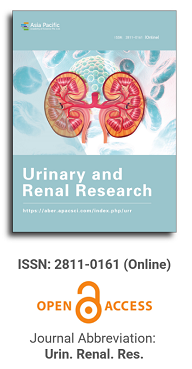
Asia Pacific Academy of Science Pte. Ltd. (APACSCI) specializes in international journal publishing. APACSCI adopts the open access publishing model and provides an important communication bridge for academic groups whose interest fields include engineering, technology, medicine, computer, mathematics, agriculture and forestry, and environment.
Decision tree algorithm applied to MIMIC-III database for the prediction of acute kidney injury in ICU patients
Vol 2, Issue 1, 2021
Download PDF
Abstract
Objective Acute kidney injury (AKI) is one of the most common complications and fatal factors in intensive care unit (ICU). Accurate prediction of AKI risk and identification of key factors related to AKI can provide effective guidance for clinical decision-making and intervention for patients with AKI risk. Methods A total of 30 020 patients in ICU (including 17 222 AKI patients and 12 798 Non-AKI patients) were selected from the public database MIMIC-III in this study, and basic information, physiological and biochemical indicators, drug use, and comorbidity during their stay in ICU were collected. All patients were randomly divided into training sets and independent testing sets according to the ratio of 4:1, and logistic regression, random forest, and lightgbm were applied to construct models for AKI predication in three time points including 24 h, 48 h and 72 h, respectively. The 10-fold cross validation was used to train and validate various models to predict the occurrence of AKI, and obtain important features. Furthermore, 24 h prediction models were used to predict AKI every 24 h during the 7-day window. Results lightgbm achieved the best performance with AUC values of 0.90, 0.88, 0.87 for 24 h, 48 h, and 72 h prediction, respectively, and F1 values were 0.91, 0.88, and 0.86. In prediction of every 24 h, the success rates of identifying AKI patients were 89%, 83%, and 80% in one day, two days and three days in advance, respectively. It was found that the length of stay in ICU, body weight, albumin, systolic blood pressure, bicarbonate, glucose, white blood cell count, body temperature, diastolic blood pressure and blood urea nitrogen played vital roles in predicting AKI for ICU patients. Using only 24 important features, the models could still achieve prominent prediction performance. Conclusions Based on basic information, physiological and biochemical indicators, drug use, and comorbidity, machine learning methods can be adopted to effectively predict AKI risk for ICU patients at several time points, and determine the dominant factors relative to AKI.
Keywords
References
1. Lameire NH, Bagga A, Cruz D, et al. Acute kidney injury: an increasing global concern [J]. Lancet, 2013, 382 (9887) : 170-179.
2. Bagshaw SM, George C, Gibney RTN, et al. A multi-center evaluation of early acute kidney injury in critically ill trauma patients[J]. Renal Failure, 2008, 30(6) : 581-589.
3. Mizuno T, Sato W, Ishikawa K, et al. KDIGO (kidney disease: improving global outcomes) criteria could be a useful outcome predictor of cisplatin-induced acute kidney injury[J]. Oncology, 2012, 82(6) : 354-359.
4. Eriksson M, Brattstrm O, Mrtensson J, et al. Acute kidney injury following severe trauma: risk factors and long-term outcome[J]. Journal of Trauma and Acute Care Surgery, 2015, 79 (3) : 407-412.
5. Ostermann M, Joannidis M. Acute kidney injury 2016: diagnosis and diagnostic workup[J]. Critical Care, 2016, 20: 299.
6. Panagidis D, Nanas S, Kokkoris S. Biomarkers of acute kidney injury in a mixed ICU population. A narrative review[J]. Health & Research Journal, 2019, 5(4) : 150.
7. Rojas JC, Carey KA, Edelson DP, et al. Predicting intensive care unit readmission with machine learning using electronic health record data[J]. Annals of the American Thoracic Society, 2018, 15(7) : 846-853.
8. Haines RW, Lin SP, Hewson R, et al. Acute kidney injury in trauma patients admitted to critical care: development and validation of a diagnostic prediction model [J]. Scientific Reports, 2018, 8: 3665.
9. Malhotra R, Kashani KB, Macedo E, et al. A risk prediction score for acute kidney injury in the intensive care unit[J]. Nephrology Dialysis Transplantation, 2017, 32(5) : 814-822.
10. Li QH. Prediction of acute kidney injury and clinical application optimization based on machine learning[D]. Beijing: Beijing Jiaotong University, 2019.
11. Zhang Y, Feng C, Li KY, et al. Lightgbm model for predicting acute kidney injury risk in ICU patients[J]. Academic Journal of Chinese PLA Medical School, 2019, 40(4) : 316-320.
12. Zimmerman LP, Reyfman PA, Smith A, et al. Early prediction of acute kidney injury following ICU admission using a multivariate panel of physiological measurements [J]. BMC Medical Informatics and Decision Making, 2019, 19(Suppl 1) : 16.
13. Johnson A, Pollard TJ, Shen L, et al. MIMIC-III, a freely accessible critical care database[J]. Scientific Data, 2016 (3) : 160035.
14. Peng C, Waitman LR, Yong H, et al. Predicting inpatient acute kidney injury over different time horizons: How early and accurate? [J]. AMIA... Annual Symposium Proceedings/AMIA Symposium, 2018, 2017(2017) : 565-574.
15. van Houwelingen S. Ridge estimators in logistic regression[J]. Journal of the Royal Statistical Society Series C, 1992, 41 (1) : 191-201.
16. Criminisi A, Shotton J, Konukoglu E. Decision forests: a unified framework for classification, regression, density estimation, manifold learning and semi-supervised learning[J]. Foundations and Trends in Computer Graphics and Vision, 2011, 7 (2-3) : 81-227.
17. Ke G, Meng Q, Finley T, et al. Lightgbm: a highly efficient gradient boosting decision tree [C]//NIPS’17: the 31st International Conference on Neural Information Processing Systems. Long Beach, USA: NIPS, 2017, 3149-3157.
18. Mallet ML. Pathophysiology of accidental hypothermia[J]. QJM, 2002, 95(12) : 775-785.
19. Mégarbane B, Axler O, Chary I, et al. Hypothermia with indoor occurrence is associated with a worse outcome[J]. Intensive Care Medicine, 2000, 26(12) : 1843-1849.
20. Han SS, Ahn SY, Ryu J, et al. U-shape relationship of white blood cells with acute kidney injury and mortality in critically ill patients[J]. The Tohoku Journal of Experimental Medicine, 2014, 232(3) : 177-185.
21. Gujadhur A, Tiruvoipati R, Cole E, et al. Serum bicarbonate may independently predict acute kidney injury in critically ill patients: an observational study [J]. World Journal of Critical Care Medicine, 2015, 4(1) : 71-76.
Refbacks
- There are currently no refbacks.
Copyright (c) 2021 Wenpeng Gao, Haijin Lyu, Lang Zhou, Shengwen Guo

This work is licensed under a Creative Commons Attribution-NonCommercial 4.0 International License.
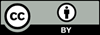
This site is licensed under a Creative Commons Attribution 4.0 International License (CC BY 4.0).
.png)
Prof. Wei-Yen Hsu
National Chung Cheng University, Taiwan