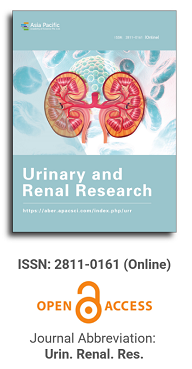
Asia Pacific Academy of Science Pte. Ltd. (APACSCI) specializes in international journal publishing. APACSCI adopts the open access publishing model and provides an important communication bridge for academic groups whose interest fields include engineering, technology, medicine, computer, mathematics, agriculture and forestry, and environment.
Identification of space-occupying lesions in medical imaging of the kidney: A review.
Vol 5, Issue 1, 2024
Download PDF
Abstract
Usually, the kidneys can be affected by renal masses or space-occupying lesions (LOE). When reference is made to the term renal mass, all benign and malignant processes that occupy, distort and affect the renal parenchyma and its environment are included, regardless of etiology, shape and volume. Therefore, renal masses include all cystic formations (abscesses), calculi, pseudotumors, neoplasms, inflammatory diseases and traumatic lesions. Thus, for the evaluation of cystic renal masses in medical imaging, according to their characteristics such as their wall (thin, irregular, thickened), septa (thin, irregular, thickened), borders (defined or not) and size, classifications such as Bosniak's classification shown in Table 1 are used, which classifies renal cysts into five categories based on the appearance of the image, to help predict whether it is a benign or malignant tumor.
Keywords
References
1. Quiroga W, Fernández F, Citarella D, Rangel J, Estrada A, Patiño I. Guidelines for the management of renal cell carcinoma. Revista Urología Colombiana [Internet]. 2016; 35(2):169-189. Retrieved from: https://www.redalyc.org/artic ulo.oa?id=149146287016.
2. Sanz E, García R, Rodríguez R, Arias F, Lennie A, Mayayo T. Ultrasonographic study of renal masses of small size. Arch. Esp. Urol., 59(4), 333-342, 2006.
3. Fernández J, Zuluaga A, Valle Diaz F. Characterization by imaging of renal masses. Atlas by imaging. Actas Urol Esp 33(5): 482-498, 2009.
4. Tonolini M, Lerardi A, Carrafiello G. Letter to the editor : spontaneous renal haemorrhage in end-stage renal disease. Insights Imaging 6: 693-695 (2015). https://doi.org/10.1007/s13244-015-0439-4.
5. Ameri C, Contreras P, Villasante N, Ríos H, Richards N, Mazza O. Solid renal occupying mass up to 4 cm : analysis of 78 cases. Rev Arg de Urol. 7(1): 28-39, 2006.
6. Global Cancer Observatory : International Agency for Research on Cancer [Online]. Available:https://gco.iarc.fr/today/onlineanalysistable?v=2018&mode=cancer&mode_population =continents&population=900&populations=900&key=asr&sex=0&cancer=39&type=0&statistic=5&prev alence=0&population_group=0&ages_group%5B%5D=0&ages_group%5B%5D=17&group_cancer=1&inc lude_nmsc=1&include_nmsc_other=1.
7. Spanish Society of Medical Oncology : Cancer figures in Spain 2020 https://seom.org/seomcms/images/stories/recursos/Cifras_del_cancer_2020.pdf
8. Weekly Epidemiological Bulletin. Epidemiological week 08 (February 17-23, 2019). National Institute of Health. Directorate of surveillance and analysis of Public Health Risk. Online Publication: ISSN 2357-6189. 2019
9. Schmidt L, Linehan W. Genetic predisposition to kidney cancer. Seminars in Oncology, 43(5) : 566-574. 2016.
10. Millet I, Doyon F, Hoa D, ¾uret R, Merigeaud S, Serre I, Taourel P. Characterization of small solid renal lesions: can benign and malignant tumors be differentiated with ct? American journal of roentgenology 197, 887-896. 2011.
11. Huérfano Y, Vera M, Del Mar A, et al., Medical Imaging : Fundamentals and scopes AVFT 35(3): 71-76. 2016.
12. Agnello F, Albano D, Micci G, et al. CT and MR imaging of cystic renal lesions. Insights Imaging 11(5) 2020. https://doi.org/10.1186/s13244-019-0826-3. https://doi.org/10.1186/s13244-019-0826-3
13. Gimpel Ch, Fred E, Breysem L, et al. Imaging of Kidney Cysts and Cystic Kidney Diseases in Children: An International Working Group Consensus Statement. 2019. https://doi.org/10.1148/radiol.2018181243
14. Hélénon O, Crosnier A, Verkarre V, Merran S, Méjean A, Correas J. Simple and complex renal cysts in adults: Classification system for renal cystic masses. Diagnostic and Interventional Imaging 99(1):189-218. 2018.
15. Bartlett S, Solomon C, Tassell B, et al. Complex renal cysts associated with crizotinib treatment Cancer Medicine 4(6):887-896. 2015.
16. Eknoyan G A. Clinical view of simple and complex renal cysts. JASN 20 (9)1874-1876 2009.
17. Israel G, Bosniak M. An update of the Bosniak renal cyst classification system 66(3)484-488. 2005
18. Graumann O, Osther S, Karstoft J, Horlyck A, Sloth P. Evaluation of Bosniak category IIF complex renal cysts Insights Imaging 4(1):471-480. 2013.
19. Ahmad M, Sabr M, Roshy E. Assessment of apparent diffusion coeffi cient value as prognostic factor for renal cell carcinoma aggressiveness Egyptian Journal of Radiology and Nuclear Medicine 50(32). 2019. https://doi.org/ 10.1186∕s43055-019-0038-3.
20. Ishigami K, Jones A, Dahmoush L, Leite L, Pakalniskis M, Barloon T. Imaging spectrum of renal oncocytomas: a pictorial review with pathologic correlation Insights Imaging 6(1):53-64. 2015.
21. Walsh B, Sutijono D, Moore C. Emergency ultrasound diagnosis of traumatic renal cyst rupture Critical Ultrasound Journal 1:127-128. 2010.
22. Peters et al. Incidence of synchronous and metachronous adrenal metastases following tumor nephrectomy in renal cell cancer patients: a retrospective bi-center analysis. Springer Plus 2:293-297. 2013.
23. Rhee H, Blazak J, ¾am CM, Ng KL, Shepherd B, Lawson M, Preston J, Vela I, nomas P, Wood S. Pilot study: use of gallium-68 PSMA PET for detection of metastatic lesions in patients with renal tumour. EJNMMI Res. 2016 Dec;6(1):76. doi: 10.1186∕s13550-016-0231-6. Epub 2016 Oct 22. PMID: 27771904; PMCID: PMC5075321.
24. Mauri G, Nicosia L, Varano G, Bonomo G, Della Vigna P, Monfardini L, Orsi F. Tips and tricks for a safe and effective image-guided percutaneous renal tumour ablation. Insights Imaging 8:357-363 2017.
25. Jin C, Shi F, Xiang D, Jiang X, Zhang B, Wang X, Zhu W, Gao E, Chen X, 3D Fast Automatic Segmentation of Kidney Based on Modified AAM and Random Forest IEEE Transactions on Medical Imaging 35(6), 195-1407. 2016.
26. Shehata M, Mahmoud A, Soliman A, Khalifa F, Ghazal M, Abou El-Ghar M, et al. (2018) 3D kidney segmentation from abdominal diffusion MRI using an appearance-guided deformable boundary. PLoS ONE 13(7). https:// doi.org/10.1371/journal.pone.0200082.
27. Myronenko A, Hatamizadeh A 3D Kidneys and Kidney Tumor Semantic Segmentation using Boundary-Aware Networks arXiv:1909.06684v1 [eess.IV] 14 Sep 2019
28. Kim Y, Ge Y, Tao C, et al. Automated Segmentation of Kidneys from MR Images in Patients with Autosomal Dominant Polycystic Kidney Disease Clin J Am Soc Nephrol 11:576-584, 2016. doi: 10.2215/CJN.08300815.
29. Yang G, Li G, Pan T, Automatic Segmentation of Kidney and Renal Tumor in CT Images Based on Pyramid Pooling and Gradually Enhanced Feature Modules 24th International Conference on Pattern Recognition, ICPR 2018, Aug 2018, Beijing, China. 3790-3795, DOI: 10.1109/ICPR.2018.8545143.
30. Kline T, Korfiatis P, Edwards M, et al. Automatic total kidney volume measurement on follow-up magnetic resonance imaging to facilitate monitoring of autosomal dominant polycystic kidney disease progression Nephrol Dial Transplant (2016) 31:241-248 doi: 10.1093/ndt/gfv314
31. Vu M, Grimbergen G, Simkó A, Nyholm T, Lof⅛tedt T, End-to-End Cascaded U-Nets with a Localization Network for Kidney Tumor Segmentation arXiv:1910.07521v1 [eess.IV] 16 Oct 2019
32. Yin S, Zhang Z, Li H, Peng Q, You X, Furth S, Tasian S, Tasian G, Fan Y Fully-automatic Segmentation of Kidneys in Clinical Ultrasound Images using a Boundary Distance Regression Network Proc IEEE Int Symp Biomed Imaging. 2019: 1741-1744. doi:10.1109/ISBI.2019.8759170.
33. Santini G, Moreau N, Rubeaux M Kidney tumor segmentation using an ensembling multi-stage deep learning approach. A contribution to the KiTS19 challenge. arXiv:1909.00735v1 [eess.IV] 2 Sep 2019.
34. Nithya A, Appathurai A, Venkatadri N, Ramji D, Palagan C Kidney disease detection and segmentation using artificial neural network and multi-kernel k-means clustering for ultrasound images Measurement 149 (2020) 106952 https://doi.org/10.1016.
35. Lituiev D, Cha S, Chin An Automated Localization and Segmentation of Mononuclear Cell Aggregates in Kidney Histological Images Using Deep Learning 2019 medRxiv preprint doi: https://doi.org/10.1101/19002634.
36. Rana S, Jain S, Virmani J. SVM-Based Characterization of Focal Kidney Lesions from B-Mode Ultrasound Images Research Journal of Pharmaceutical, Biological and Chemical Sciences 7(4) 837-846. 2016.
37. Heller N, Isensee F, Maeir-Hein K, et al. ¾e state of the art in kidney and kidney tumor segmentation in contrast-enhanced CT imaging: Results of the KiTS19 Challenge arXiv:1912.01054v2 [eess.IV]. 2019.
38. Heller N, Sathianathen N, Arveen Kalapara et al. ¾e KiTS19 Challenge Data: 300 Kidney Tumor Cases with Clinical Context, CT Semantic Segmentations, and Surgical Outcomes arXiv:1904.00445v1 [q-bio.QM] 31 Mar 2019.
39. Raudales-Díaz I. Diagnostic imaging : Concepts and generalities. Rev Fac Cienc Med. 2014;11(1):35-43. Available from: http://www.bvs.hn/RFCM/pdf/2014/pdf/RFCMVol11-1-2014-6.pdf
Refbacks
- There are currently no refbacks.
Copyright (c) 2024 Author(s)

This work is licensed under a Creative Commons Attribution-NonCommercial 4.0 International License.
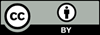
This site is licensed under a Creative Commons Attribution 4.0 International License (CC BY 4.0).
.png)
Prof. Wei-Yen Hsu
National Chung Cheng University, Taiwan