ecgs via ensemble of classifiers. Biomedical Signal Processing and Control 2019; 47: 41–48.
3. Shi H T, Wang HR, Zhang F, et al. Inter patient heartbeat classification based on region feature extraction and ensemble classifier. Biomedical Signal Processing and Control 2019; 51: 97–105.
4. Li Hongqiang, weixiaoqing, Wang Youxi, et al Application of principal component analysis and linear discriminant analysis in ECG feature extraction and diagnosis algorithm. Biomedical engineering research 2019; 38 (2): 145, 150
5. Rajpurkar P, Hannun A Y, Haghpanahi M, et al. Cardiologist level arrhythmia detection with convolutional neural networks [EB/ OL]. (2017 07 06) [2020 07 17]. Available from: Https://arxiv.org/ abs/1707.01836.
6. Shi H T, Qin C J, Xiao D Y, et al. Automated heartbeat classification based on deep neural network with multiple input layers. Knowledge Based Systems 2020; 188: 105–136.
7. Parvaneh S, Rubin J, Babaeizadeh S, et al. Cardiac arrhythmia detec-tion using deep learning: A review. Journal of Electrocardiology 2019; 57: S70–S74.
8. Moody G B, markrg. The impact of the mit bih arrhythmia database. IEEE Engineering in Medicine and Biology Magazine 2001; 20(3): 45–50.
9. Liyanjun, tangxiaoying, Xu Zhi, et al A method of detecting arrhythmia by heart rate. Aerospace Medicine and medical engineering 2016; 29 (1): 21–27.
10. Teijeiro T, García C A, Castro D, et al. Arrhythmia classification from the abductive interpretation of short single lead ECG records [EB/ OL]. [2020 07 17]. Available from: Https://arxiv.org/abs/1711.03892.
11. de Chazal P, O'Dwyer M, reillyrb. Automatic classification of heartbeats using ECG morphology and heartbeat interval features. IEEE Transactions on Biomedical Engineering 2004; 51(7): 1196–1206.
12. Kiranyaz S, Ince T, Gabbouj M. Real time patient specific ecgclassification by 1-D convolutional neural networks. IEEE Transactions on Biomedical Engineering, 2016, 63(3): 664–675.
13. Kennedy A, Finlay D D, Guldenring D, et al. Automated detection of atrial fibrillation usingrrintervals and multivariate based classification. Journal of Electrocardiology 2016; 49(6): 871–876.
14. Luo K, Li J, Wang Z, et al. Patient specific deep architectural model for ECG classification. Journal of Healthcare Engineering 2017; 2: 264–274.
15. Xiang YD, Luo JH, Zhu TT, et al. ECG based heartbeat classification using two level convolutional neural network andrrinterval difference. IEICE Transactions on Information and Systems 2018; (4): 1189–1198.
Refbacks
- There are currently no refbacks.
Copyright (c) 2021 Chenhua Xu, Sichao Ye, Qingli Qiao

This work is licensed under a Creative Commons Attribution 4.0 International License.
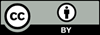
This site is licensed under a Creative Commons Attribution 4.0 International License (CC BY 4.0).
eISSN
2811-0099
Publication Frequency
Semi-annual