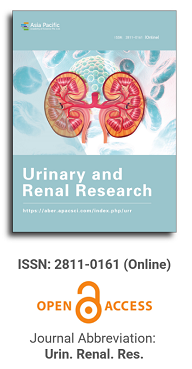
Asia Pacific Academy of Science Pte. Ltd. (APACSCI) specializes in international journal publishing. APACSCI adopts the open access publishing model and provides an important communication bridge for academic groups whose interest fields include engineering, technology, medicine, computer, mathematics, agriculture and forestry, and environment.
Classification of renal tumor histology subtypes based on radiomics features of CT images
Vol 5, Issue 1, 2024
Download PDF
Abstract
Objective Accurate preoperative differential diagnosis of fat⁃poor angiomyolipoma (fp⁃AML) and clear cell renal cell carcinoma (ccrcc) is essential for proper treatment planning. In order to increase the accuracy of discrimination of fp⁃AML from ccrcc, we develop a classification model based on radiomics technology. Methods The study retrospectively collected CT images of 18 cases with fp⁃AML and 42 cases with ccrcc from department of radiology, the Second Affiliated Hospital of Suzhou University. Firstly, 430 radiomics features were extracted from CT images. Then, the feature selection was carried by three steps: Pearson’s correlation matrices were calculated to remove redundant features, Welch’s t⁃test was utilized to determine the statistically significant features, and sequential forward floating selection method was used to select the discriminative features. Finally, k⁃nearest neighborhood, random forest, support vector machine and adaboost classifiers were built for classification. Results The model built by SVM classifier achieved the best classification performance, with accuracy, sensitivity, specificity, positive predictive value, negative predictive value, and area under the receiver operating characteristic curves of 91.67%, 88.89%, 92.86%, 84.21%, 95.12%, and 0.9418. Conclusions The proposed model can increase the classification accuracy of discrimination of fp⁃AML from ccrcc, and has great potential in helping radiologists to discriminate fp⁃AML from ccrcc.
Keywords
References
1. Hsieh JJ, Purdue MP, Signoretti S, et al. Renal cell carcinoma [J] . Nature Reviews Disease Primers, 2017, 3:17009.
2. Wei J, Zhao J, Zhang X, et al. Analysis of dual energy spectral CT and pathological grading of clear cell renal cell carcinoma (ccrcc) [J] . Plos One, 2018, 13(5) :e0195699.
3. Prasad SR, Surabhi VR, Menias CO, et al. Benign renal neoplasms in adults: cross⁃sectional imaging findings [J] . American Journal of Roentgenology, 2008, 190(1) :158-164.
4. Lane BR, Aydin H, Danforth TL, et al. Clinical correlates of renal angiomyolipoma subtypes in 209 patients: classic, fat poor, tuberous sclerosis associated and epithelioid [J] . The Journal of Urology, 2008, 80(3) :836-843.
5. Yan L, Liu Z, Wang G, et al. Angiomyolipoma withminimal fat : differentiation from clear cell renal cell carcinoma and papillary renal cell carcinoma by texture analysis on CT images [J]. Academic Radiology,2015,22(9):1115.
6. Xu Y. Preliminary study on differential diagnosis of renal angiomyolipoma and renal clear cell carcinoma based on CT image texture analysis [D]. Shenyang:China Medical University, 2017.
7. Feng Z, Rong P, Cao P, et al. Machine learning⁃based quantitative texture analysis of CT images of small renal masses: Differentiation of angiomyolipoma without visible fat from renal cell carcinoma [J]. European Radiology, 2018,28(4):1625-1633.
8. Lee HS, Hong H, Jung DC, et al. Differentiation of fat⁃poor angiomyolipoma from clear cell renal cell carcinoma in contrast⁃enhanced MDCT images using quantitative feature classification [J]. Medical Physics,2017,44(7):3604-3614.
9. Lambin P,Leijenaar RTH,Deist TM,et al. Radiomics:the bridge between medical imaging and personalized medicine [J]. Nature Reviews Clinical Oncology,2017,14:749.
10. Bernardi ED,Buda A,Guerra L, et al. Radiomics of the primary tumour as a tool to improve 18F -FDG⁃PET sensitivity in detecting nodal metastases in endometrial cancer [J]. EJNMMI Research, 2018,8(1):86.
11. Ferreira Junior JR, Koenigkam⁃Santos M, Cipriano FEG, et al. Radiomics⁃based features for pattern recognition of lung cancer histopathology and metastases [J]. Computer Methods and Programs in Biomedicine, 2018,159:23-30.
12. Sapate SG, Mahajan A, Talbar SN, et al. Radiomics based detection and characterization of suspicious lesions on full field digital mammograms [J]. Computer Methods and Programs in Biomedicine,2018,163:1-20.
13. Meng X,Xia W,Xie P,et al. Preoperative radiomic signature based on multiparametric magnetic resonance imaging for noninvasive evaluation of biological characteristics in rectal cancer [J]. European Radiology,2019,29(6):3200-3209.
14. Inza I,Larran~aga P,and Saeys Y. A review of feature selection techniques in bioinformatics [J]. Bioinformatics,2007,23(19): 2507-2517.
15. Zwanenburg A, Leger S, Vallières M, et al. Image biomarker standardisation initiative ⁃feature definitions [D/ OL]. [2016-12-21]. Http://arxiv. Cry/ pdf/0612.07003. Pdf.
16. Lubner MG, Smith AD, Sandrasegaran K, et al. CT texture analysis: definitions, applications, biologic correlates, and Challenges [J]. Radiographics, 2017,37(5):1483-1503.
17. Harikumar R,Vinoth kumar B. Performance analysis of neural networks for classification of medical images with wavelets as a feature extractor [J]. International Journal of Imaging Systems and Technology,2015,25(1):33-40.
18. Pearson K. Note on regression and inheritance in the case of two parents [J]. Proceedings of the Royal Society of London,1895, 58:240-242.
19. Derrick B,White P. Why Welch’ s test is Type I error robust [J]. The Quantitative Methods in Psychology,2016,12 (1): 30-38.
20. Aha DW, Bankert RL. A comparative evaluation of sequential feature selection algorithms [M]// Learning from data:artificial intelligence and statistics. Fisher VD,Lenz HJ,Eds. New York: Springer New York,1996:199-206.
21. Bugdol MD, Bugdol MN, Lipowicz AM, et al. Prediction of menarcheal status of girls using voice features [J]. Computers in Biology and Medicine,2018,100:296-304.
22. Fallahpour S, Lakvan EN, Zadeh MH. Using an ensemble classifier based on sequential floating forward selection for financial distress prediction problem [J]. Journal of Retailing and Consumer Services,2017,34:159-167.
23. Chapelle O, Vapnik V, Bousquet O, et al. Choosing multiple parameters for support vector machines [J]. Machine Learning, 2002,46(1):131-159.
Refbacks
- There are currently no refbacks.
Copyright (c) 2024 Author(s)

This work is licensed under a Creative Commons Attribution-NonCommercial 4.0 International License.
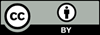
This site is licensed under a Creative Commons Attribution 4.0 International License (CC BY 4.0).
.png)
Prof. Wei-Yen Hsu
National Chung Cheng University, Taiwan